A Compact and Accuracy-Reconfigurable Univariate RBF Kernel Based on Stochastic Logic
ISCAS(2020)
摘要
This paper presents a novel architecture for univariate radial basis kernel computation employing Stochastic Computing. The univariate radial basic function is optimized using simple stochastic logic circuits. We validated this approach by comparison with both Bernstein polynomial and two-dimensional finite-state-machine-based implementation. Optimally, the mean absolute error is reduced 40% and 80% compared to two other well-known approaches, Bernstein polynomial and two-dimensional finite-state-machine-based implementation, respectively. In terms of hardware cost, our proposed solution required as much as the Bernstein method did. Moreover, the proposed approach outperforms the two-dimensional finite-state machine-based mplementation, roughly 54% less hardware cost. Regarding the critical path delay, the proposed approach is 12% less than others on average. Besides, our work also required 70% less power than two-dimensional finite-state-machine-based implementation.
更多查看译文
关键词
Terms Stochastic Computing, radial basis kernel, Bernstein polynomial, two-dimensional finite state machine
AI 理解论文
溯源树
样例
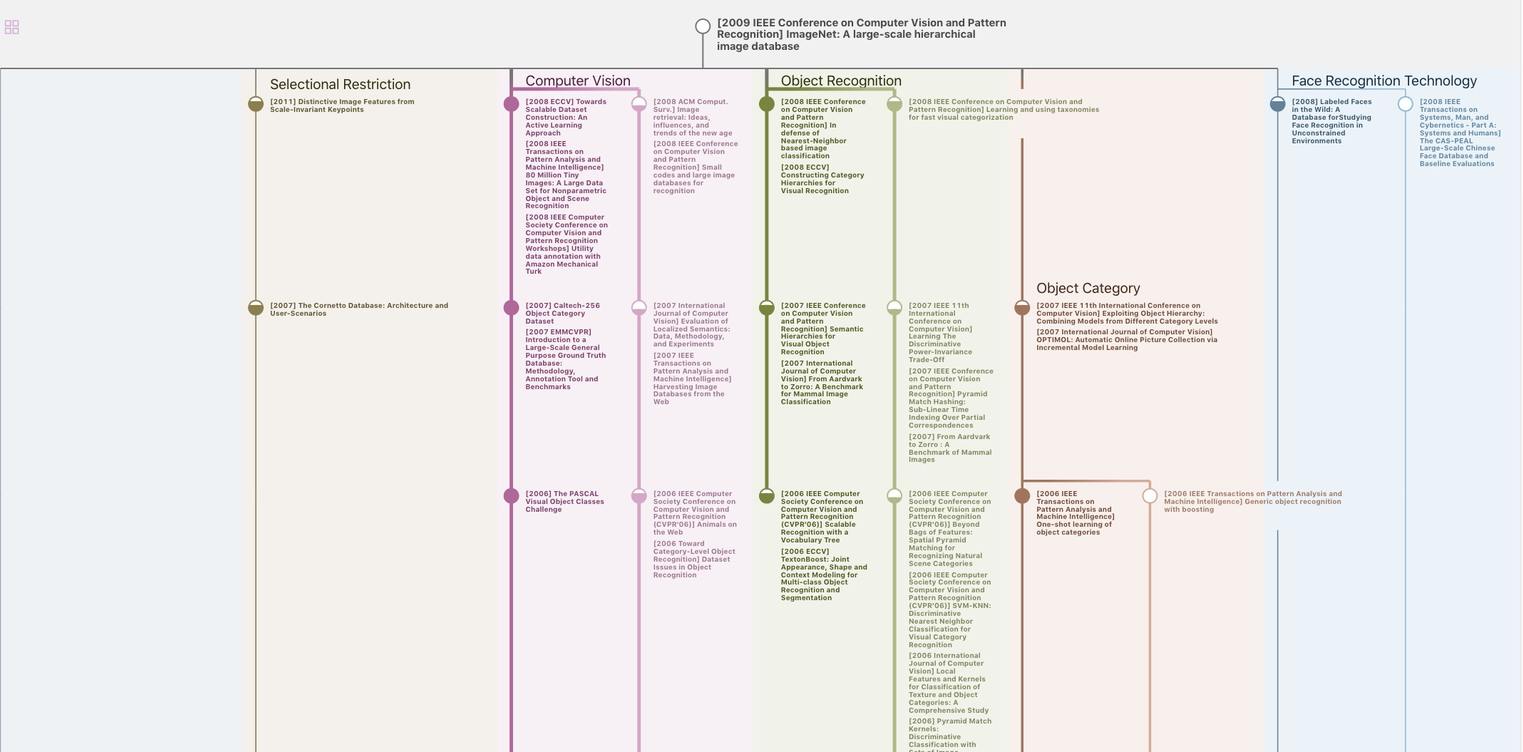
生成溯源树,研究论文发展脉络
Chat Paper
正在生成论文摘要