Habitat mapping using deep neural networks
MULTIMEDIA SYSTEMS(2020)
摘要
Habitat mapping is an important and challenging task that helps in monitoring, managing, and preserving ecosystems. It becomes more challenging when marine habitats are mapped, as it is difficult to get quality images in an underwater environment. Moreover, achieving good location accuracy in underwater environments is an additional issue. Sonar imagery has good quality but is hard to be analyzed. Therefore, camera imagery is used for research purposes. Our research targets marine habitats - more specifically, coral reef marine habitats. Recognition of coral reef in underwater images poses a significant difficulty due to the nature of the data. Many species of coral reef have similar characteristics, i.e. higher inter-class similarity and lower intra-class similarity. Spatial borders between coral reef classes are hard to separate, as they tend to appear together in groups. For these reasons, the classification of coral reef species requires aid from marine biologists. This research work presents a technique for accurate coral reef classification using deep convolutional neural networks. The proposed approach has been validated on Moorea Labeled Corals (MLC), an imbalanced dataset, which is a subset of Moorea Coral Reef Long Term Ecological Research (MCR LTER) packaged for computer vision research. A custom valid patch dataset is extracted using the annotation files provided with the dataset. Two image enhancement algorithms and data-driven feature extraction techniques are employed using several pre-trained deep convolutional neural networks as feature extractors. Local-SPP technique is combined with feature extractors and followed by 2-layers multi-layer perceptron (MLP) classifier to achieve high classification rates.
更多查看译文
关键词
Habitat mapping, Coral reef, Color channel stretching, CLAHE, Convolutional neural networks, Feature extraction
AI 理解论文
溯源树
样例
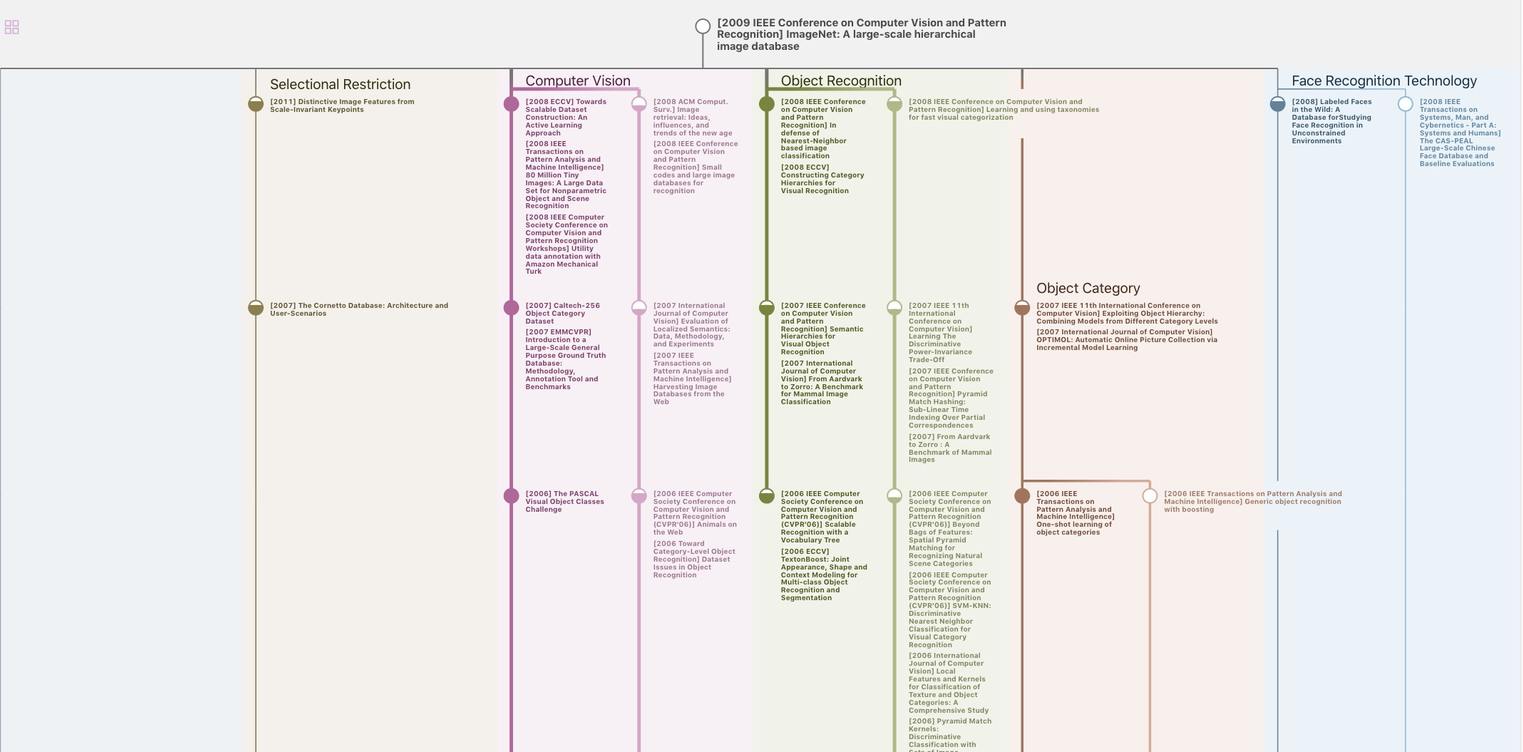
生成溯源树,研究论文发展脉络
Chat Paper
正在生成论文摘要