Predicting Obstructive Hydronephrosis Based on Ultrasound Alone.
medical image computing and computer-assisted intervention(2020)
摘要
Prenatal hydronephrosis (HN) makes up nearly 30% of pediatric Urology Department visits, yet remains challenging to prognosticate without repeated ultrasounds and invasive clinical tests. We build a deep learning model, which uses still images from kidney ultrasound as input and predicts whether HN is due to an obstruction that will receive surgical intervention. We compare our custom convolutional neural network performance against other existing state-of-the-art models. Our best model predicts obstruction with an AUC of 0.93 and an AUPRC of 0.75 in a prospective test set of 89 patients (286 repeated kidney ultrasounds). We show that while maintaining a 5% false negative rate, our classifier identifies 58% of those who will have surgery due to obstruction yet received a functional renogram, indicating that this model could feasibly reduce the amount of testing done in more than half of non-surgical cases. This work demonstrates the ability of deep learning to predict obstructive HN with clinically relevant accuracy based on kidney ultrasound alone, without requiring other clinical variables as input. This algorithm has the potential to change clinical practice by stratifying HN patient risk, reducing repeated follow ups and invasive testing for less severe cases, and bringing more consistency to clinical management.
更多查看译文
关键词
obstructive hydronephrosis,ultrasound
AI 理解论文
溯源树
样例
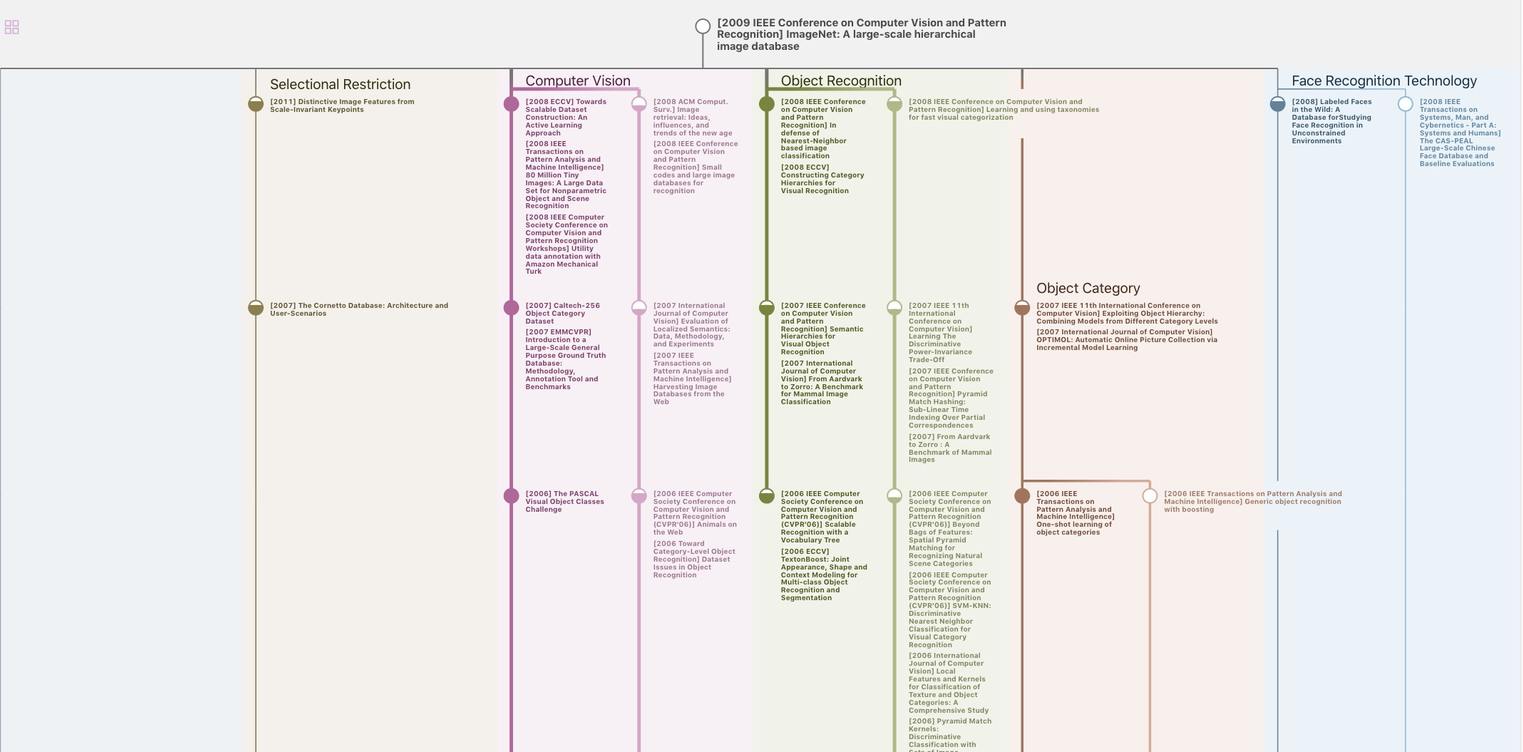
生成溯源树,研究论文发展脉络
Chat Paper
正在生成论文摘要