Segmentation of Paraspinal Muscles at Varied Lumbar Spinal Levels by Explicit Saliency-Aware Learning
medical image computing and computer assisted intervention(2020)
摘要
Automated segmentation for paraspinal muscles on axial lumbar MRIs of varied spinal levels is clinically demanded. However, it is challenging and there is no reported success due to the large inter- and intra-organ variations, unclear muscle boundaries and unpredictable muscle degeneration patterns. In this paper, we propose a novel explicit saliency-aware learning framework (BS-ESNet) for fine segmentation of multiple paraspinal muscles and other major components at varied spinal levels across the full lumbar spine. BS-ESNet is designed to first detect the location of each organ in forms of bounding box (b-box); then performs accurately segmentation which utilizes detected b-boxes to enable spatial saliency awareness. BS-ESNet creatively conducts detection upon a preliminary segmentation mask instead of input MRI, which eliminates the influence of inter-organ variations and is robust against unclear muscle boundaries. Such segment-then-detect workflow also provides a paradigm to formulate multi-organ detection in an end-to-end trainable process. Our framework also embeds an elaborate spatial attention gate which adopts detection b-boxes to obtain a saliency activation map in an explicitly supervised manner. Acquired salient attention map can automatically correct and enhance segmentation features, and further guides the adaptation of variable precise anatomical structures. The method is validated on a challenging dataset of 320 MRIs. Evaluation results demonstrate that our BS-ESNet achieves high segmentation performance with mean Dice score of 0.94 and outperforms other state-of-the-art frameworks.
更多查看译文
关键词
paraspinal muscles,varied lumbar paraspinal levels,saliency-aware
AI 理解论文
溯源树
样例
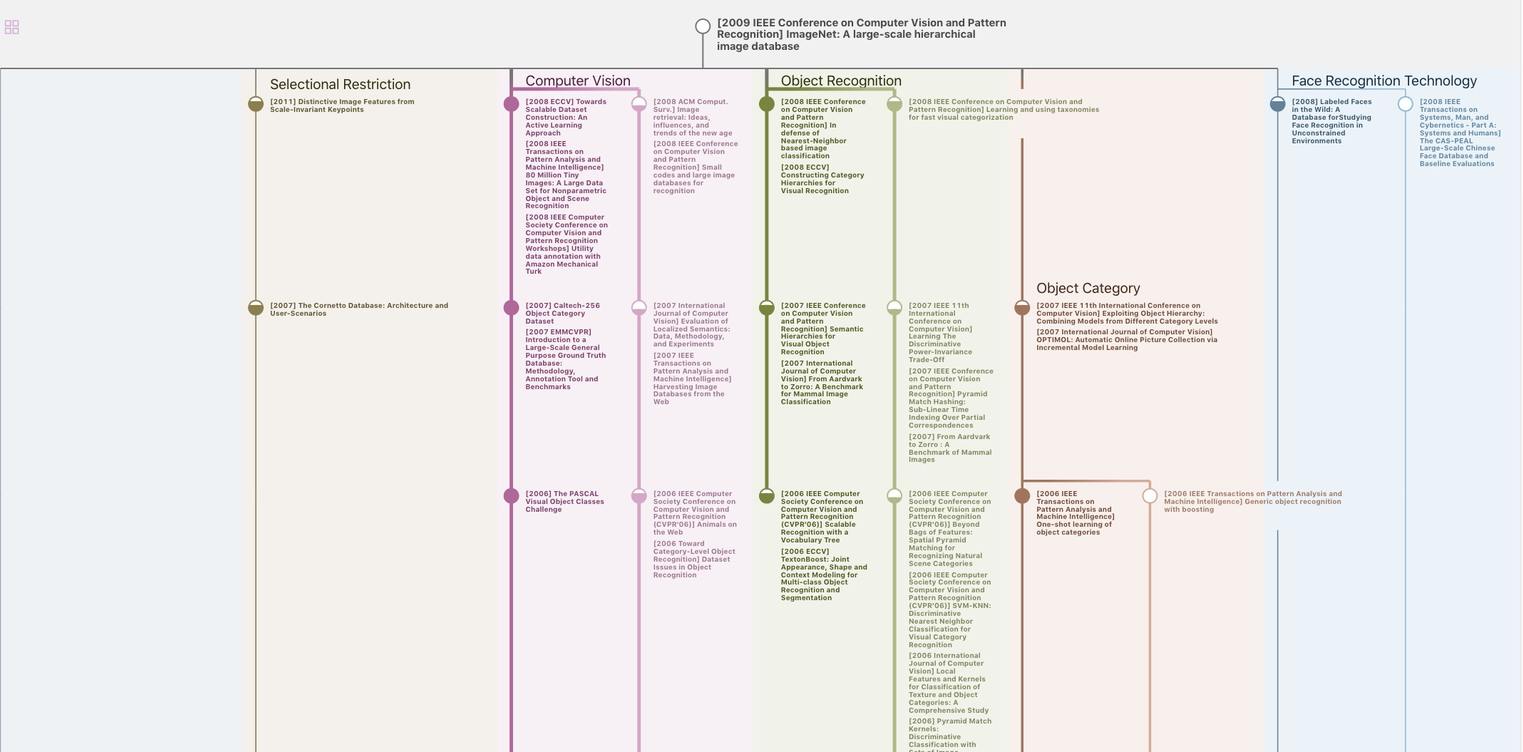
生成溯源树,研究论文发展脉络
Chat Paper
正在生成论文摘要