Multiple Instance Learning with Center Embeddings for Histopathology Classification
medical image computing and computer assisted intervention(2020)
摘要
Histopathology image analysis plays an important role in the treatment and diagnosis of cancer. However, analysis of whole slide images (WSI) with deep learning is challenging given that the duration of pixel-level annotations is laborious and time consuming. To address this, recent methods have considered WSI classification as a Multiple Instance Learning (MIL) problem often with a multi-stage process for learning instance and slide level features. Currently, most methods focus on either instance-selection or instance prediction-aggregation that often fails to generalize and ignores instance relations. In this work, we propose a MIL-based method to jointly learn both instance- and bag-level embeddings in a single framework. In addition, we propose a center loss that maps embeddings of instances from the same bag to a single centroid and reduces intra-class variations. Consequently, our model can accurately predict instance labels and leverages robust hierarchical pooling of features to obtain bag-level features without sacrificing accuracy. Experimental results on curated colon datasets show the effectiveness of the proposed methods against recent state-of-the-art methods.
更多查看译文
关键词
histopathology classification,multiple instance learning,center embeddings
AI 理解论文
溯源树
样例
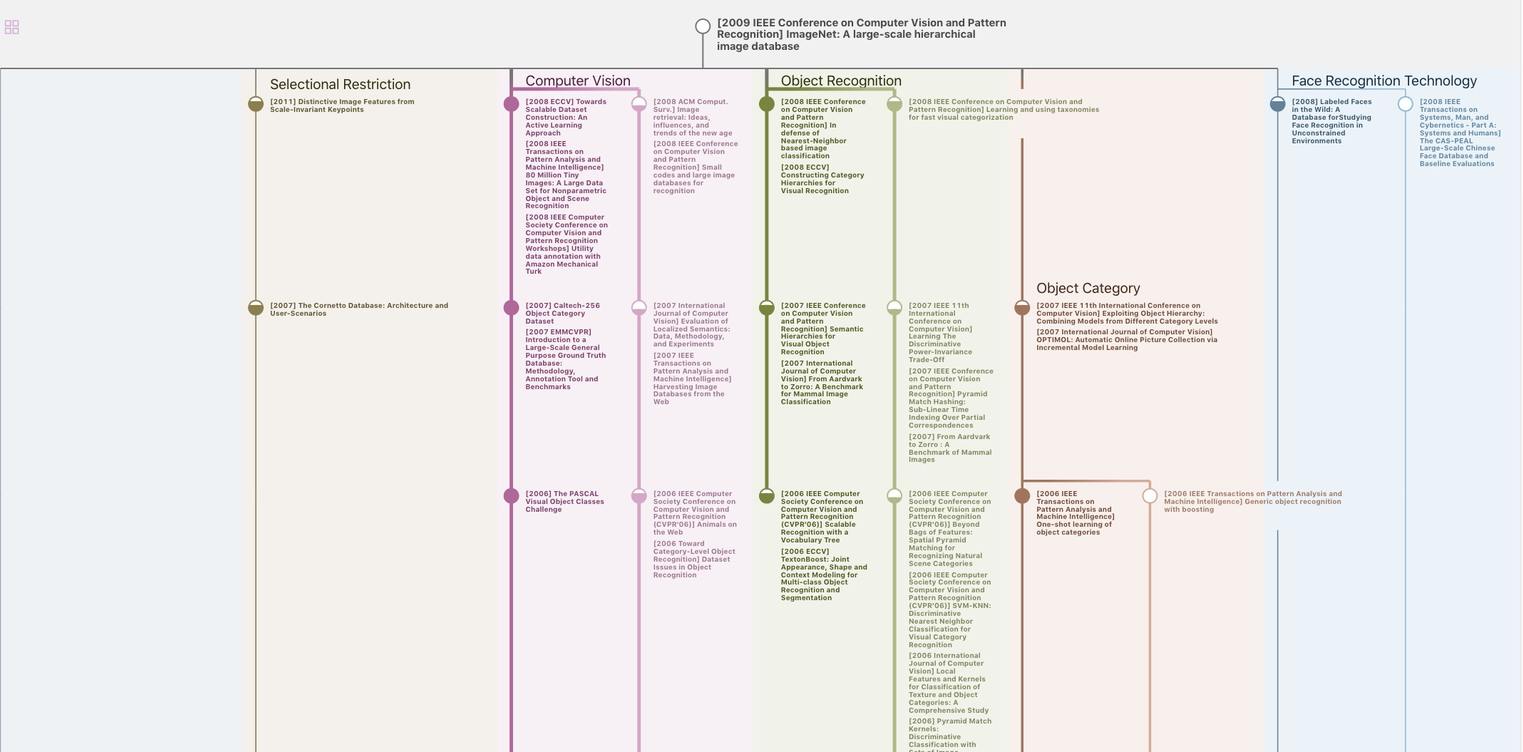
生成溯源树,研究论文发展脉络
Chat Paper
正在生成论文摘要