Modularity-based Representation Learning for Networks
Chinese Physics B/Chinese physics B(2020)
Abstract
Network embedding aims at learning low-dimensional representation of vertexes in a network and effectively preserving network structures. These representations can be used as features for many complex tasks on networks such as community detection and multi-label classification. Some classic methods based on the skip-gram model have been proposed to learn the representation of vertexes. However, these methods do not consider the global structure(i.e., community structure) while sampling vertex sequences in network. To solve this problem, we suggest a novel sampling method which takes community information into consideration. It first samples dense vertex sequences by taking advantage of modularity function and then learns vertex representation by using the skip-gram model. Experimental results on the tasks of community detection and multi-label classification show that our method outperforms three state-of-the-art methods on learning the vertex representations in networks.
MoreTranslated text
Key words
network embedding,low-dimensional representation,vertex sequences,community detection
AI Read Science
Must-Reading Tree
Example
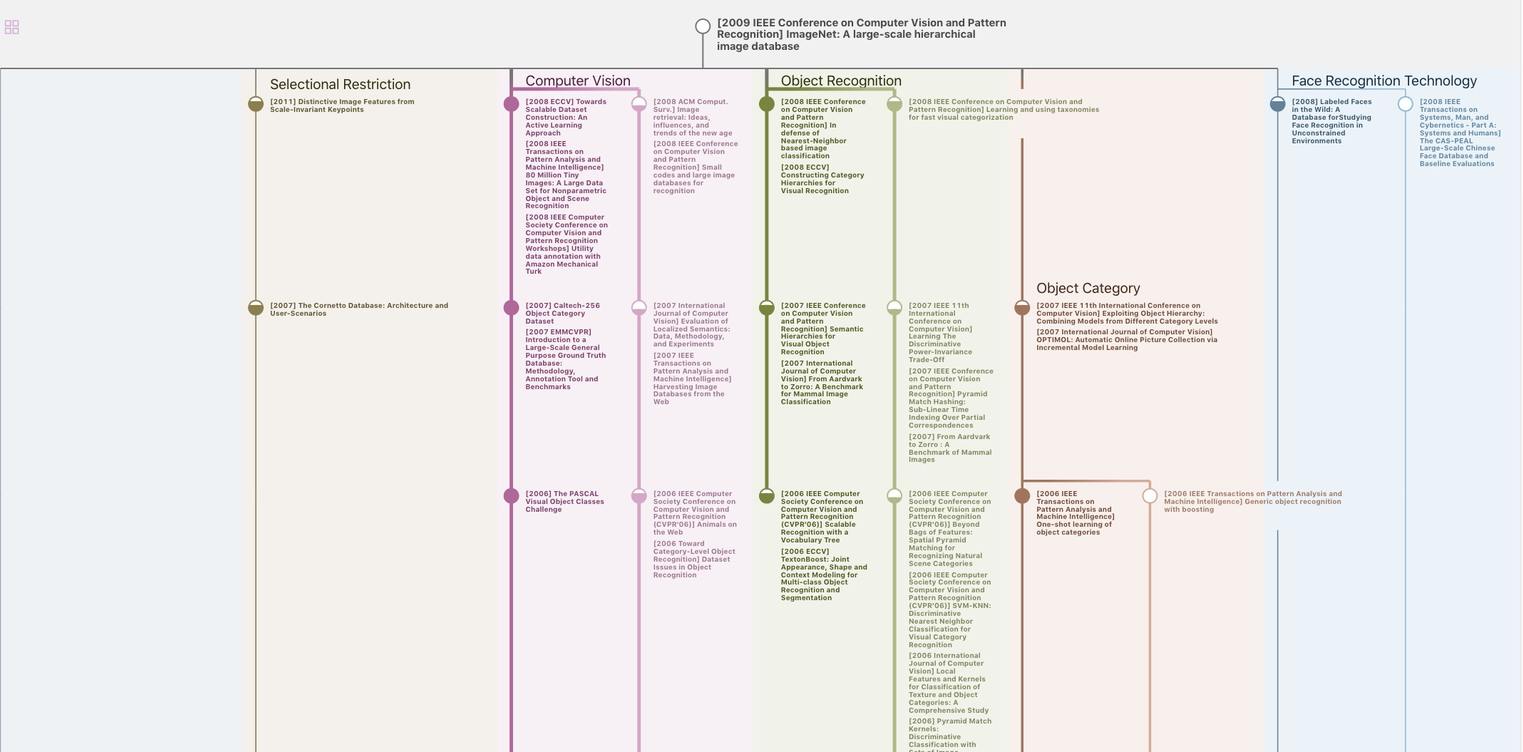
Generate MRT to find the research sequence of this paper
Chat Paper
Summary is being generated by the instructions you defined