A Noise Adaptive Model for Distantly Supervised Relation Extraction
international conference natural language processing(2020)
摘要
Relation extraction is an important task in natural language processing. To obtain a large amount of annotated data, distant supervision is introduced by using large-scale knowledge graphs as external resources. The disadvantage is that distant supervision brings a new issue: noise label, which means the labels obtained by distant supervision may be unreliable and the performance of the models decreases significantly on these datasets. To address the problem, we propose a new framework where noise labels are modeled directly by context-dependent rectification strategy. Intuitively, we adjust the labels that might otherwise be wrong in the right direction. In addition, considering the lack of effective guidance in training with noise, we propose a new curriculum learning-based adaptive mechanism. It learns simple relation extraction task first, then takes the reliability of labels into consideration, so that the model can learn more from the data. The experimental results on a widely used dataset show a significant improvement in our approach and outperform current state-of-the-art.
更多查看译文
关键词
Relation extraction, Denoise, Curriculum learning
AI 理解论文
溯源树
样例
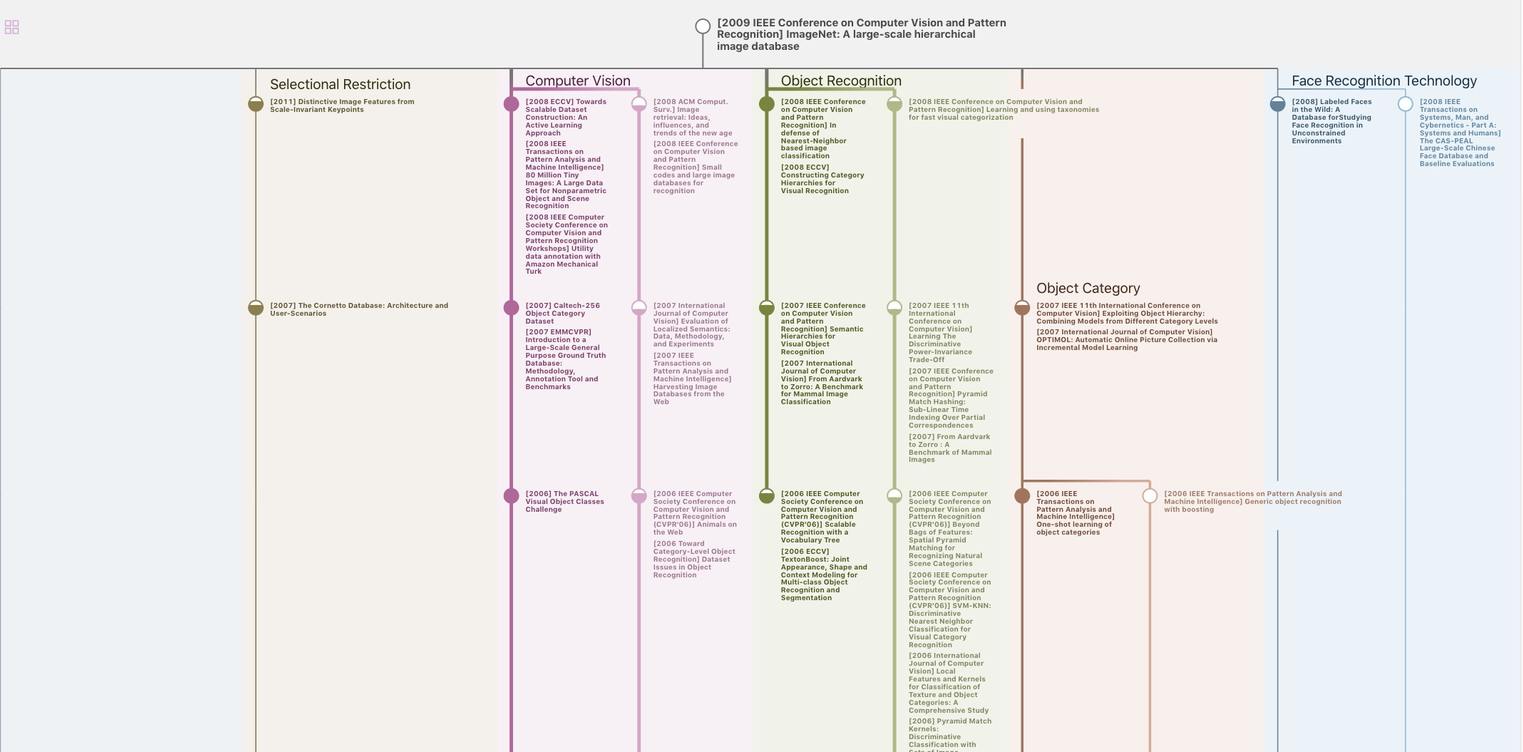
生成溯源树,研究论文发展脉络
Chat Paper
正在生成论文摘要