Graph Convolutional Networks of Reconstructed Graph Structure with Constrained Laplacian Rank.
Multimedia tools and applications(2020)
摘要
Convolutional neural networks (CNNs) have achieved unprecedented competitiveness in text and two-dimensional image data processing because of its good accuracy performance and high detection speed. Graph convolutional networks (GCNs), as an extension of classical CNNs in graph data processing, have attracted wide attention. At present, GCNs often use domain knowledge (such as citation recommendation system, biological cell networks) or artificial created fixed graph to achieve various semi-supervised classication tasks. Poor quality graph will lead to suboptimal results of semi-supervised classification tasks. We propose a more general GCN of reconstructed graph structure with constrained Laplacian rank. First, we use hypergraph to establish multivariate relationships between data. On the basis of the hypergraph, In virtue of Laplacian rank constraint to the graph matrix, we learn a new graph structure which has c connected components (where c is the number of classification), and then we construct an ideal graph matrix which is more suitable for the task of semi-supervised classification on GCNs. Finally, the data and the new graph are input GCNs model to get the results of classification. Experiments on 10 different datasets demonstrate that this method is more competitive than the comparison method.
更多查看译文
关键词
Graph convolutional networks,Adaptive graph,Hypergraph,Semi-supervised classification,Graph structure
AI 理解论文
溯源树
样例
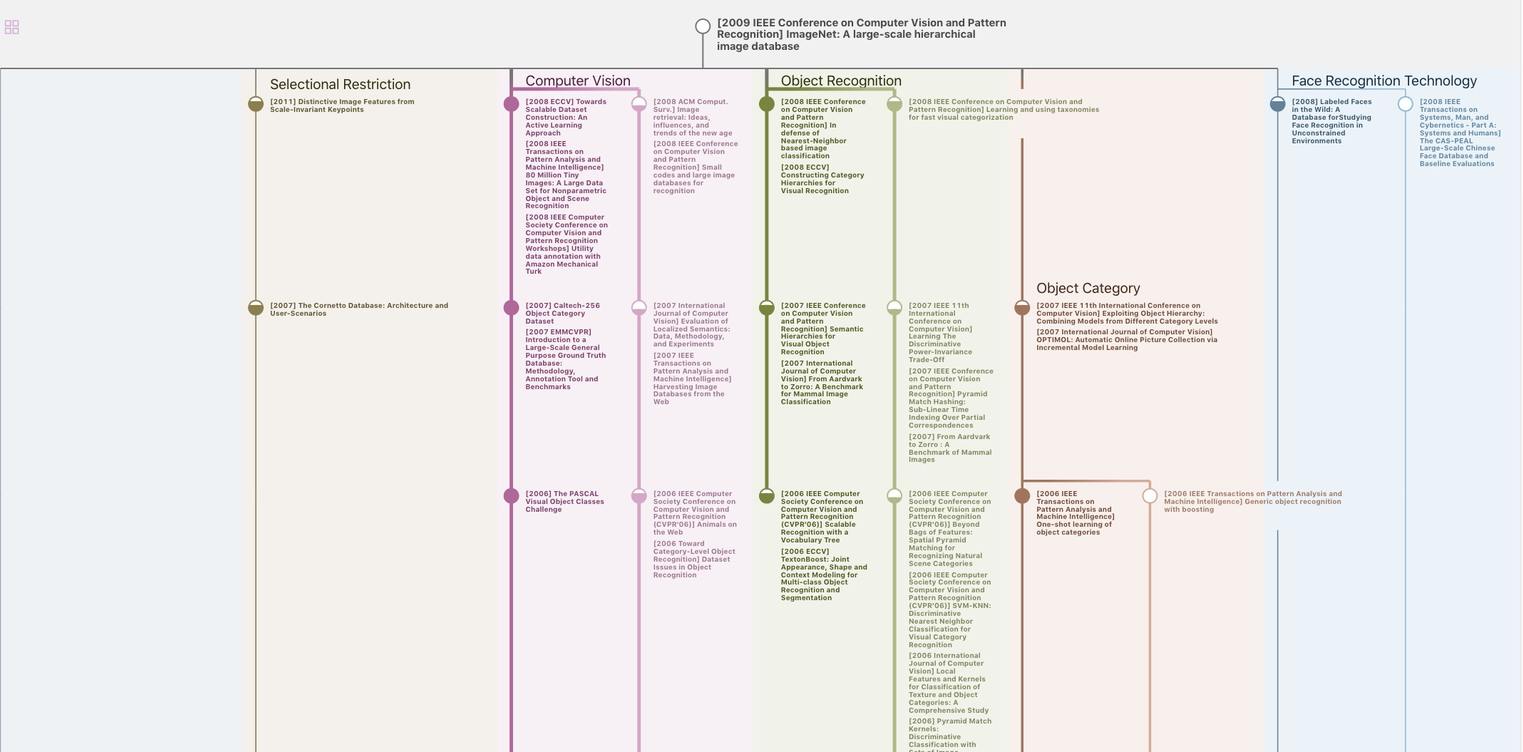
生成溯源树,研究论文发展脉络
Chat Paper
正在生成论文摘要