Performance Analysis of NOMA Enabled Fog Radio Access Networks
IEEE Transactions on Communications(2021)
摘要
In the fifth generation (5G) era, mobile networks will provide diversified services such as enhanced Mobile BroadBand (eMBB) and ultra-reliable low-latency communication (URLLC). Furthermore, the 5G architecture will be fog-like, enabling a functional split of network functionalities between cloud and edge nodes. In this article, we propose a non-orthogonal multiple access (NOMA)-enabled fog-cloud structure in a novel density-aware fog-based radio access networks (F-RAN) to tackle different aspects of high and low-density regions, in order to meet the heterogeneous requirements of eMBB and URLLC traffic. A framework of two different problem formulations is considered to cater the high throughput and low-latency requirements in a high and low-density mode respectively. In the first problem, we study the joint caching placement and association strategy aiming at minimizing the average delay. To deal with the first problem, we apply McCormick envelopes and Lagrange partial relaxation method to transform it into three convex sub-problems, which is then solved by proposing a distributed algorithm. The second problem is to jointly optimize transmission mode selection, subchannel assignment and power allocation to maximize the sum data rate of all fog user equipments (F-UEs) while satisfying fronthaul capacity and fog-computing access point (F-AP) power constraints. Moreover, for given transmission mode selection and subchannel assignment, the optimal power allocation is derived in a closed-form expression. Simulation results are provided to validate the effectiveness of the proposed NOMA-enabled F-RAN framework and reveal that the ultra-low latency and high throughput can be achieved by properly utilizing the available resources.
更多查看译文
关键词
Fog radio access networks (F-RANs),non-orthogonal multiple access (NOMA),ultra-reliable low-latency communication (URLLC),enhanced mobile BroadBand (eMBB),mode selection,content popularity
AI 理解论文
溯源树
样例
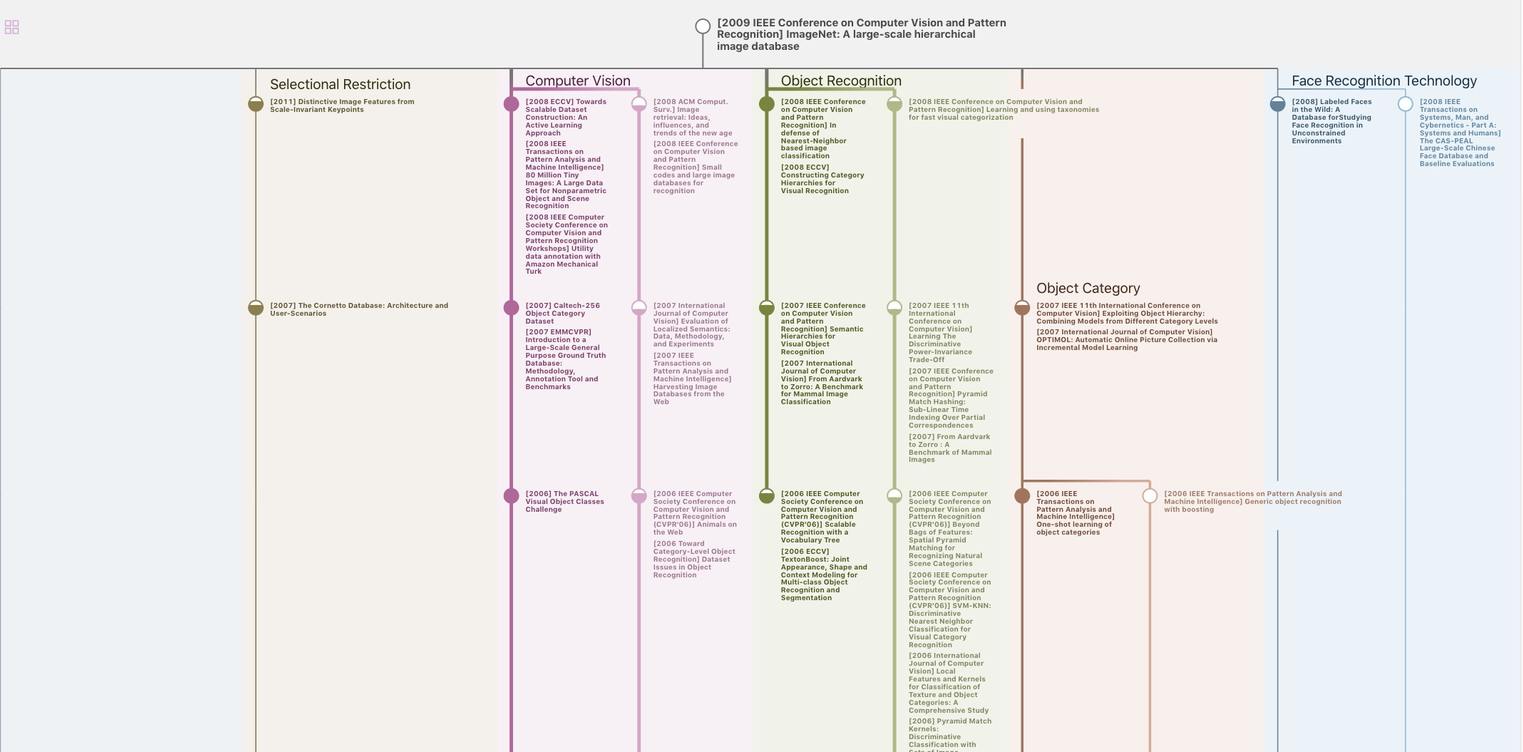
生成溯源树,研究论文发展脉络
Chat Paper
正在生成论文摘要