Content Caching Oriented Popularity Prediction: A Weighted Clustering Approach
IEEE Transactions on Wireless Communications(2021)
摘要
Content popularity prediction plays an important role on proactive content caching. Different to most of the existing works which focus on improving the popularity prediction accuracy, in this article, we consider the content caching oriented popularity prediction through a weighted clustering approach in order to improve the caching performance. We formulate the loss of the cache hit ratio as the system regret to indicate the caching performance, and construct a clustering-based popularity prediction framework for overcoming the user request sparsity with considering the similarity of popularity evolution trends. For depicting the explicit relationship between the caching performance and the popularity prediction accuracy, we derive the popularity prediction error distribution of each content, and design the caching threshold. By extracting the insights in the relationship between the popularity prediction accuracy and the user clustering strategy, we develop a weighted clustering-based popularity prediction algorithm, which takes the caching regret probability of files as the weights. Based on two real-world datasets, the simulation results demonstrate that the proposed popularity prediction scheme achieves better caching performance than the state-of-the-art schemes.
更多查看译文
关键词
Content caching,popularity prediction,clustering,regret minimization,feedforward neural network (FNN)
AI 理解论文
溯源树
样例
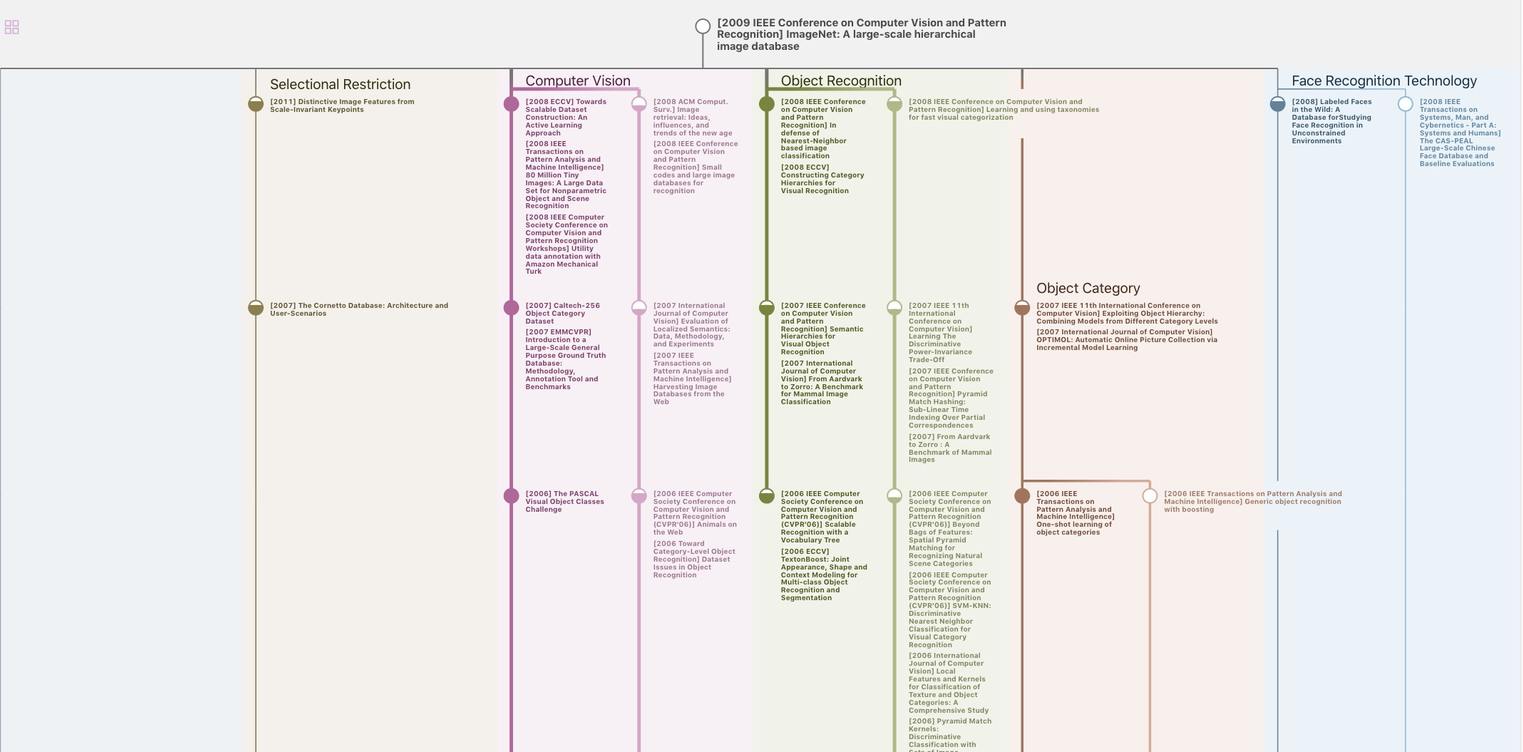
生成溯源树,研究论文发展脉络
Chat Paper
正在生成论文摘要