Online Estimation Of Voltage Stability Margin Via Deep Neural Network With Consideration Of The Local Structures In Power Grid
INTERNATIONAL TRANSACTIONS ON ELECTRICAL ENERGY SYSTEMS(2020)
摘要
Objective Online estimation of voltage stability margin (VSM) is critical to the long-term safety and stable operation of power system. In this paper, a deep neural network (DNN)-based model that incorporates the intrinsic grid topology information is proposed to achieve accurate VSM estimation. Methods The node embedding information together with the electrical parameter measurements are combined together into an array of local structures as the input feature vector. A local-global deep neural network (LGDNN) model is proposed to extract and integrate the local information into high-level global representation for VSM estimation. The Node2vec algorithm is employed to obtain embedded node vectors and a NodeRank algorithm is designed to form local structures, which are composed of the measurements of a fixed number of similar nodes according to the similarity among their embedded vectors. A sequential DNN with cascade-connected depthwise separable 1D convolutional layer and fully connected layer is trained to estimate the current VSM. Results The model performance is validated on the IEEE-39 and IEEE-118 benchmark systems under normal and post-contingency situations. Experiment results indicate that the proposed model can achieve VSM estimation with high precision that surpasses seven mainstream approaches in multiple estimation error indices. Conclusion Through the integration of local structures, the proposed LGDNN increases the estimation accuracy with relatively few parameters than various DNN-based models. Thus, it is an practical model for online power grid voltage stability monitoring.
更多查看译文
关键词
deep neural network, grid topology, local structures, voltage stability margin
AI 理解论文
溯源树
样例
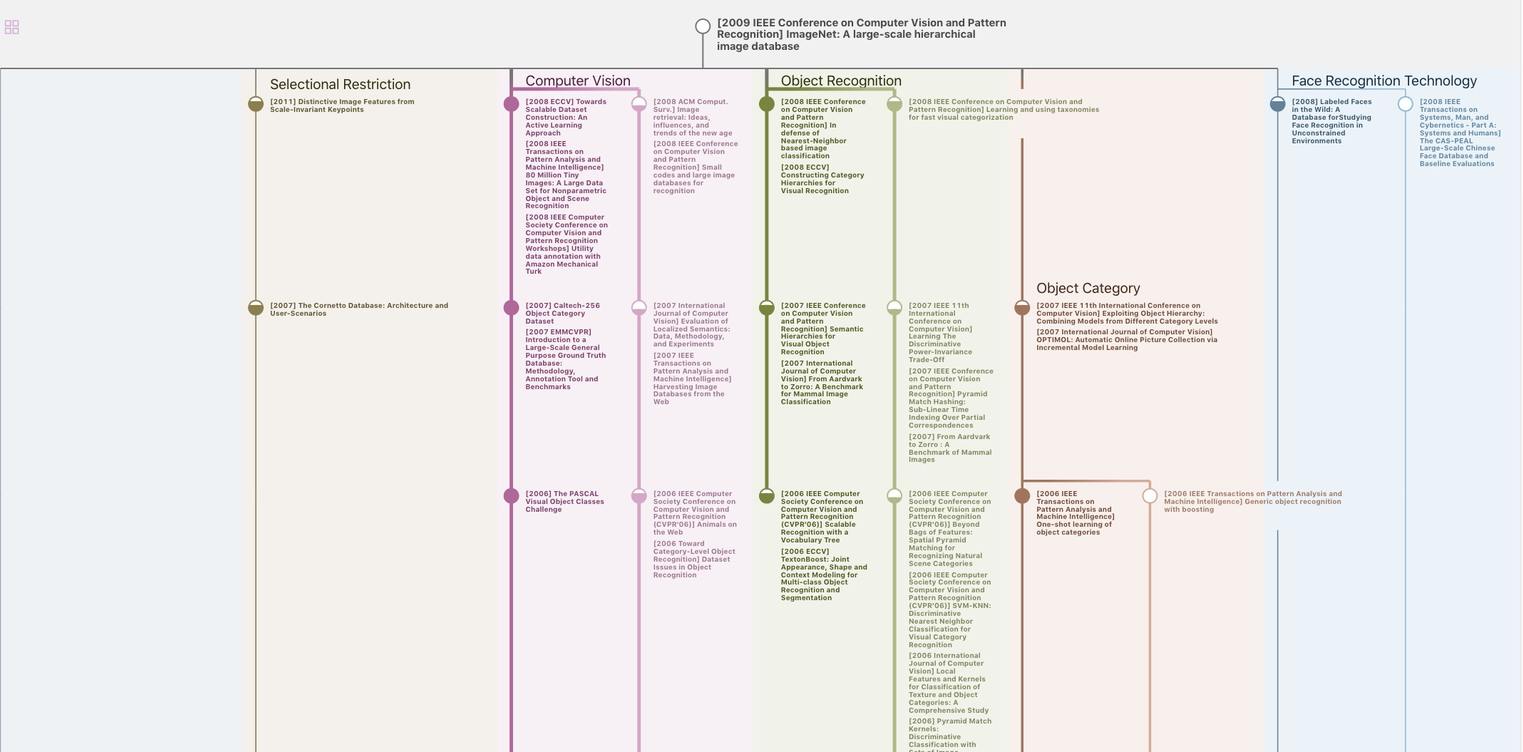
生成溯源树,研究论文发展脉络
Chat Paper
正在生成论文摘要