Change Detection Based on Low-Level to High-Level Features Integration With Limited Samples
IEEE JOURNAL OF SELECTED TOPICS IN APPLIED EARTH OBSERVATIONS AND REMOTE SENSING(2020)
摘要
Detailed land cover change in multitemporal images is an important application for earth science. Many techniques have been proposed to solve this problem in different ways. However, accurately identifying changes still remains a challenge due to the difficulties in describing the characteristics of various change categories by using single-level features. In this article, a multilevel feature representation framework was designed to build robust feature set for complex change detection task. First, four different levels of information from low level to high level, including pixel-level, neighborhood-level, object-level, and scene-level features, were extracted. Through the operation of extracting different level features from multitemporal images, the differences between them can be described comprehensively. Second, multilevel features were fused to reduce the dimension and then used as the input for supervised change detector with initial limited labels. Finally, for reducing the labeling cost and improving the change detection results simultaneously, active learning was conducted to select the most informative samples for labeling, and this step together with the previous steps were iteratively conducted to improve the results in each round. Experimental results of three pairs of real remote sensing datasets demonstrated that the proposed framework outperformed the other state-of-the-art methods in terms of accuracy. Moreover, the influences of scene scale for high-level semantic features in the proposed approach on change detection performance were also analyzed and discussed.
更多查看译文
关键词
Feature extraction,Remote sensing,Image segmentation,Task analysis,Labeling,Semantics,Detectors,Active learning,attribute profiles (APs),change detection,convolutional neural network (CNN),multilevel feature,object feature,scene feature
AI 理解论文
溯源树
样例
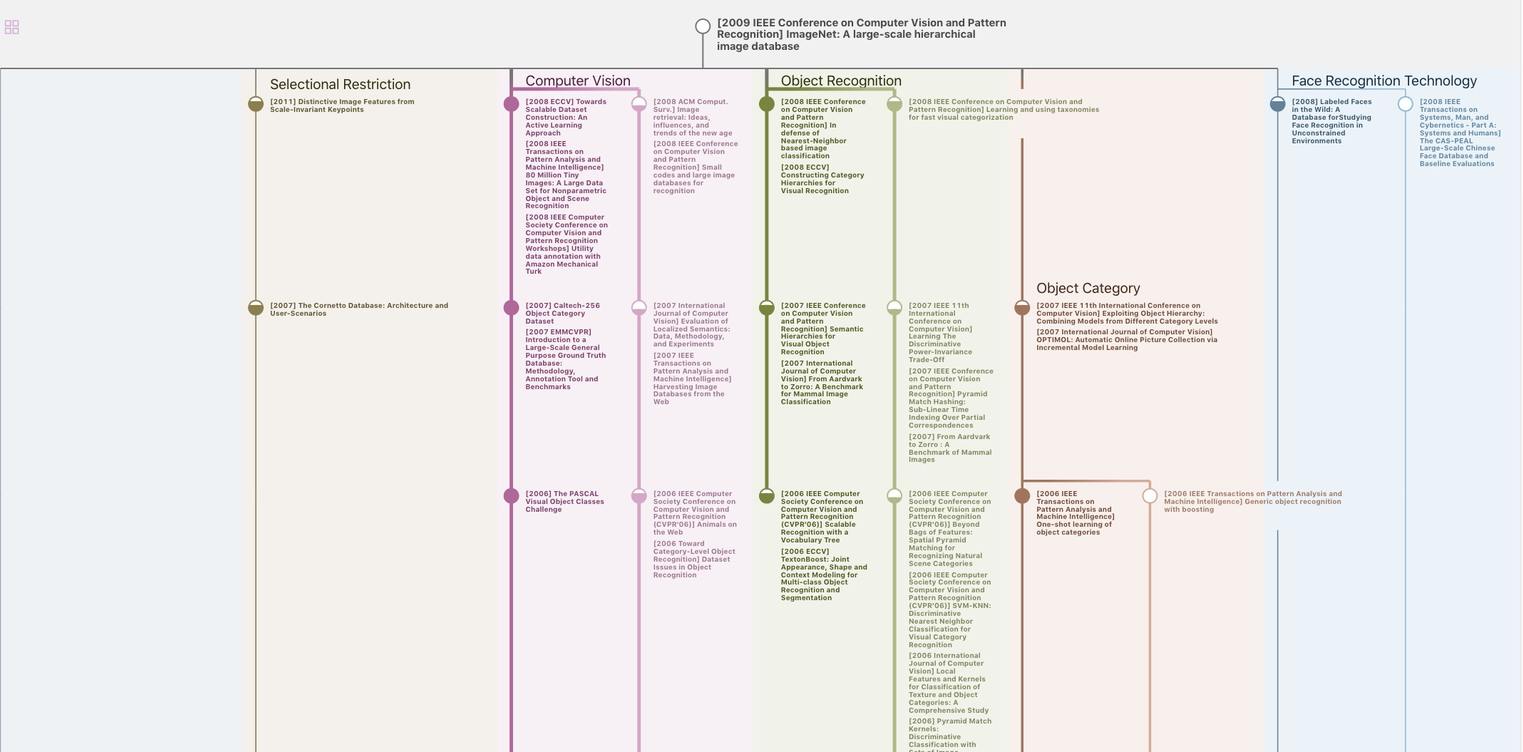
生成溯源树,研究论文发展脉络
Chat Paper
正在生成论文摘要