Deep Learning-Enabled Sparse Industrial Crowdsensing and Prediction.
IEEE transactions on industrial informatics(2021)
摘要
Mobile Crowdsensing (MCS) is a powerful sensing paradigm, which provides sufficient social data for cognitive analytics in industrial sensing, and industrial manufacturing. Considering the sensing costs, sparse MCS, as a variant, only senses the data in a few subareas, and then infers the data of unsensed subareas by the spatio-temporal relationship of the sensed data. Existing works usually assume that the sensed data are linearly spatiotemporal dependent, which cannot work well in real-world nonlinear systems, and thus, result in low data inference accuracy. Moreover, in many cases, users not only require inferring the current data, but also have an interest in predicting the near future, which can provide more information for users' decision making. Facing these problems, we propose a deep learning-enabled industrial sensing, and prediction scheme based on sparse MCS, which consists of two parts: matrix completion and future prediction. Our goal is to achieve high-precision prediction of future moments under the hypothesis of sparse historical data. To make full use of the sparse data for prediction, we first propose a deep matrix factorization method, which can retain the nonlinear temporal-spatial relationship, and perform high-precision matrix completion. In order to predict the subareas' data in several future sensing cycles, we further propose a nonlinear autoregressive neural network, and a stacked denoising autoencoder to obtain the temporal-spatial correlation between the data from different cycles or subareas. According to the results gained by experiments on four real-world industrial sensing datasets consisting of six typical tasks, it can be seen that the method in this article improves the accuracy of prediction using sparse data.
更多查看译文
关键词
Sensors,Sparse matrices,Correlation,Manufacturing,Task analysis,Inference algorithms,Data models,Data inference,deep matrix completion,mobile crowdsensing (MCS),spatiotemporal series prediction
AI 理解论文
溯源树
样例
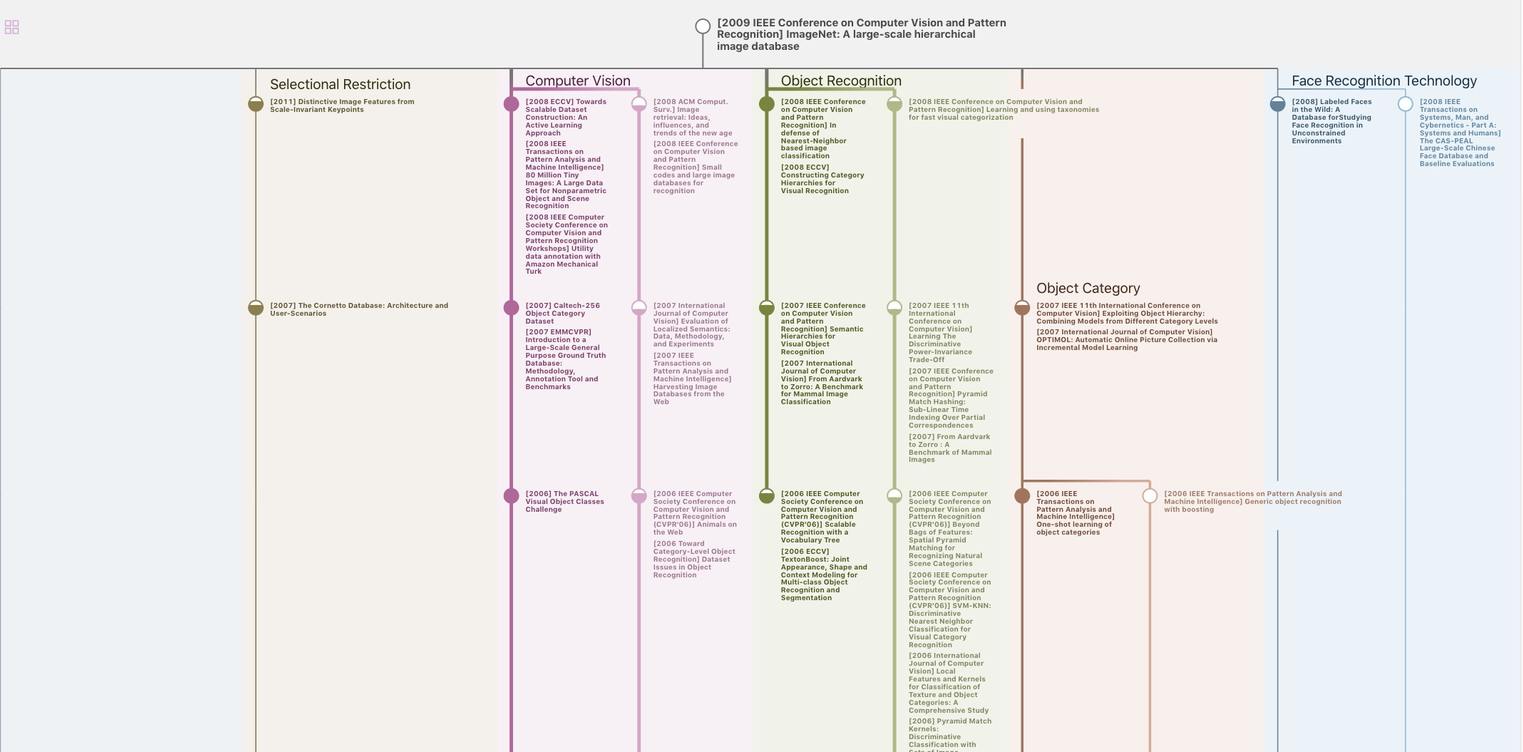
生成溯源树,研究论文发展脉络
Chat Paper
正在生成论文摘要