Physics-guided deep learning for predicting geological drilling risk of wellbore instability using seismic attributes data
Engineering Geology(2020)
摘要
Wellbore instability is a major safety and environmental concern in both onshore and offshore drilling. Geological drilling risk assessment for wellbore collapse is critical for the optimization of well plans, and to reduce potential costs before drilling. In this work, we propose a physics-guided deep learning approach to predict wellbore instability using seismic attributes data. We first trained an auto-encoder to extract latent representation (five principle features) from 17 typical seismic attributes, and then we introduced a regularization term, based on geomechanics in the objective function to train a neural network. As long as there is no significant over-pressure in the formation, the physics-based regularization term indicating wellbore instability risk is a function of neutron porosity, and of the true vertical depth obtained from well logging data. In this way, we combined drill log data for five wells, as prior information, with latent seismic attribute representations, to train the neural network. After training, our approach needed only seismic data to predict wellbore instability risk in new locations, and our case study showed that the physics-based regularizer, with an appropriate weight, prevented overfitting to training data and enhanced the generalization accuracy of the neural network (by ~4%) in two new test wells. We argue that statistical correlations between seismic attributes and rock properties are algorithm dependent, and have to be treated cautiously in the absence of a base of petrophysical reasoning. The physics-guided deep learning method presented here has potential application for the quantification of geology-based wellbore instability risk before drilling.
更多查看译文
关键词
Physics-guided deep learning,Wellbore instability,Seismic attribute,Drilling risk
AI 理解论文
溯源树
样例
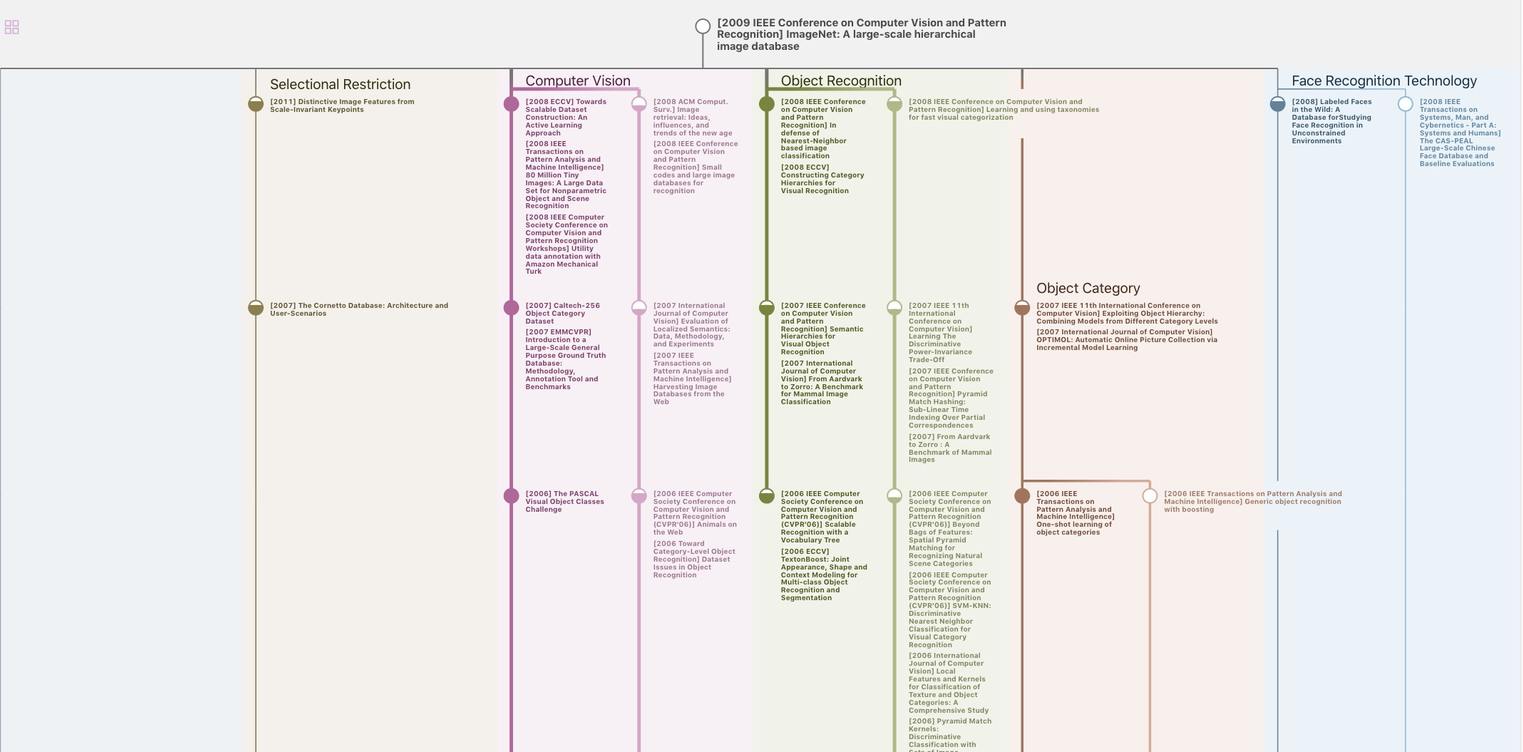
生成溯源树,研究论文发展脉络
Chat Paper
正在生成论文摘要