A New Model to Distinguish Railhead Defects Based on Set-Membership Type-2 Fuzzy Logic System
INTERNATIONAL JOURNAL OF FUZZY SYSTEMS(2020)
摘要
This paper focuses on the new model for the classification of railhead defects, through images acquired by a rail inspection vehicle. In this regard, we discuss the use of set-membership concept, derived from the adaptive filter theory, into the training procedure of an upper and lower singleton type-2 fuzzy logic system, aiming to reduce computational complexity and to increase the convergence speed. The performance is based on the data set composed of images provided by a Brazilian railway company, which covers the four possible railhead defects (cracking, flaking, head-check and spalling) and the normal condition of the railhead. Additionally, we apply different levels of additive white Gaussian noise in the images in order to challenge the proposed model. Finally, we discuss performance analysis in terms of convergence speed, computational complexity reduction, and classification ratio. The reported results show that the proposal achieved improved convergence speed, slightly higher classification ratio and remarkable computation complexity reduction when we limit the number of epochs for training, which may be required under real-time constraint or low computational resource availability.
更多查看译文
关键词
Type-2 fuzzy logic systems, Set-membership, Computational complexity reduction, Adaptive algorithms
AI 理解论文
溯源树
样例
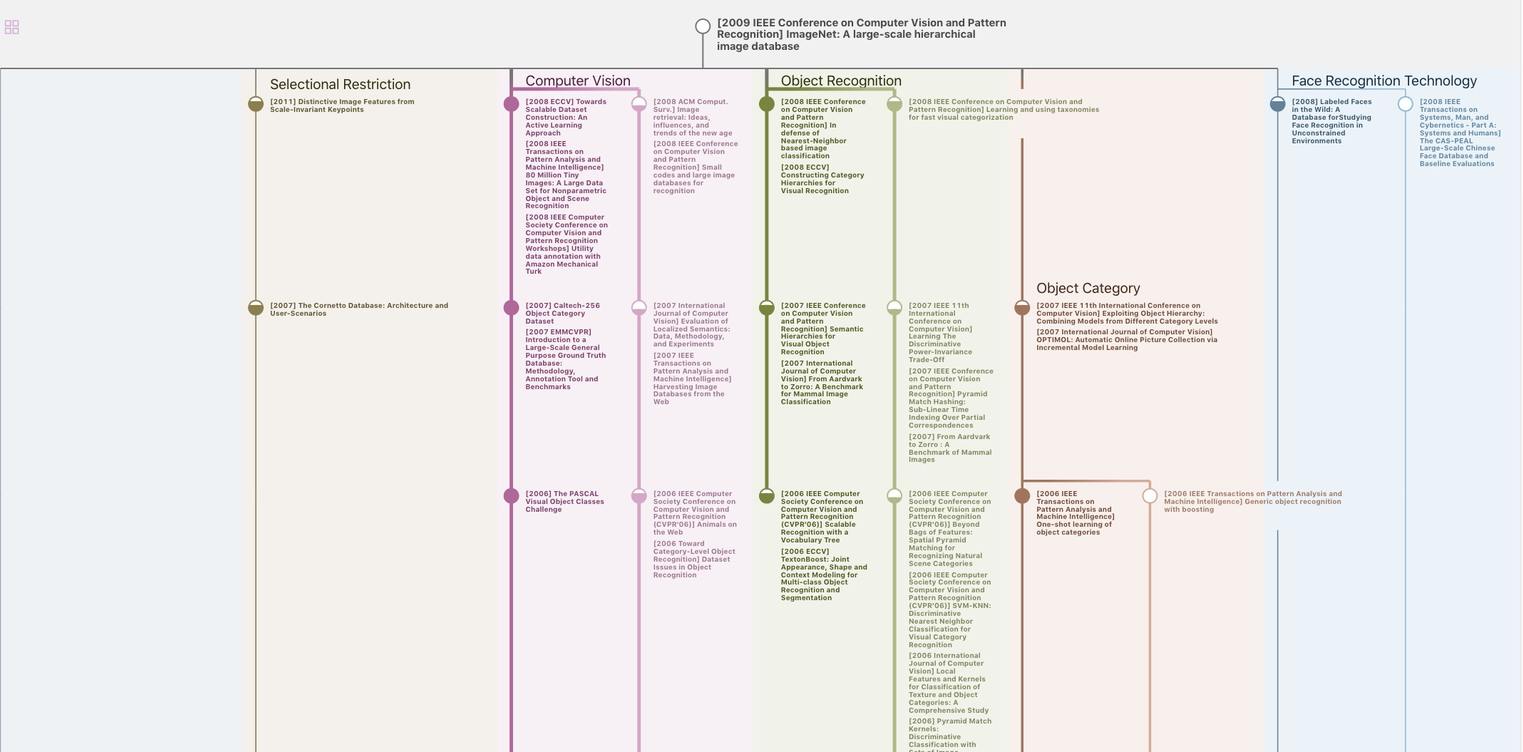
生成溯源树,研究论文发展脉络
Chat Paper
正在生成论文摘要