Potential-Driven Model For Influence Maximization In Social Networks
IEEE ACCESS(2020)
摘要
The adoption of a new technology, the spreading of ideologies, the transmission of contagions, are examples of spreading phenomena that can be modelled at two distinct levels of resolution, a macroscopic and a microscopic level.In the present work, we consider the network at the microscopic level and represent its nodes as a system of interacting particles and propose a novel, physics-inspired approach. This approach assumes that nodes interact via forces that derive from the potential that each node creates at the location of the other nodes, leading to a potential gradient that indicates the natural direction of diffusion through the network. A set of influencers in the network, is determined from strategically selected nodes based on the value of their net potential. We use synthetic networks of various sizes and compare the influence spread resulting from a seed set determined from the potential-based model and alternate approaches, including the greedy algorithm of Kempe et al. Our findings indicate that this approach achieves comparable results to those of the greedy algorithm without the prohibitive computational cost, and consistently outperforms the other approaches by a large margin. We then apply our methodology to information spreading related to the Twitter Higgs Boson user dataset. The results are analyzed in the context of influence maximization, and we provide insights into the general application of this technique for information dissemination.
更多查看译文
关键词
Technological innovation, Microscopy, Greedy algorithms, Entropy, Computational modeling, Electric potential, Social network services, Influence maximization, information diffusion, viral marketing, linear threshold model, early adopters, influencers
AI 理解论文
溯源树
样例
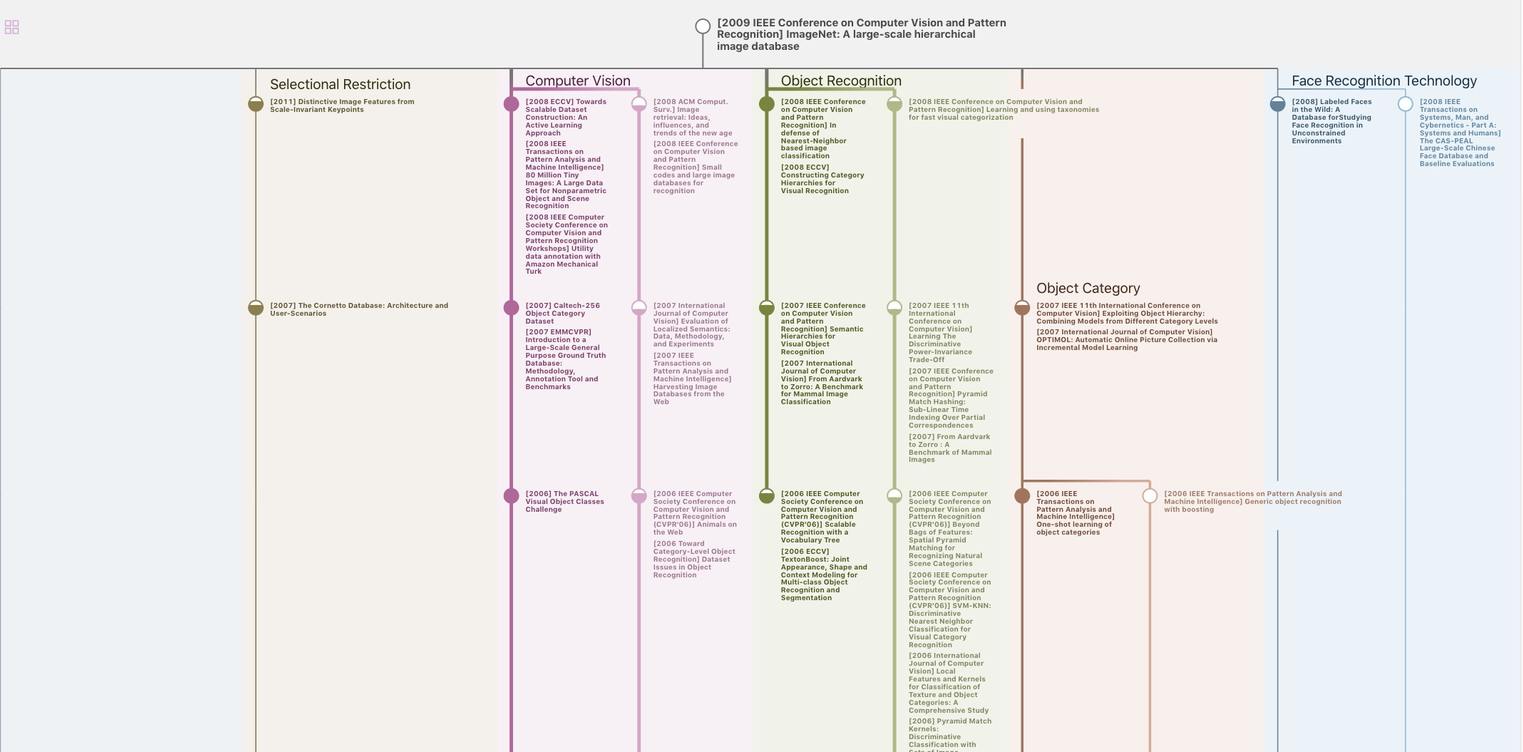
生成溯源树,研究论文发展脉络
Chat Paper
正在生成论文摘要