Application of Near-Infrared Hyperspectral Imaging for Variety Identification of Coated Maize Kernels with Deep Learning
INFRARED PHYSICS & TECHNOLOGY(2020)
摘要
Tracing the varieties of seeds is of great importance for the seed industry. Maize kernels for planting are generally coated to protect kernels from fungi and insects. In this study, near-infrared hyperspectral imaging ranging from 874 nm to 1734 nm was used to identify the varieties of coated maize kernels. Spectral data were extracted and preprocessed. Logistic regression (LR) and support vector machine (SVM), convolutional neural network (CNN), recurrent neural network (RNN) and Long Short-Term Memory (LSTM) were used to build classification models. Furthermore, principal component analysis (PCA), CNN, RNN and LSTM were adopted to extract features. The extracted features were fused as the inputs of the classification models. Classification models using full spectra, extracted features and fused features obtained performances with the classification accuracy over 90% in the calibration, validation and prediction sets of most models. Models using extracted features obtained equivalently or slightly worse results than those using full spectra. The models using fused features all obtained good performances, with the classification accuracy over 90% in all sets. The overall results illustrated that near-infrared hyperspectral imaging with deep learning methods was a useful alternative for identifying coated maize varieties.
更多查看译文
关键词
Deep learning,Coated maize kernels,Feature extraction,Feature fusion,Near-infrared hyperspectral imaging
AI 理解论文
溯源树
样例
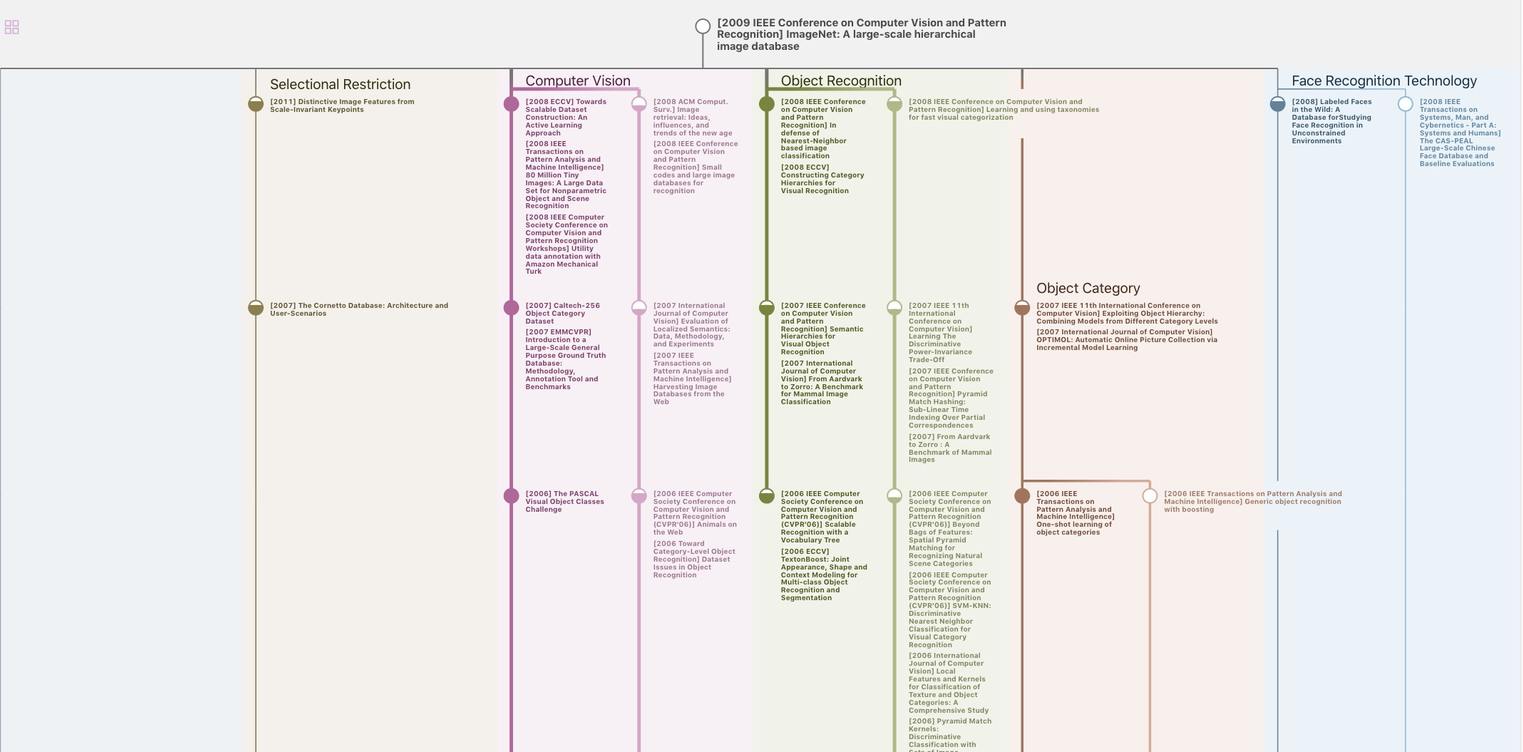
生成溯源树,研究论文发展脉络
Chat Paper
正在生成论文摘要