Improving Deep Learning-Based UWB LOS/NLOS Identification with Transfer Learning: An Empirical Approach
ELECTRONICS(2020)
摘要
This paper presents an improved ultra-wideband (UWB) line of sight (LOS)/non-line of sight (NLOS) identification scheme based on a hybrid method of deep learning and transfer learning. Previous studies have limitations, in that the classification accuracy significantly decreases in an unknown place. To solve this problem, we propose a transfer learning-based NLOS identification method for classifying the NLOS conditions of the UWB signal in an unmeasured environment. Both the multilayer perceptron and convolutional neural network (CNN) are introduced as classifiers for NLOS conditions. We evaluate the proposed scheme by conducting experiments in both measured and unmeasured environments. Channel data were measured using a Decawave EVK1000 in two similar indoor office environments. In the unmeasured environment, the existing CNN method showed an accuracy of approximately 44%, but when the proposed scheme was applied to the CNN, it showed an accuracy of up to 98%. The training time of the proposed scheme was measured to be approximately 48 times faster than that of the existing CNN. When comparing the proposed scheme with learning a new CNN in an unmeasured environment, the proposed scheme demonstrated an approximately 10% higher accuracy and approximately five times faster training time.
更多查看译文
关键词
ultra-wideband (UWB),deep learning,transfer learning,non-line-of-sight (NLOS),wireless channel,spatial awareness
AI 理解论文
溯源树
样例
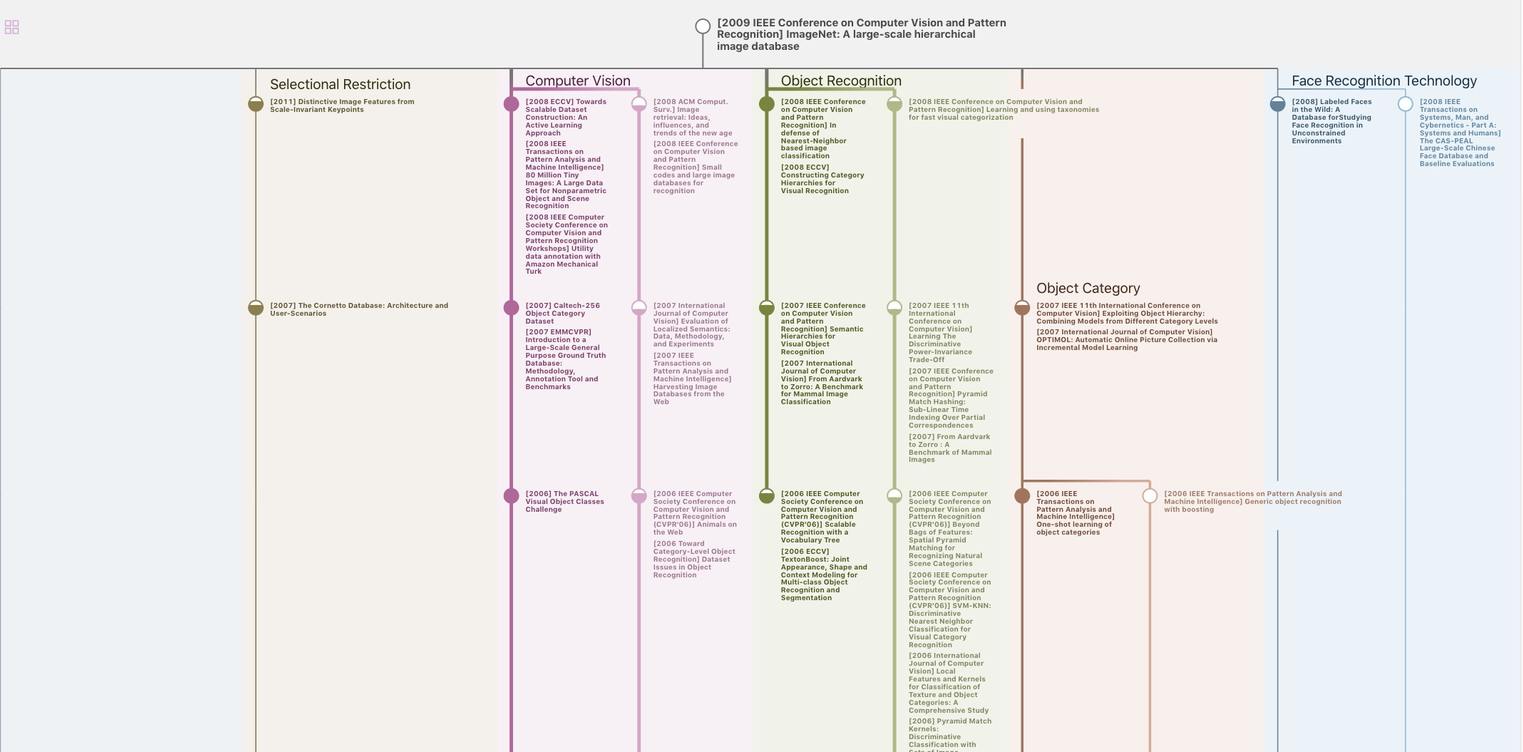
生成溯源树,研究论文发展脉络
Chat Paper
正在生成论文摘要