Human Activity Recognition Based on Gramian Angular Field and Deep Convolutional Neural Network
IEEE Access(2020)
摘要
With the development of the Internet of things (IoT) and wearable devices, the sensor-based human activity recognition (HAR) has attracted more and more attentions from researchers due to its outstanding characteristics of convenience and privacy. Meanwhile, deep learning algorithms can extract high-dimensional features automatically, which makes it possible to achieve the end-to-end learning. Especially the convolutional neural network (CNN) has been widely used in the field of computer vision, while the influence of environmental background, camera shielding, and other factors are the biggest challenges to it. However, the sensor-based HAR can circumvent these problems well. Two improved HAR methods based on Gramian angular field (GAF) and deep CNN are proposed in this paper. Firstly, the GAF algorithm is used to transform the one-dimensional sensor data into the two-dimensional images. Then, through the multi-dilated kernel residual (Mdk-Res) module, a new improved deep CNN network Mdk-ResNet is proposed, which extracts the features among sampling points with different intervals. Furthermore, the Fusion-Mdk-ResNet is adopted to process and fuse data collected by different sensors automatically. The comparative experiments are conducted on three public activity datasets, which are WISDM, UCI HAR and OPPORTUNITY. The optimal results are obtained by using the indexes such as accuracy, precision, recall and F-measure, which verifies the effectiveness of the proposed methods.
更多查看译文
关键词
Deep convolutional neural network,Gramian angular field,human activity recognition,multi-source sensor data fusion
AI 理解论文
溯源树
样例
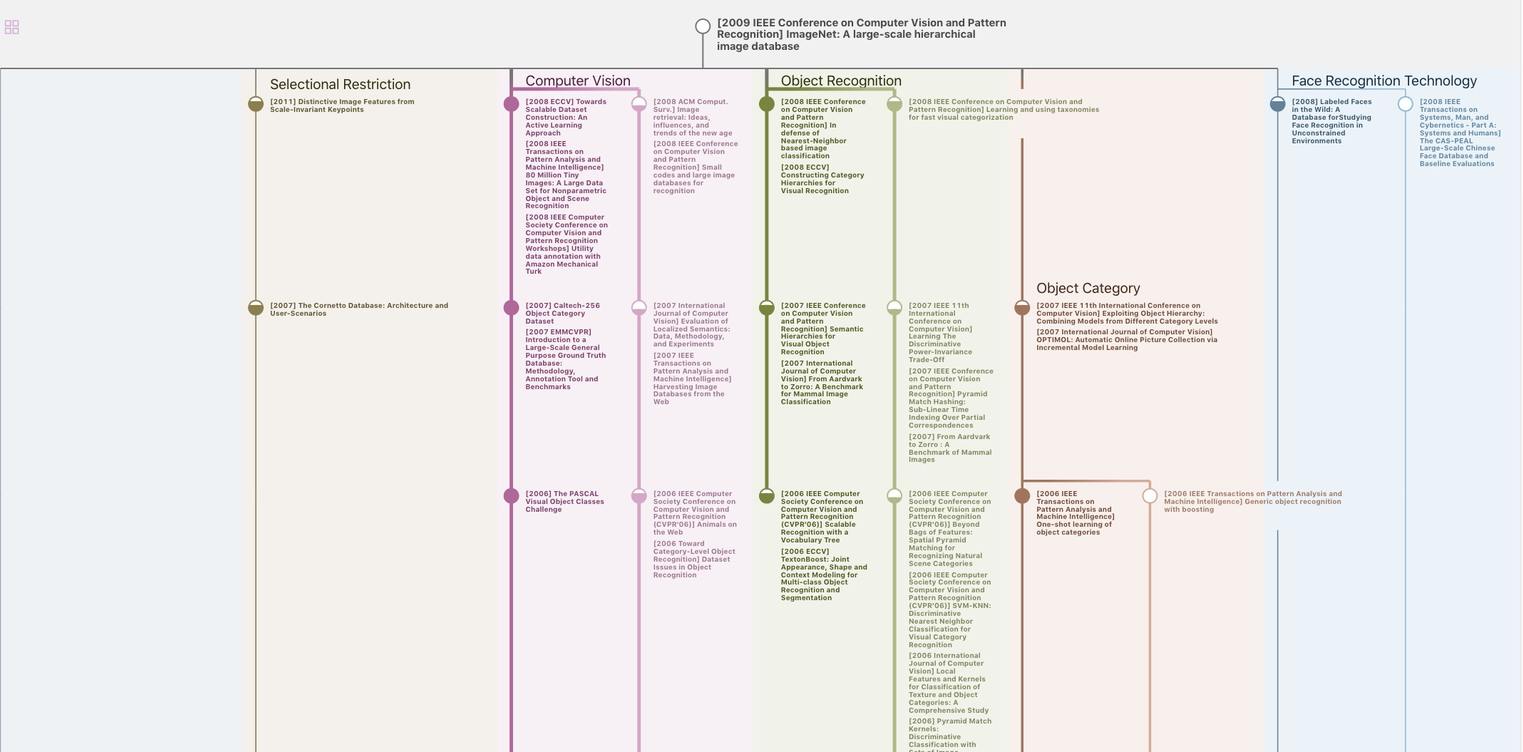
生成溯源树,研究论文发展脉络
Chat Paper
正在生成论文摘要