Conditional random fields as message passing mechanism in anchor-free network for multi-scale pedestrian detection
Information Sciences(2021)
摘要
Many deep learning detectors have been proposed to address the scale variation issue in pedestrian detection. However, it is still far from being fully solved because most of these detectors handle the issue by directly fusing multiple extracted features with the weighted average or concatenation. Therefore, this paper designs a novel and effective anchor-free network (namely MPAF-Net) for multi-scale pedestrian detection. Specifically, we first improve Res2Net as our backbone network by designing a novel bottleneck block to make the proposed detector has a strong multi-scale representation ability for pedestrians. Furthermore, based on Conditional Random Fields (CRFs), the multi-scale features obtained from three different feature pyramid levels of backbone network are refined with a designed message passing mechanism. By doing so, the complementary information from the features at other scales can be dynamically passed to enhance the scale-specific feature. Finally, pedestrian detection is simplified as a straightforward center and scale prediction task by performing convolution on the concatenation of refined multi-scale convolution features. This way, the proposed MPAF-Net can be trained in an end-to-end fashion. Experimental results show that MPAF-Net achieves state-of-the-art performance on CityPersons, Caltech, and CrowdHuman benchmarks.
更多查看译文
关键词
Multi-scale pedestrian detection,Anchor-free,Conditional random fields,Message passing mechanism
AI 理解论文
溯源树
样例
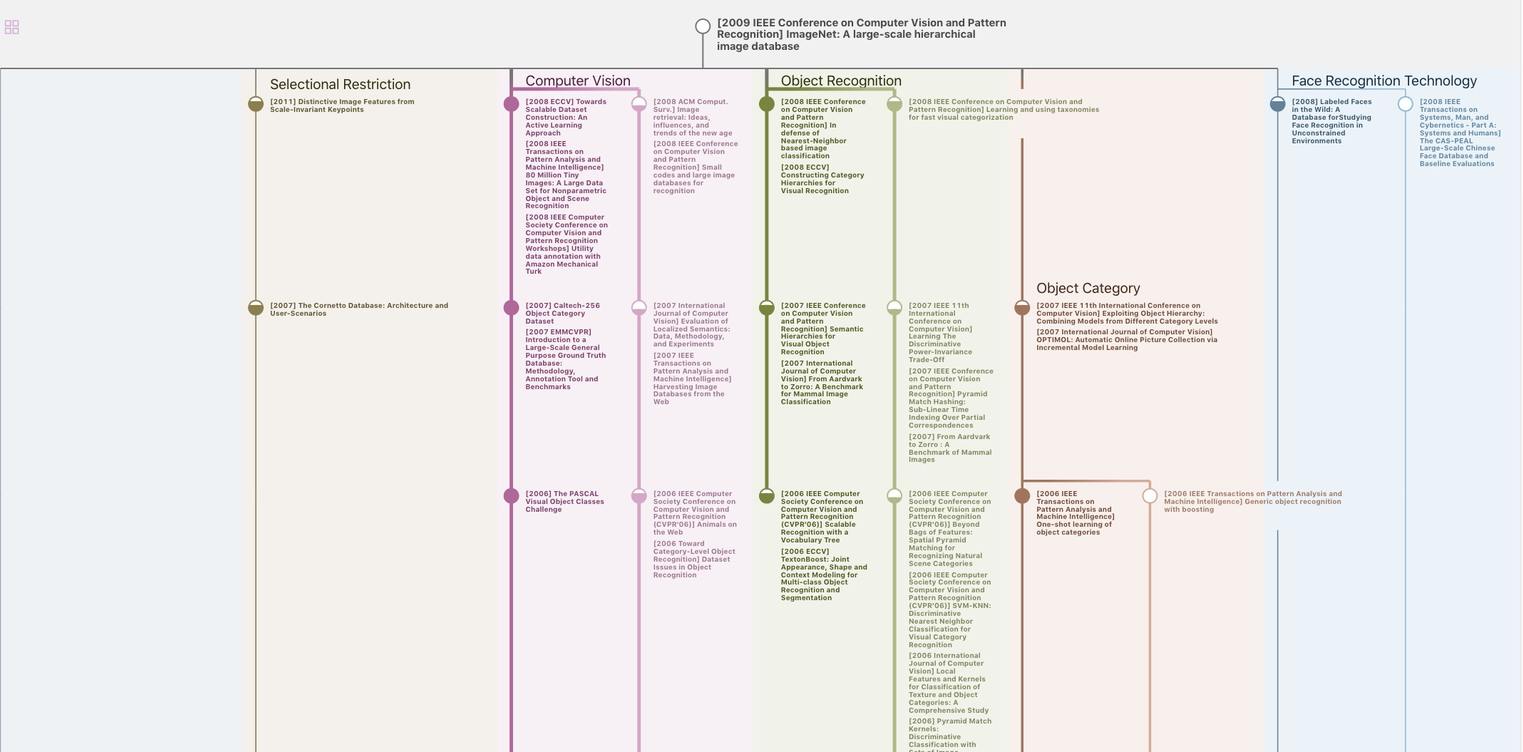
生成溯源树,研究论文发展脉络
Chat Paper
正在生成论文摘要