Predicting Hypertensive Patients With Higher Risk Of Developing Vascular Events Using Heart Rate Variability And Machine Learning
IEEE ACCESS(2020)
摘要
The prognosis of cardiovascular and cerebrovascular events for patients suffering from hypertension is considered of a high importance in preventing any further development of cardiac diseases. Despite of the ability of current gold standard techniques in predicting vascular events risks, they still lack the required clinical efficiency. In this vein, the study proposed herein provides an investigation on the feasibility of using heart rate variability (HRV) utilized through a machine learning approach to predict hypertensive patients at higher risk of developing vascular events. Initially, HRV features were extracted from all patient's data using time-domain, frequency-domain, non-linear, and fragmentation metrics. The extraction of features was based on a 24-hour cycle analysis segmented into four time periods; namely late-night, early-morning, afternoon, and evening. Analysis of all features was performed using a one-way analysis of variance (ANOVA) test on period by period basis. Furthermore, the selection of best features was performed following a Chi-squared test for demographic and HRV features. Then, a model based on decision trees and random under-sampling boosting (RUSBOOST) was trained using demographic features, HRV features, and a combination of both features. The performance of the trained model achieved a maximum accuracy of 97.08% using the combined set of features during the afternoon time period. In addition, the precision and F1-score in predicting high risk patients reached 81.25% and 86.67%, respectively. The overall area under the curve for the model was at 0.98, suggesting a high performance in the sensitivity and specificity measures. This study paves the way towards utilizing machine learning models and heart rate variability for the prognosis of vascular events in hypertensive patient. Furthermore, it assists clinicians in decision making by providing a simple, yet effective, and continuous prediction approach when compared to other available techniques.
更多查看译文
关键词
Hypertension, cardiovascular events, cerebrovascular events, heart rate variability, ANOVA, machine learning, feature selection, RUSBOOST
AI 理解论文
溯源树
样例
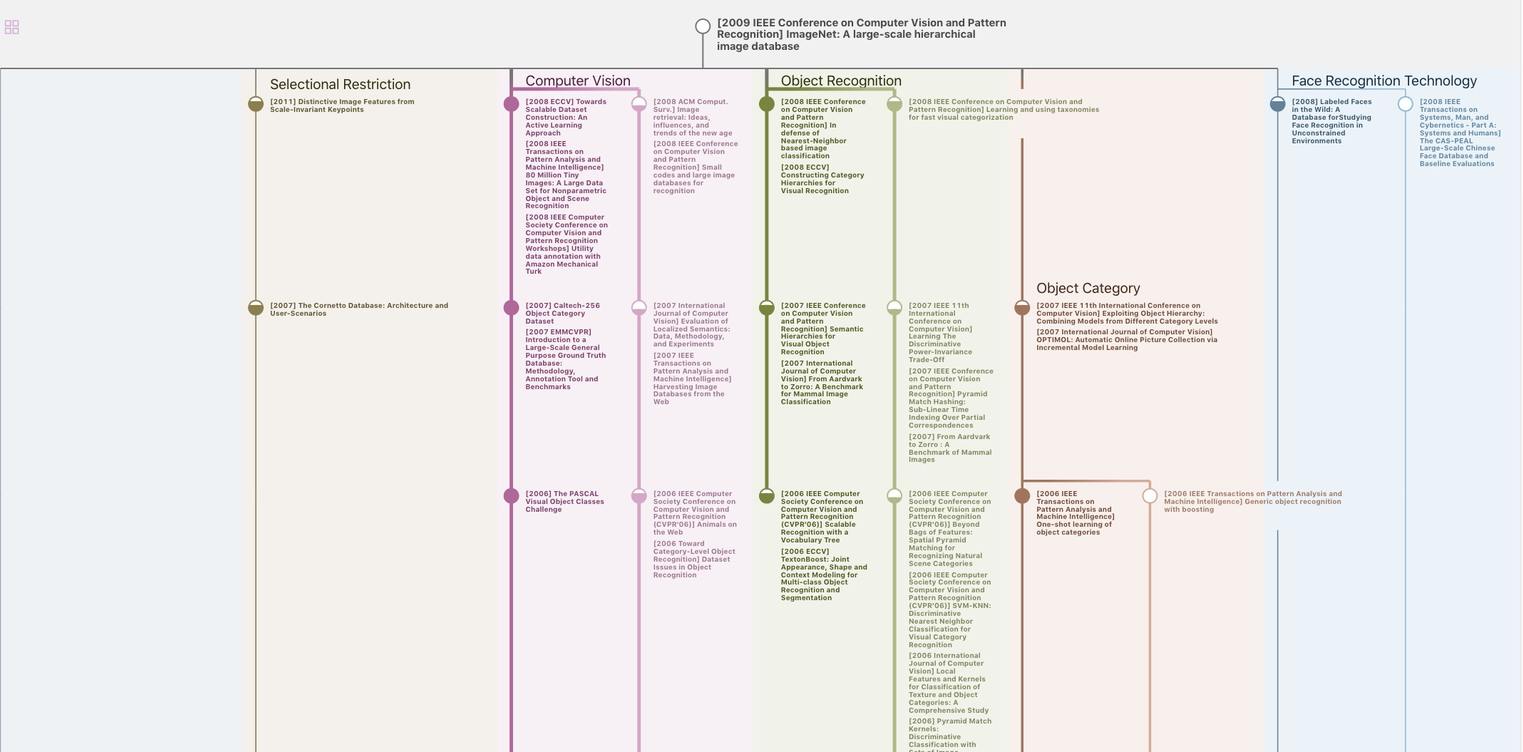
生成溯源树,研究论文发展脉络
Chat Paper
正在生成论文摘要