A general two-phase Markov chain Monte Carlo approach for constrained design optimization: Application to stochastic structural optimization
Computer Methods in Applied Mechanics and Engineering(2021)
摘要
This contribution presents a general approach for solving structural design problems formulated as a class of nonlinear constrained optimization problems. A Two-Phase approach based on Bayesian model updating is considered for obtaining the optimal designs. Phase I generates samples (designs) uniformly distributed over the feasible design space, while Phase II obtains a set of designs lying in the vicinity of the optimal solution set. The equivalent model updating problem is solved by the transitional Markov chain Monte Carlo method. The proposed constraint-handling approach is direct and does not require special constraint-handling techniques. The population-based stochastic optimization algorithm generates a set of nearly optimal solutions uniformly distributed over the vicinity of the optimal solution set. The set of optimal solutions provides valuable sensitivity information. In addition, the proposed scheme is a useful tool for exploration of complex feasible design spaces. The general approach is applied to an important class of problems. Specifically, reliability-based design optimization of structural dynamical systems under stochastic excitation. Numerical examples are presented to evaluate the effectiveness of the proposed design scheme.
更多查看译文
关键词
Constrained optimization,Feasible design space,Meta-models,Markov sampling method,Reliability-based design,Stochastic optimization
AI 理解论文
溯源树
样例
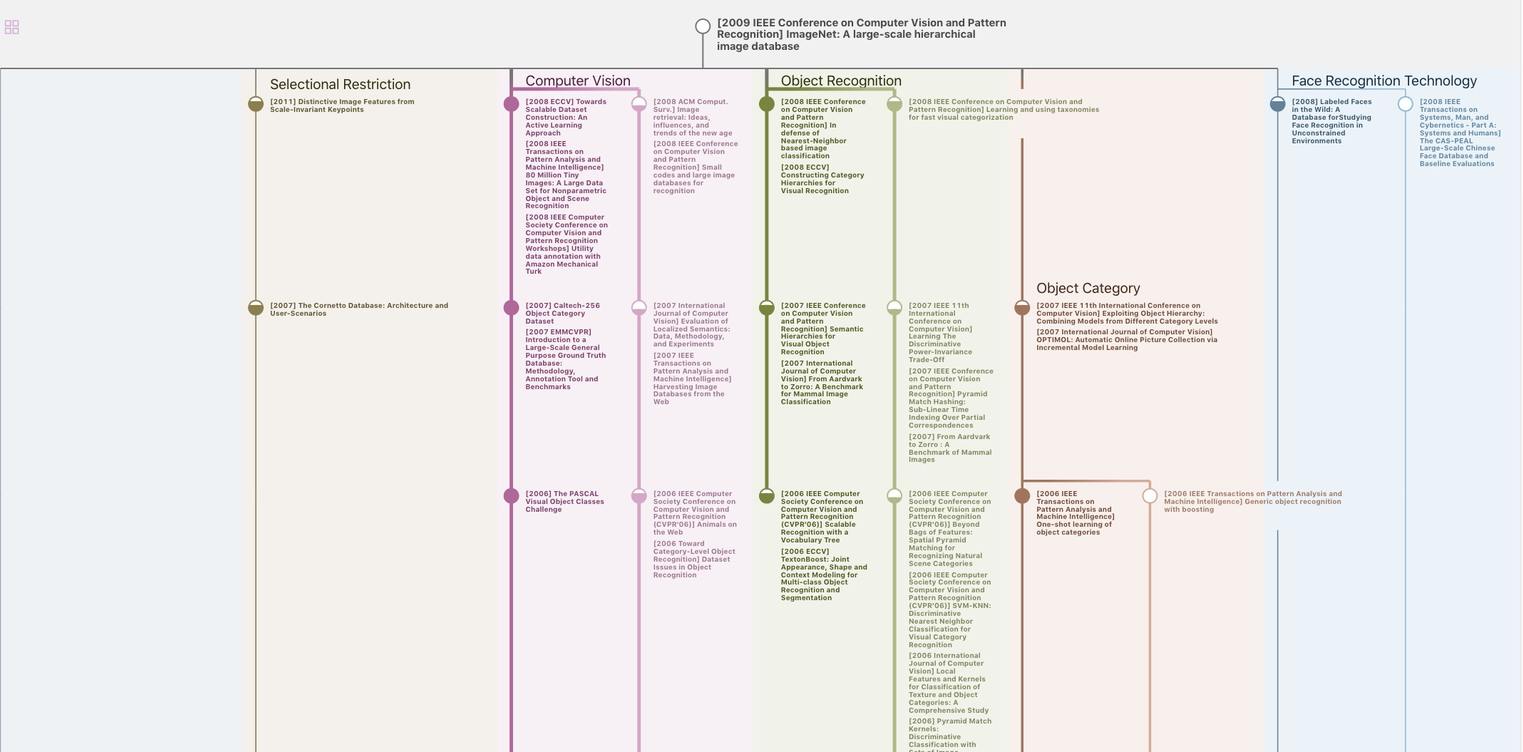
生成溯源树,研究论文发展脉络
Chat Paper
正在生成论文摘要