Automated Platform for Microseismic Signal Analysis: Denoising, Detection, and Classification in Slope Stability Studies
IEEE transactions on geoscience and remote sensing(2021)
摘要
Microseismic monitoring has been increasingly used in the past two decades to illuminate (sub)surface processes, such as landslides, due to its ability to record small seismic waves generated by soil movement and/or brittle behavior of rock. Understanding the evolution of landslide processes is of paramount importance in predicting or even avoiding an imminent failure. Microseismic monitoring recordings are often continuous, noisy, and consist of signals emitted by various sources. Manually detecting and distinguishing the signals emitted by an unstable slope is challenging. Research on automated end-to-end denoising, detection, and classification of microseismic events, as an early warning system, is still in its infancy. To this effect, our work is focused on jointly evaluating and developing suitable approaches for signal denoising, accurate event detection, nonsite-specific feature construction, feature selection, and event classification. We propose an automated end-to-end system that can process big data sets of continuous seismic recordings fast and demonstrate applicability and robustness to a wide range of events (distant and local earthquakes, slidequakes, anthropogenic noise, etc.). Algorithmic contributions lie in novel signal processing and analysis methods with fewer tunable parameters than the state of the art, evaluated on two field data sets and benchmarked against the state of the art.
更多查看译文
关键词
Landslides,Noise reduction,Signal processing algorithms,Benchmark testing,Soil,Signal processing,Feature extraction,Graph-based bilateral filter,microseismic,Neyman-Pearson lemma,graph Laplacian regularization (GLR) classification
AI 理解论文
溯源树
样例
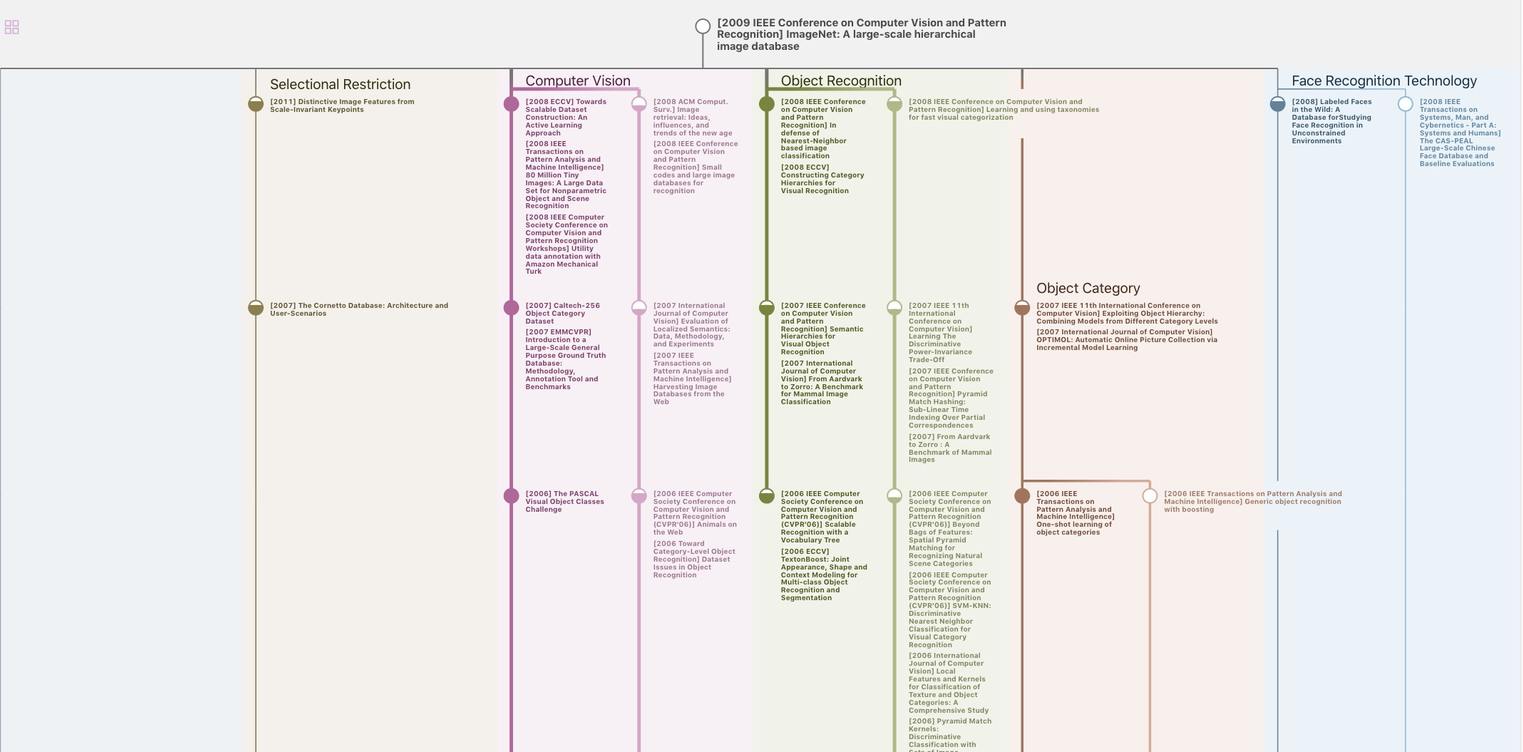
生成溯源树,研究论文发展脉络
Chat Paper
正在生成论文摘要