Prediction and Characterization of Flooding in Pulsed Sieve Plate Extraction Columns Using Data-Driven Models
INDUSTRIAL & ENGINEERING CHEMISTRY RESEARCH(2020)
摘要
Reliable prediction of flooding conditions is needed for sizing and operation of sieve plate extraction columns. Due to the complex interplay of chemical properties, the extraction column geometry and material and the pulsation intensity, the development of physical models and semiempirical correlations for a broad validity range is complicated. Available models and correlations may fail in predicting the flooding curve accurately. To overcome this problem, a data-driven model has been developed, which is capable of predicting flooding with a higher accuracy than conventional correlations from the literature. The optimized black-box approach, a purely data-driven approach, is a Gaussian process with a root mean squared error of 1.65 x 10(-3 )m/s and a coefficient of determination of 0.942. The combination of the data-driven model with additional physical models improves the accuracy not significantly. This gray-box approach results in a Gaussian process with a root mean squared error of 1.61 x 10(-3) m/s and a coefficient of determination of 0.944. The data-driven model is able to calculate correct flooding curves for different representative chemical systems and extraction column geometries.
更多查看译文
关键词
extraction,flooding,data-driven
AI 理解论文
溯源树
样例
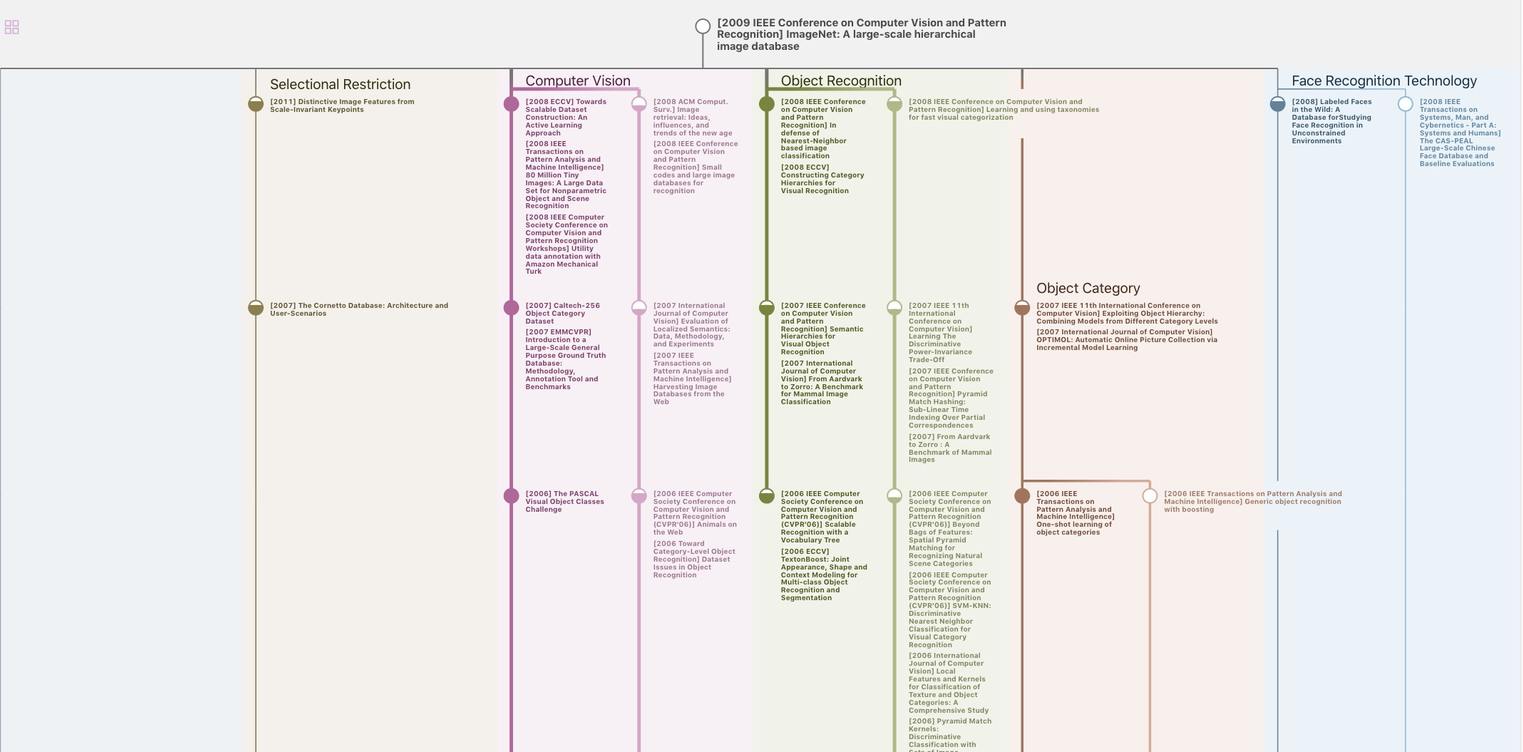
生成溯源树,研究论文发展脉络
Chat Paper
正在生成论文摘要