Physics-augmented Neural Compact Model for Emerging Device Technologies
2020 International Conference on Simulation of Semiconductor Processes and Devices (SISPAD)(2020)
摘要
This paper proposes a novel compact modeling framework based on artificial neural networks and physics informed machine learning techniques. This physics- augmented neural compact model shows highly accurate fitting abilities and physically consistent inferences even at the unseen data. It is also scalable and technology independent, and consequently, is suitable for electrical modeling of new emerging devices. In addition, this neural compact model is able to cover both digital and analog circuit analysis due to the weight decay regularization as well as high order derivative losses. Finally, it is applied to promising DRAM and Logic technologies to be evaluated in terms of its scalability and fitting accuracy. The CMC's (Compact Model Coalition) standard model API (Application Programming Interface) supports the custom model implementation for SPICE. Therefore, this framework enables the circuit simulators to assess technology-independent PPA (Power, Performance, Area) and early-stage DTCO (Design Technology Cooptimization) for new emerging devices.
更多查看译文
关键词
Compact Model,PDK,SPICE Circuit Simulation,Machine Learning,Artificial Neural Networks,Physical theory augmentation,Emerging device modeling,PPA and DTCO
AI 理解论文
溯源树
样例
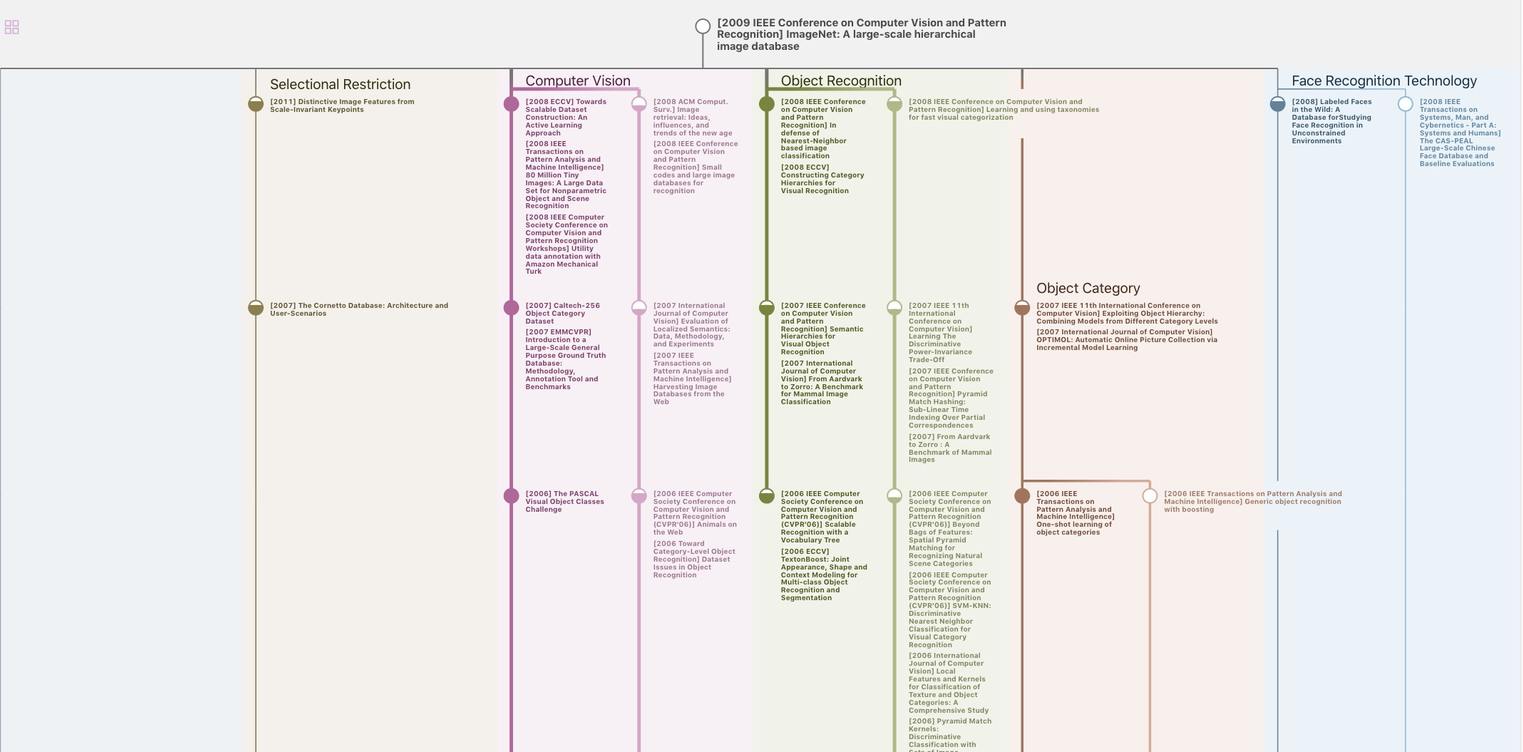
生成溯源树,研究论文发展脉络
Chat Paper
正在生成论文摘要