RUL prediction for automatic machines: a mixed edge-cloud solution based on model-of-signals and particle filtering techniques
JOURNAL OF INTELLIGENT MANUFACTURING(2020)
摘要
This work aims to provide useful insights into the course of action and the challenges faced by machine manufacturers when dealing with the actual application of Prognostics and Health Management procedures in industrial environments. Taking into account the computing capabilities and connectivity of the hardware available for smart manufacturing, we propose a particular solution that allows meeting one of the essential requirements of intelligent production processes, i.e., autonomous health management. Indeed, efficient and fast algorithms, that does not require a high computational cost and can be appropriately performed on machine controllers, i.e., on edge, are combined with others, which can handle large amounts of data and calculations, executed on remote powerful supervisory platforms, i.e., on the cloud. In detail, new condition monitoring algorithms based on Model-of-Signals techniques are developed and implemented on local controllers to process the raw sensor readings and extract meaningful and compact features, according to System Identification rules and guidelines. These results are then transmitted to remote supervisors, where Particle Filters are exploited to model components degradation and predict their Remaining Useful Life. Practitioners can use this information to optimise production planning and maintenance policies. The proposed architecture allows keeping the communication traffic between edge and cloud in the nowadays affordable “Big data” range, preventing the unmanageable “Huge data” scenario that would follow from the transmission of raw sensor data. Furthermore, the robustness and effectiveness of the proposed method are tested considering a meaningful benchmark, the PRONOSTIA dataset, allowing reproducibility and comparison with other approaches.
更多查看译文
关键词
Condition monitoring, Automatic machines, Edge-computing, System identification, Prognostics and health management, Particle filtering
AI 理解论文
溯源树
样例
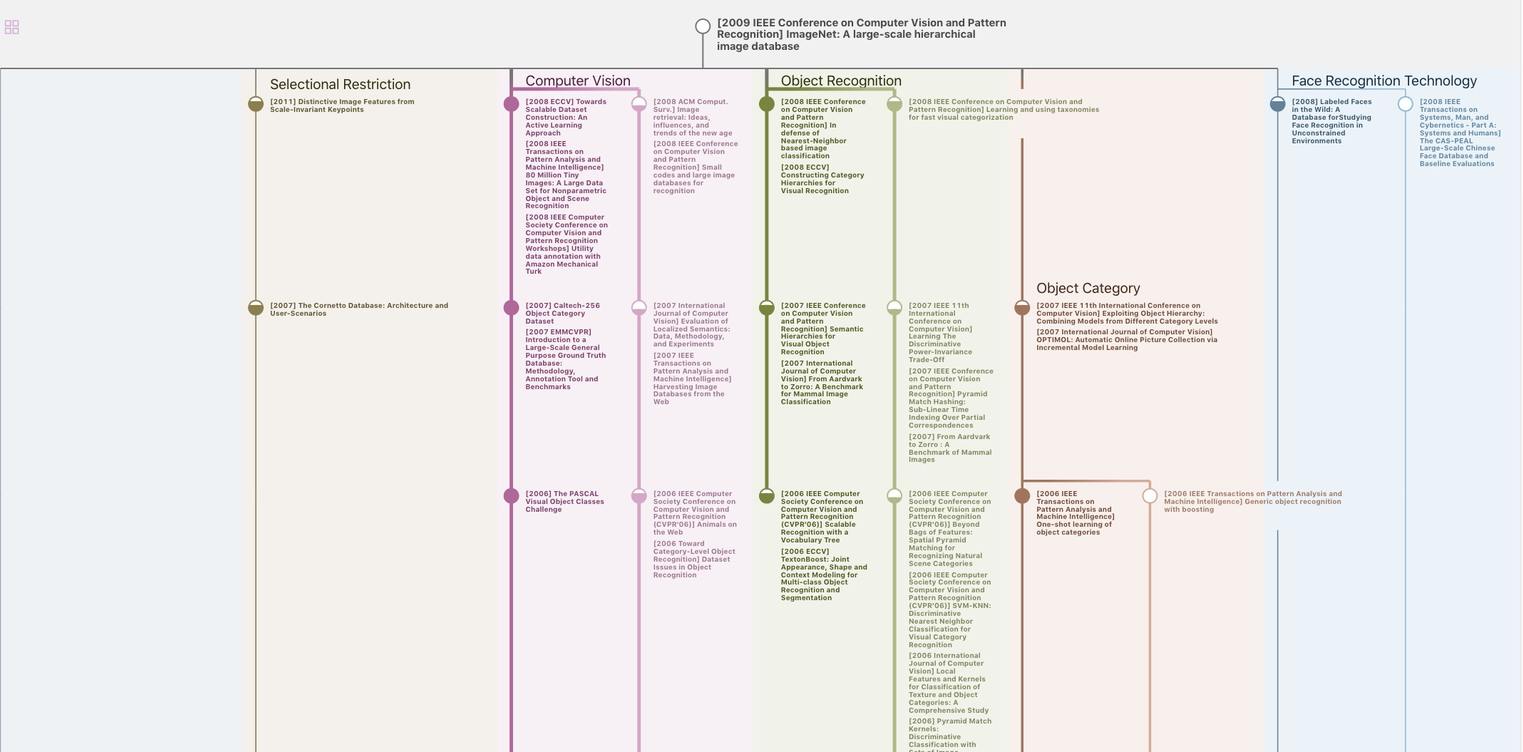
生成溯源树,研究论文发展脉络
Chat Paper
正在生成论文摘要