Temporal Knowledge Graph Incremental Construction Model for Recommendation.
Interational Conference on Web-Age Information Management(2020)
摘要
Knowledge graph (KG) has been proven to be effective to improve the performance of recommendation because of exploiting structural and semantic paths information in a static knowledge base. However, the KG is an incremental construction process with interactions occurring in succession. Although some works have been proposed to explore the evolution of knowledge graph, which updates the entity representations by considering the previous interactions of related entities. However, we believe that the semantic path information between the involved entities and the occurring interaction itself also can refine their representations. To this end, we propose a temporal knowledge graph incremental construction model, which updates the entity representations by considering interaction itself and high-order semantic paths information. Specifically, different length semantic paths between user and item are automatically extracted when an interaction occurs. Then we respectively employ recurrent neural network and standard multi-layer perceptron (MLP) to capture different length path semantic information and interaction itself information for updating the entity representations. Finally, we use MLP to predict the probability that a user likes an item after seamlessly integrating these variations into a unified representation. We conduct experiments on real-world datasets to demonstrate the superiority of our proposed model over all state-of-the-art baselines.
更多查看译文
关键词
Knowledge graph, Semantic path, User interaction, Recurrent neural network
AI 理解论文
溯源树
样例
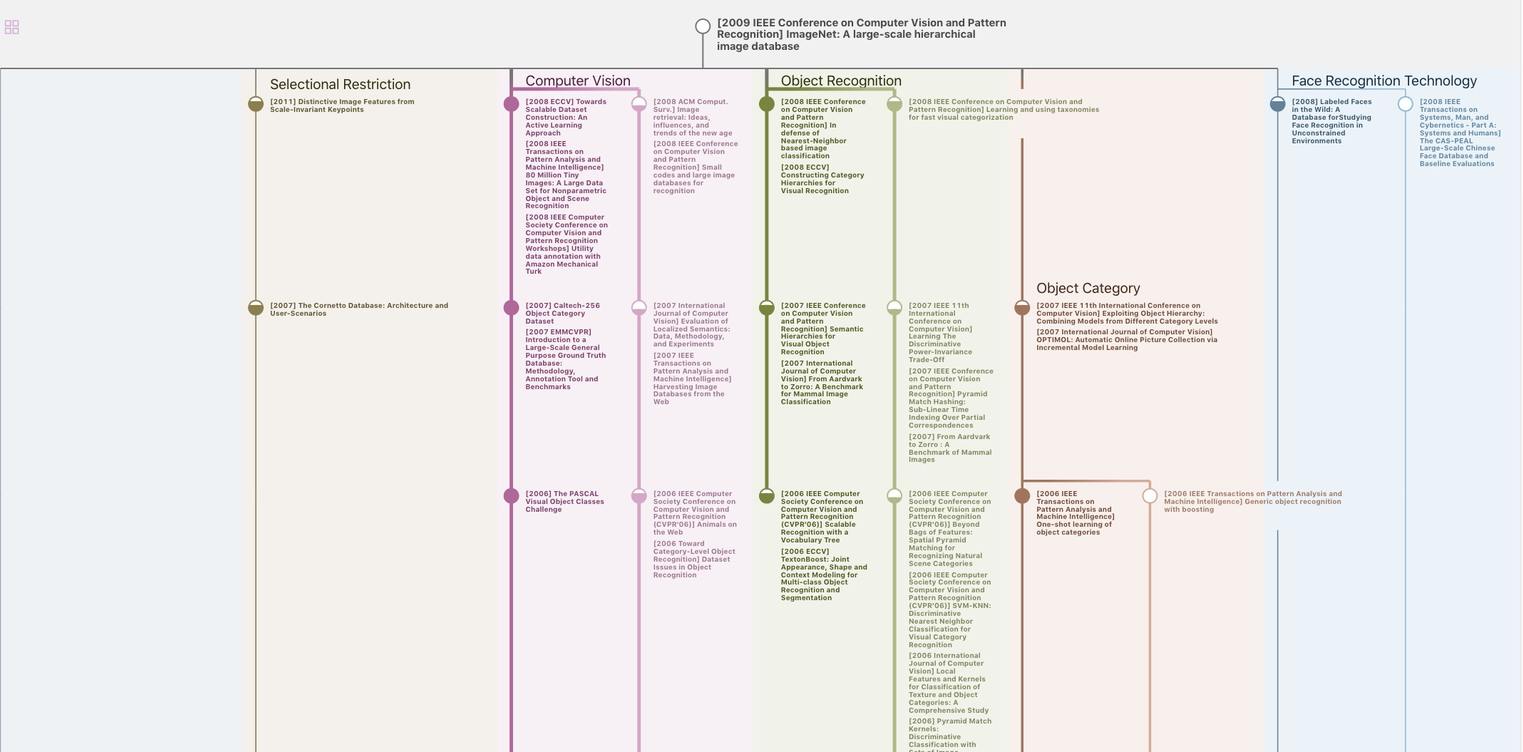
生成溯源树,研究论文发展脉络
Chat Paper
正在生成论文摘要