Synthesizing images from deep neural networks to map the hierarchy of feature complexity in human visual cortex
Journal of Vision(2020)
Abstract
Vision science often confronts the challenge of choosing stimuli that target particular stages of visual processing and whose similarity and structure can be quantified objectively. Here we leverage deep neural networks as crude approximations of the visual processing hierarchy to synthesize images containing features at any level of complexity and with a known representational structure in the model. We then validate this approach by using these stimuli during fMRI to localize areas of visual cortex with representations corresponding to different model layers. In a first study, we targeted object-selective visual areas such as lateral occipital (LO) cortex by synthesizing image pairs that varied parametrically in representational similarity in higher model layers. We found that pattern similarity across voxels in LO between paired stimuli mirrored the parametric increase in representational similarity in the model. In a second study, we extended this approach by synthesizing a single image set with the objective that the representational spaces for these images in different model layers would be uncorrelated. That is, for the set of images, the correlation of their unit activity patterns in a layer produced an image-by-image similarity matrix in each layer, and we sought images for which these similarity matrices were maximally orthogonal across layers. The resulting layer-specific representational fingerprints could be compared against the similarity matrices of patterns of voxel activity evoked by presenting these images, allowing visual areas and searchlights to be mapped precisely to model layers. Preliminary results suggest a hierarchical mapping, wherein lower layers are most strongly expressed in the occipital pole and higher layers are expressed laterally and anteriorly. These algorithms provide a new way to generate rich stimulus sets that can be formalized in a model and used to efficiently localize and differentiate even adjacent stages of visual processing.
MoreTranslated text
Key words
visual cortex,feature complexity,deep neural networks
AI Read Science
Must-Reading Tree
Example
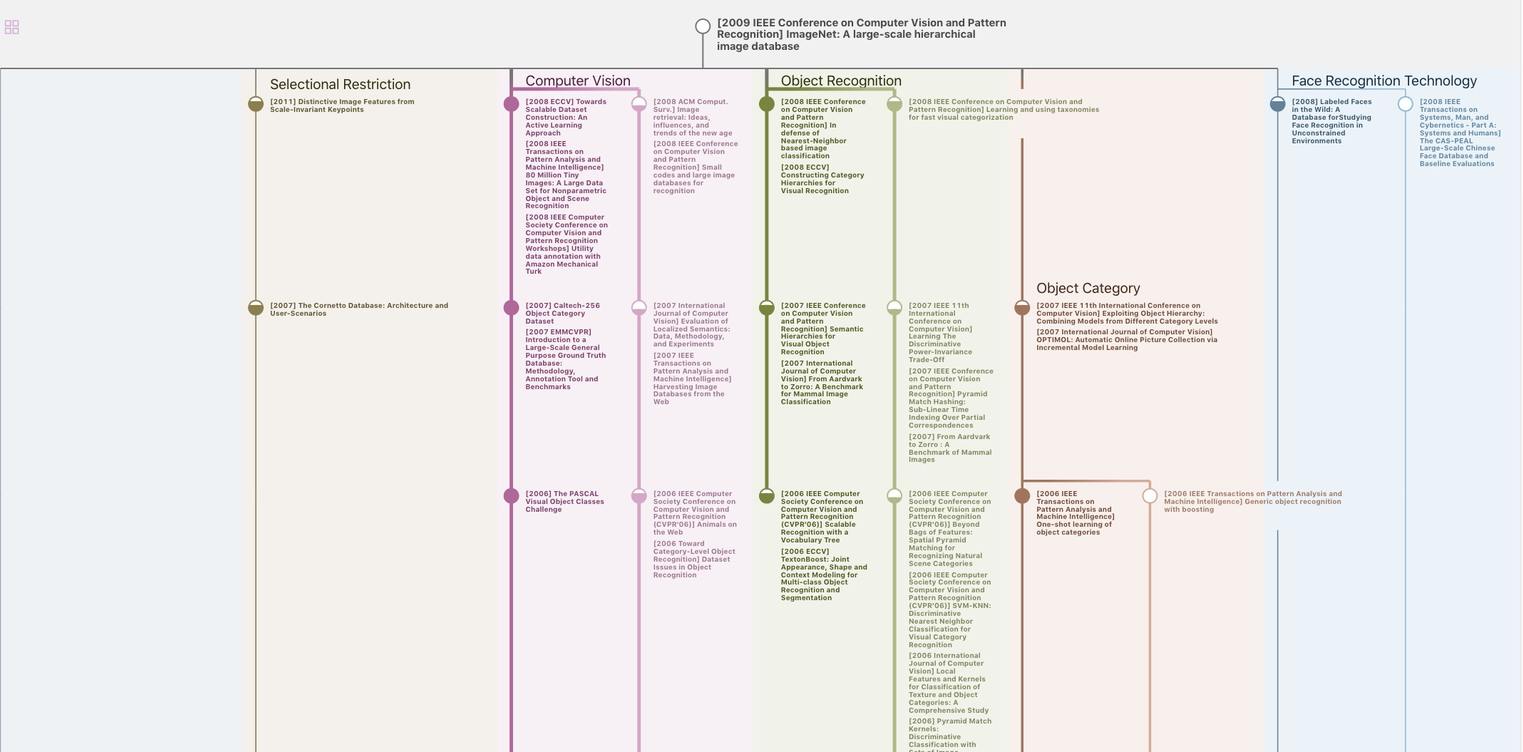
Generate MRT to find the research sequence of this paper
Chat Paper
Summary is being generated by the instructions you defined