Noise Resistant Focal Loss for Object Detection
chinese conference on pattern recognition(2020)
摘要
Noise robustness and hard example mining are two important aspects in object detection. A common view is that the two techniques are contradictory and they cannot be combined. In this paper, we show that there is a possibility to combine the best of two techniques. We find that, even using the hard example mining technique, recent deep neural network-based object detectors themselves have abilities to distinguish correct annotations and wrong annotations during the early stage of training. Based on this observation, we design a simple strategy to separate the wrong annotations from training data, reducing their loss weights and correcting their labels during training. The proposed method is simple, it doesn’t add any computational overhead during model inference. Moreover, the proposed method combines the hard example mining and noise resistance property in one model. Experiments on PASCAL VOC and DOTA datasets show that the proposed method not only archieves competitive performances on clean dataset, but also outperforms the baseline by a large margin when data contain severe noise.
更多查看译文
关键词
Noise robustness, Hard example mining, Focal loss, Noise resistant focal loss
AI 理解论文
溯源树
样例
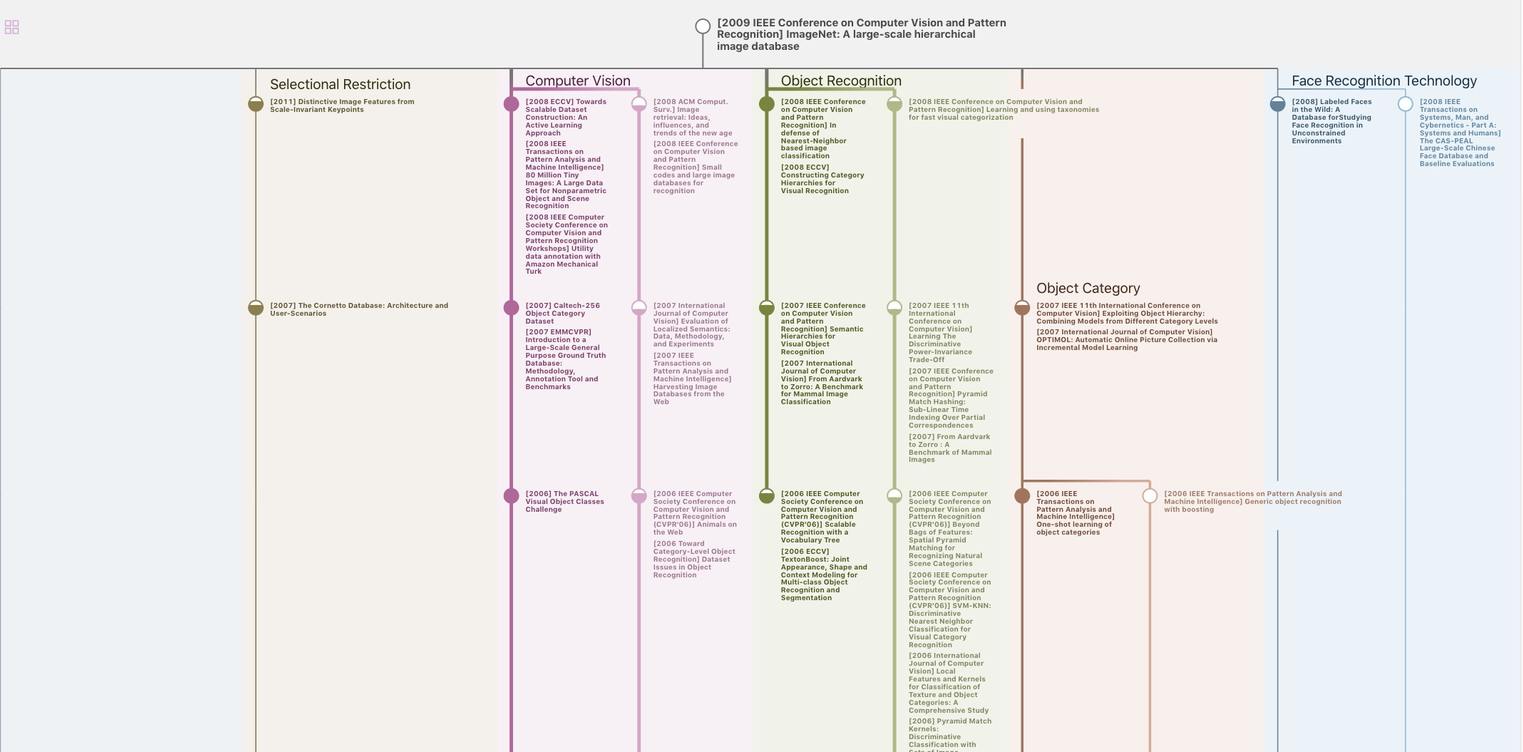
生成溯源树,研究论文发展脉络
Chat Paper
正在生成论文摘要