LST-Net: Learning a Convolutional Neural Network with a Learnable Sparse Transform
European Conference on Computer Vision(2020)
摘要
The 2D convolutional (Conv2d) layer is the fundamental element to a deep convolutional neural network (CNN). Despite the great success of CNN, the conventional Conv2d is still limited in effectively reducing the spatial and channel-wise redundancy of features. In this paper, we propose to mitigate this issue by learning a CNN with a learnable sparse transform (LST), which converts the input features into a more compact and sparser domain so that the spatial and channel-wise redundancy can be more effectively reduced. The proposed LST can be efficiently implemented with existing CNN modules, such as point-wise and depth-wise separable convolutions, and it is portable to existing CNN architectures for seamless training and inference. We further present a hybrid soft thresholding and ReLU (ST-ReLU) activation scheme, making the trained network, namely LST-Net, more robust to image corruptions at the inference stage. Extensive experiments on CIFAR-10/100, ImageNet, ImageNet-C and Places365-Standard datasets validated that the proposed LST-Net can obtain even higher accuracy than its counterpart networks with fewer parameters and less overhead.
更多查看译文
关键词
CNN,Network architecture,Learnable sparse transform
AI 理解论文
溯源树
样例
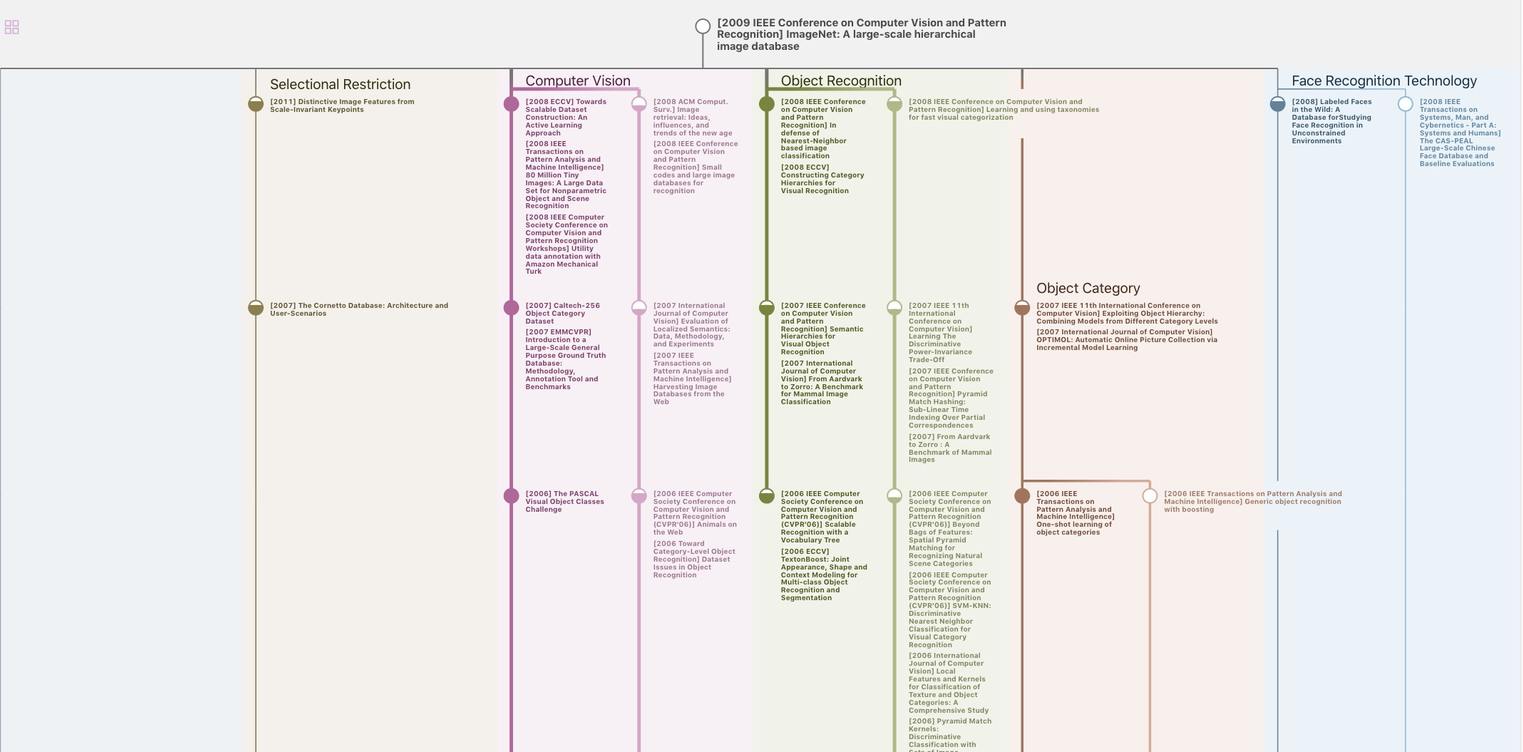
生成溯源树,研究论文发展脉络
Chat Paper
正在生成论文摘要