Mixing user-centered and generalized models for Fall Detection.
Neurocomputing(2021)
摘要
Fall Detection (FD) using wearable devices has been the focus of many research studies during the last years. Solutions are expected to work autonomously and securely; however, even the commercial products are still far from being reliable in elderly people autonomously everyday life. This research focuses on developing a FD method valid to be deployed on a wearable device with tri-axial accelerometer as sensing unit, and working autonomously without external services. Moreover, each fall type is considered independently and the solution adapts to its current user. The proposal includes three stages: a novel event detection stage followed by a one-class problem classification and a final classifier that labels the anomaly events as Fall or Normal. The process starts gathering data from the current user’s wearable provided the data does not include any fall; this data is used to tune the event detection and to train a one-class classifier. Two different methods are tested: a One-Class Support Vector Machine and a classifier based on the centroid of the normal activities’ instances. The detected anomaly events are then classified as Fall or Normal; this second classifier is previously obtained from data from other users’ past experiences. For this two-class model, two different options are tested as well: a Support Vector Machine and a feed-forward Neural Network. The experimentation stage includes two different published simulated falls data sets. The obtained results show that filtering the detected peaks corresponding to normal activities of the current user helps the two-class classifier for some types of fall events, suggesting to introduce specific one-class filtering per fall type. However, the number of false alarms is still high, and the two-class classifiers have a high variability in their performances according to the user, which still needs further research. The results suggest that it might be interesting to obtain a more accurate two-class classifier using data only from subjects with similar activity levels; the use of online learning might also improve the general performance in the classification stages.
更多查看译文
关键词
Fall Detection,Machine learning,One-class classifier,Event detection,Elderly population
AI 理解论文
溯源树
样例
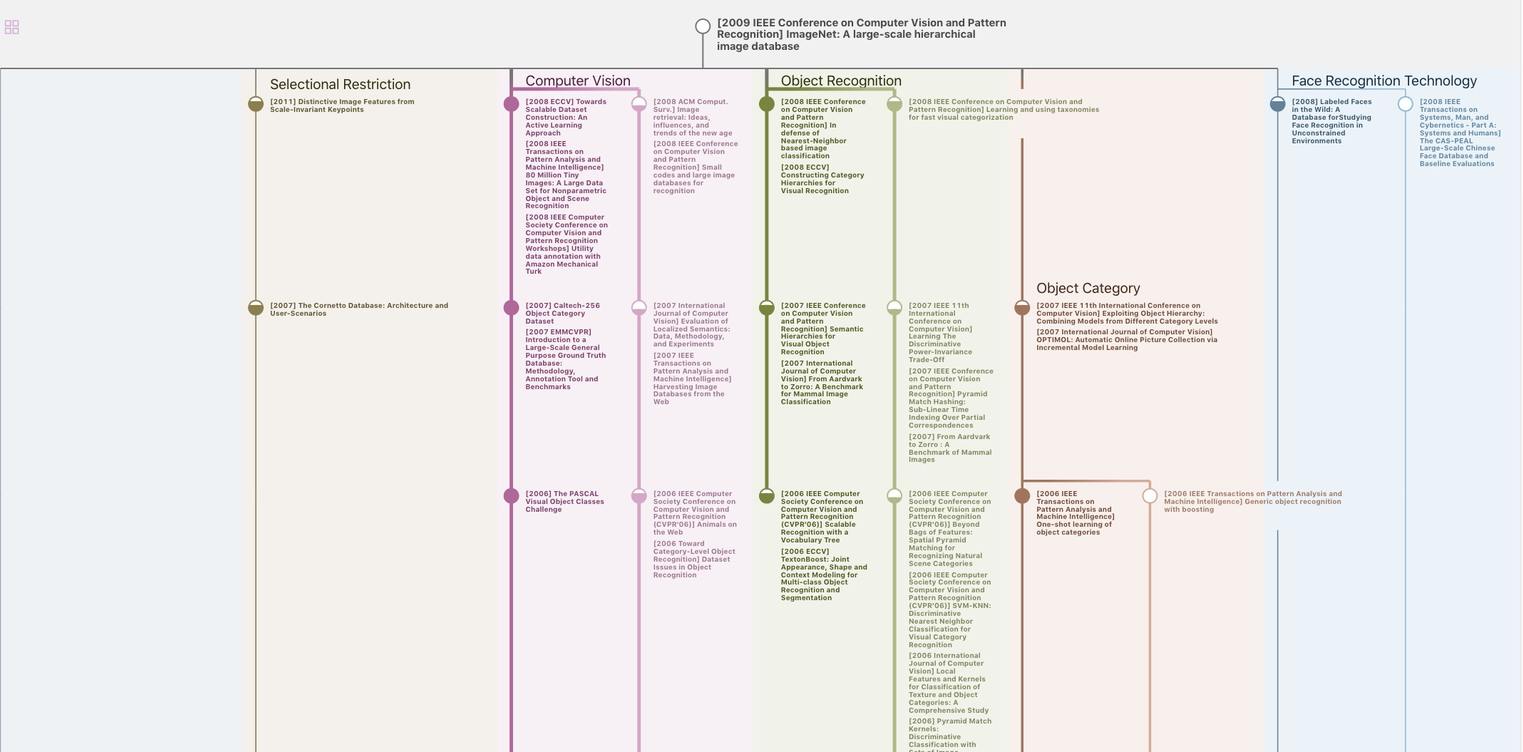
生成溯源树,研究论文发展脉络
Chat Paper
正在生成论文摘要