Assessing Action Quality via Attentive Spatio-Temporal Convolutional Networks.
PRCV (2)(2020)
摘要
Action quality assessment, which aims at evaluating the performance of specific actions, has drawn more and more attention due to its extensive demand in sports, health care, etc. Unlike action recognition, in which a few typical frames are sufficient for classification, action quality assessment requires analysis at a fine temporal granularity to discover the subtle motion difference. In this paper, we propose a novel spatio-temporal framework for action quality assessment at full-frame-rate (25fps), which consists of two steps: i.e. spatio-temporal feature extraction and temporal feature fusion, respectively. In the first step, to generate representative spatio-temporal dynamics, we utilize a spatial convolutional network (SCN) together with specially designed temporal convolutional networks (TCNs) and train them by a two-stage strategy. In the second step, we introduce an attention mechanism to fuse features in the temporal dimension according to their impact on the overall performance. Compared with existing three dimensional convolutional neural networks (3D-CNN) based methods, our model is capable of capturing more action quality relevant details. As a by-product, our model can also attend to the highlight moments in sports videos, which gives a better interpretation of the score. Extensive experiments on three public benchmarks demonstrate that the proposed method has distinct advantage in action quality assessment and achieves improvement over the state-of-the-art.
更多查看译文
关键词
action quality,spatio-temporal
AI 理解论文
溯源树
样例
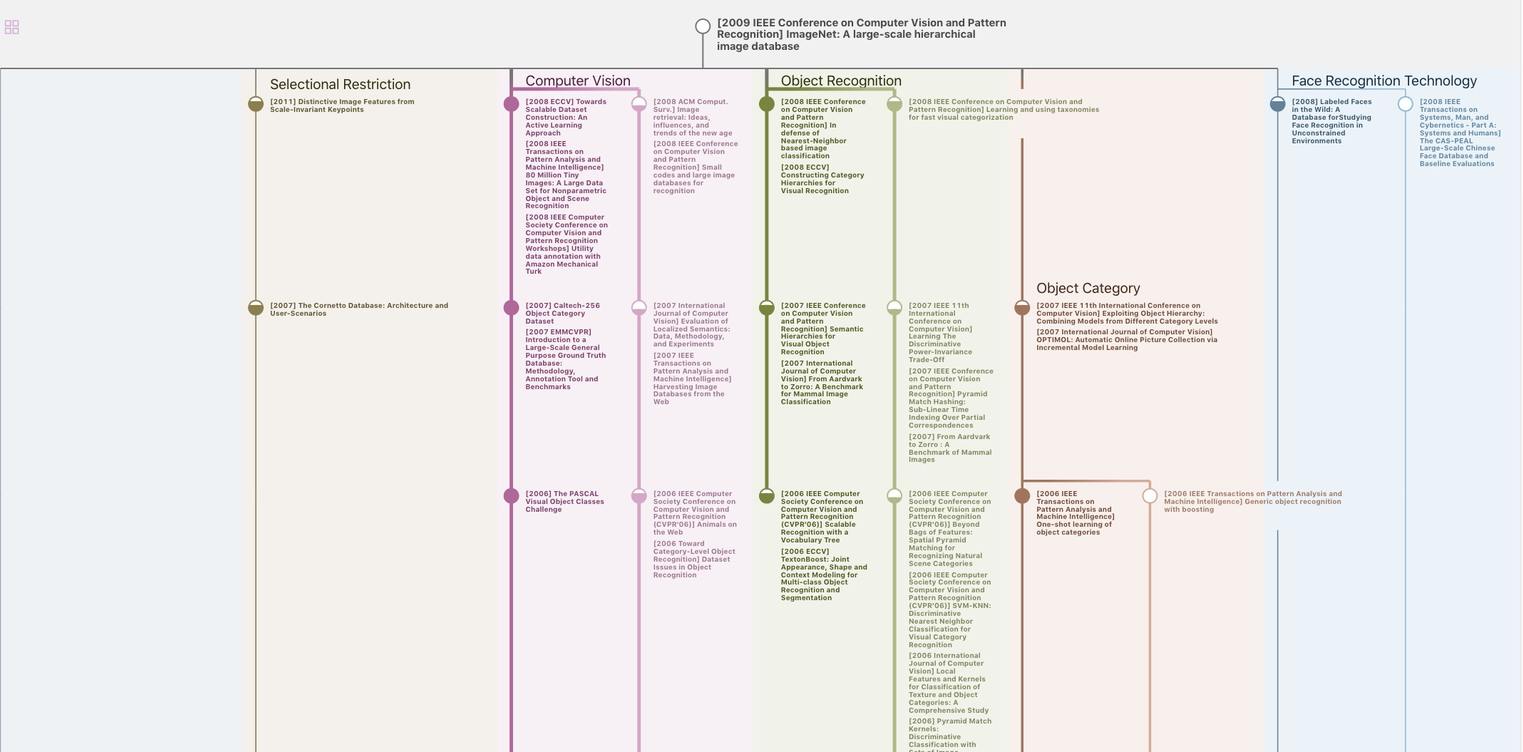
生成溯源树,研究论文发展脉络
Chat Paper
正在生成论文摘要