Robust dialog state tracker with contextual-feature augmentation
APPLIED INTELLIGENCE(2020)
摘要
Dialog state tracking (DST), which estimates dialog states given a dialog context, is a core component in task-oriented dialog systems. Existing data-driven methods usually extract features automatically through deep learning. However, most of these models have limitations. First, compared with hand-crafted delexicalization features, such features in deep learning approaches are not universal. However, they are important for tracking unseen slot values. Second, such models do not work well in situations where noisy labels are ubiquitous in datasets. To address these challenges, we propose a robust dialog state tracker with contextual-feature augmentation. Contextual-feature augmentation is used to extract generalized features; hence, it is capable of solving the unseen slot value tracking problem. We apply a simple but effective deep learning paradigm to train our DST model with noisy labels. The experimental results show that our model achieves state-of-the-art scores in terms of joint accuracy on the MultiWOZ 2.0 dataset. In addition, we show its performance in tracking unseen slot values by simulating unseen domain dialog state tracking.
更多查看译文
关键词
Human-machine interaction,Task-oriented dialog systems,Dialog state tracking,Contextual self-attention,Learning with noisy labels
AI 理解论文
溯源树
样例
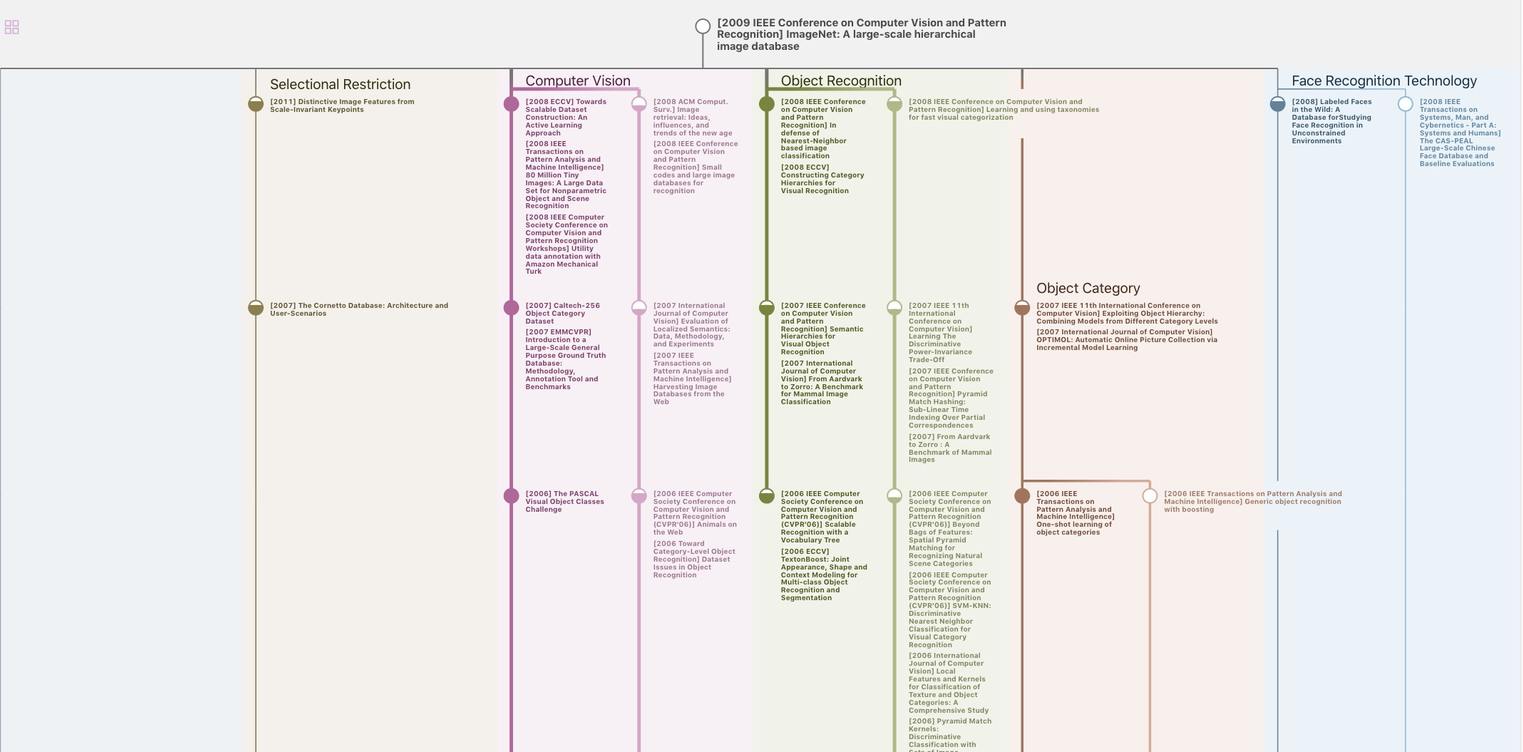
生成溯源树,研究论文发展脉络
Chat Paper
正在生成论文摘要