Leveraging Muscular Fitness Surrogates to Classify Cardiorespiratory Fitness Status in Youth: A Supervised Machine Learning Approach
international conference on human-computer interaction(2020)
摘要
Cardiorespiratory fitness (CRF) is linked with anxiety, depression, and cardiovascular disease risk. Assessing CRF is time consuming, space-prohibitive, and may require specialized equipment. Therefore, it is not routinely assessed in schools or clinical settings. This study aimed to leverage muscular fitness surrogates, anthropometrics and demographics to build optimal CRF classifiers that can be easily deployed across multiple settings. VO2PEAK was estimated using a prediction equation that integrated the 20-m Progressive Aerobic Cardiovascular Endurance Run dataset from 210 youth (116 males) (9.7 ± 1.08 years; 138.6 ± 9.4 cm; 42.3 ± 14.4 kg). Muscular fitness (i.e., 90° push-up, curl-up, etc.) was assessed. Several models (e.g., Support Vector Machine (SVM), Naïve Bayes, and Logistic Regression) were trained using both the originally imbalanced dataset and a balanced dataset resulting from synthetically oversampling the minority class. Metrics, including Area Under Curve (AUC) and True and False Positive Rates (TPR and FPR) were used to compare performance between models. The most salient model was a Logistic Regression model with nine features (i.e., sex, age, sit-and-reach, body mass, trunk lift, BMI, curl-up, 90° push-up, and height) (accuracy = 89.5%; AUC = 0.96; TPR = 0.86, FPRs = 0.08). This model correctly classified 100% and 100% of positive and negative class observations, respectively. A Logistic Regression model (accuracy = 85.7%; AUC = 0.92; TPR = 0.84, FPRs = 0.14) with four features (i.e., sex, age, sit-and-reach, and body mass) correctly predicted 100% and 91% of positive and negative class observations, respectively. Results demonstrate the feasibility of leveraging muscular fitness, anthropometrics, and basic demographics to develop accurate, streamlined models that can be easily deployed across multiple settings in order to facilitate routine CRF assessment in youth.
更多查看译文
关键词
Machine learning,Cardiorespiratory fitness classification,Weight status,Hispanic/latino youth,School fitness surveillance
AI 理解论文
溯源树
样例
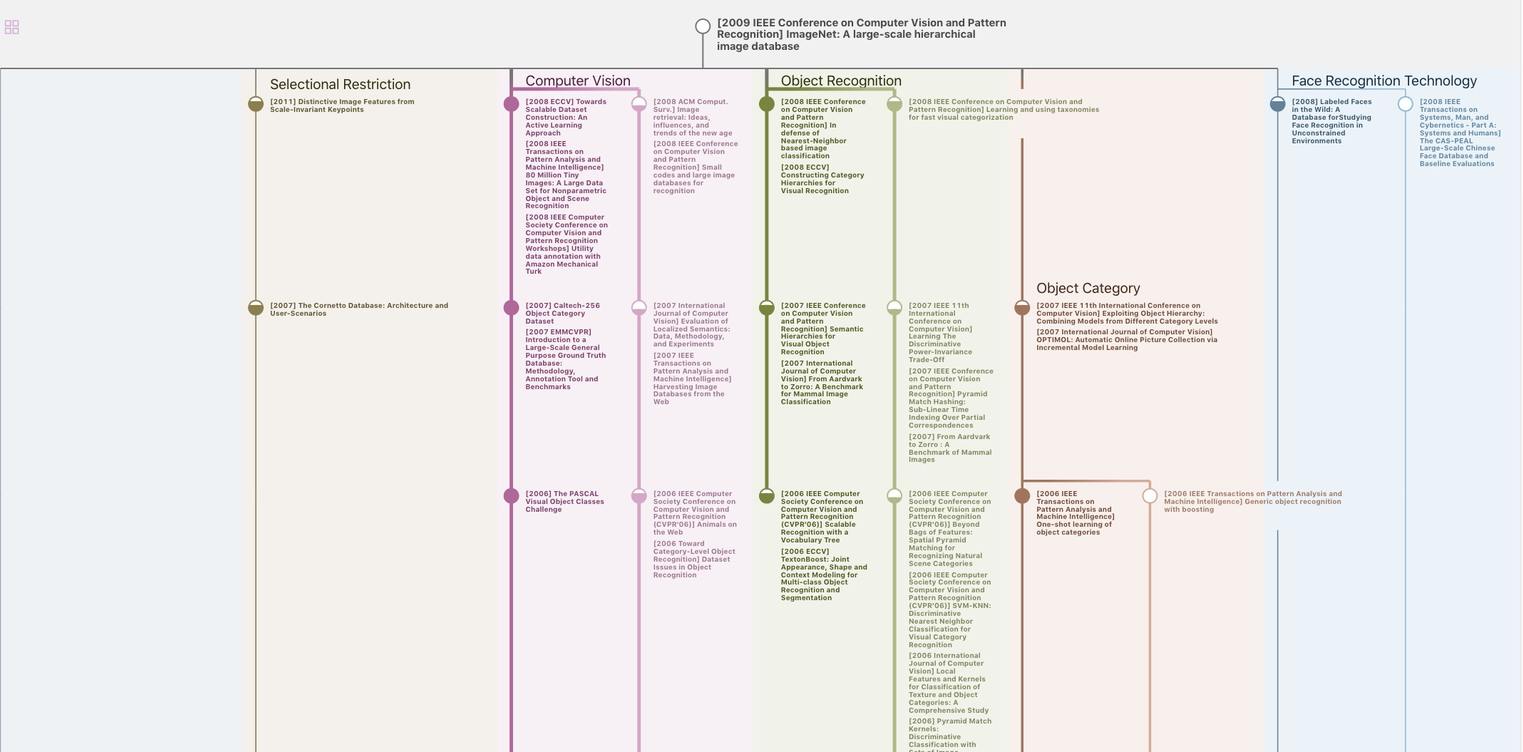
生成溯源树,研究论文发展脉络
Chat Paper
正在生成论文摘要