Behavioral Modeling Of Gan Doherty Power Amplifiers Using Memoryless Polar Domain Functions And Deep Neural Networks
IEEE ACCESS(2020)
摘要
In this paper, novel Doherty Power Amplifier (DPA) models are presented. The motivation behind the proposed models is to accurately predict static nonlinearities in the compression regions of the carrier and peaking amplifiers. DPAs suffer from a nonlinearity that originates from the carrier amplifier, and a second more pronounced nonlinearity generated at the full compression region following the turn-on of the peaking amplifier. Moreover, these distortions are often observed at different input power levels depending on whether the AM-AM or the AM-PM characteristic is considered. Therefore, the proposed static model is based on independent modeling of the memoryless gain in the polar domain. The static model of the memoryless AM-AM and AM-PM characteristics is augmented with either memory polynomials or deep neural network functions for memory effects modeling. The methodology of building the proposed models and the achieved results are discussed in this paper. The MP based proposed model achieves an NMSE as low as -45.3dB with only 78 model parameters, while the DNN based model achieves an NMSE as low as -46.1dB with only 156 model parameters. However, the DNN based model achieves the best model resilience to changes in the identification data.
更多查看译文
关键词
AM-AM, AM-PM, digital pre-distortion, Doherty power amplifier, linearization, memory effect, polynomial model, deep neural network, bidirectional LSTM, convolutional neural networks
AI 理解论文
溯源树
样例
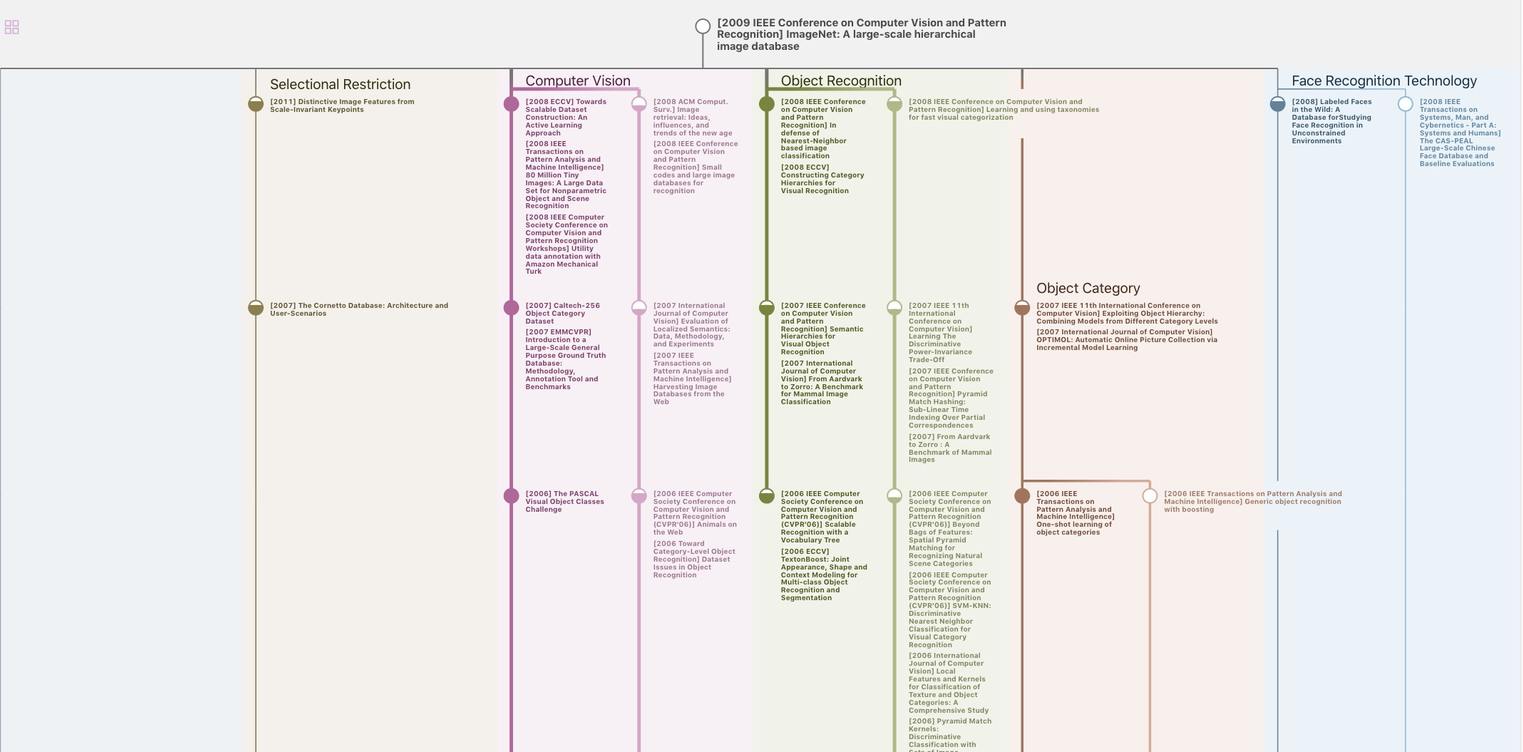
生成溯源树,研究论文发展脉络
Chat Paper
正在生成论文摘要