Activities of Daily Living Recognition With Binary Environment Sensors Using Deep Learning: A Comparative Study
IEEE SENSORS JOURNAL(2021)
摘要
The power of end-to-end deep learning techniques to automatically learn latent high-level features from raw signals has been demonstrated in numerous application fields, however, few studies systematically investigate how to properly encode the time-series firings of binary environment sensors that typically work in an event-triggering scheme and have irregular sampling rates for in-home human activity recognition. To this end, we here propose two different methods to process the streaming sensor readings and accordingly evaluate their combinations with deep learning models. Specifically, we divide the multichannel sensor events into segments and encode each segment into either a vector or two-dimensional matrix. Particularly, three different feature representations are presented for the vector form. Afterwards, we combine the encoded features with four typical deep learning models and optimize corresponding activity recognizers to study their sensitivity to different feature encodings. Furthermore, we include seven commonly used shallow classification models for comparison purposes. Finally, we conduct extensive experiments on three publicly available smart home datasets. Results indicate that the performance of both deep learning and shallow models is closely associated with the raw signal encodings and demonstrate the superiority of one-dimensional convolutional neural networks over its competitors in terms of generalization across scenarios. Besides, we preliminarily explore the influence of NULL class on an activity recognizer and experimentally show its negative impact on overall accuracy, enlightening relevant studies to consider it in developing a practical activity recognition system.
更多查看译文
关键词
Sensors, Hidden Markov models, Activity recognition, Intelligent sensors, Deep learning, Sensor phenomena and characterization, Data models, Feature encoding, feature learning, activity recognition, smart home
AI 理解论文
溯源树
样例
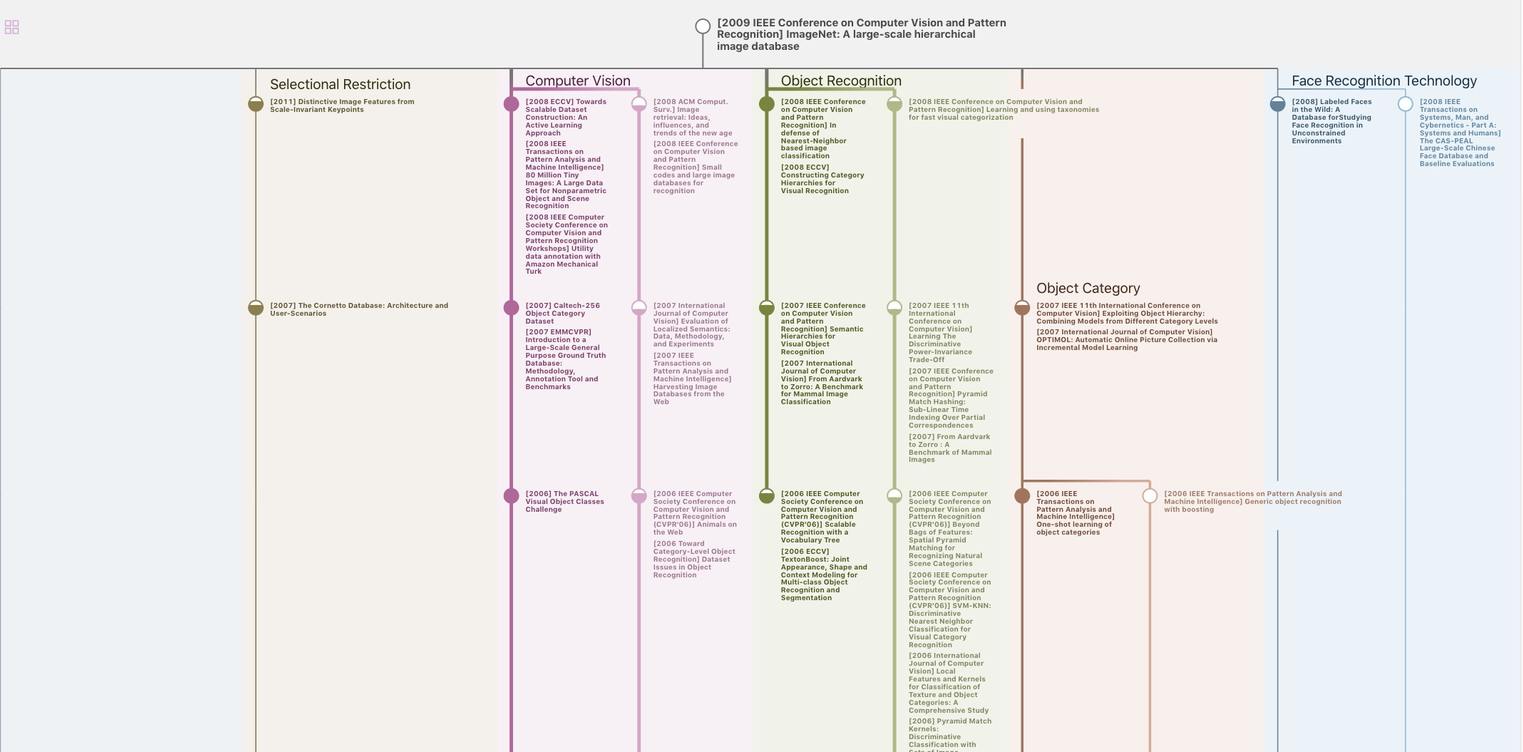
生成溯源树,研究论文发展脉络
Chat Paper
正在生成论文摘要