A Machine Learning-Based Approach For Auto-Detection And Localization Of Targets In Underwater Acoustic Array Networks
IEEE TRANSACTIONS ON VEHICULAR TECHNOLOGY(2020)
摘要
The localization and tracking of underwater objects have many applications. In a proactive underwater sensor array, some nodes will periodically broadcast linear frequency modulated (LFM) signals, which will hit the targets, get reflected and received by the other nodes. Depending on the target's position and velocity, the received signals will also be LFM signals of different frequencies and frequency rates. We can use the Fractional Fourier Transform (FrFT) to analyze the received signal's spectrum and find the peak. Based on the location of the peak, the target's distance and radial velocity can be estimated. However, the accuracy is highly dependent on the sampling interval of the spectrum. Smaller sampling interval leads to higher accuracy but also induces considerable complexity. To overcome this issue, we propose a machine learning-based approach to automatically detect the existence of the target, and roughly estimate the peak's location if targets exist. Then over-sampling can be conducted for a small area around the peak, leading to improved accuracy and reduced complexity. The idea is based on the following observation: if a target exists, we will be able to observe an "X" pattern on the spectrum. Extensive simulations are conducted to verify the effectiveness of the proposed architecture.
更多查看译文
关键词
Target tracking, Estimation, Probes, Sonar, Underwater acoustics, Fourier transforms, Complexity theory, Underwater, localization, machine learning, fractional fourier transform, sensor array
AI 理解论文
溯源树
样例
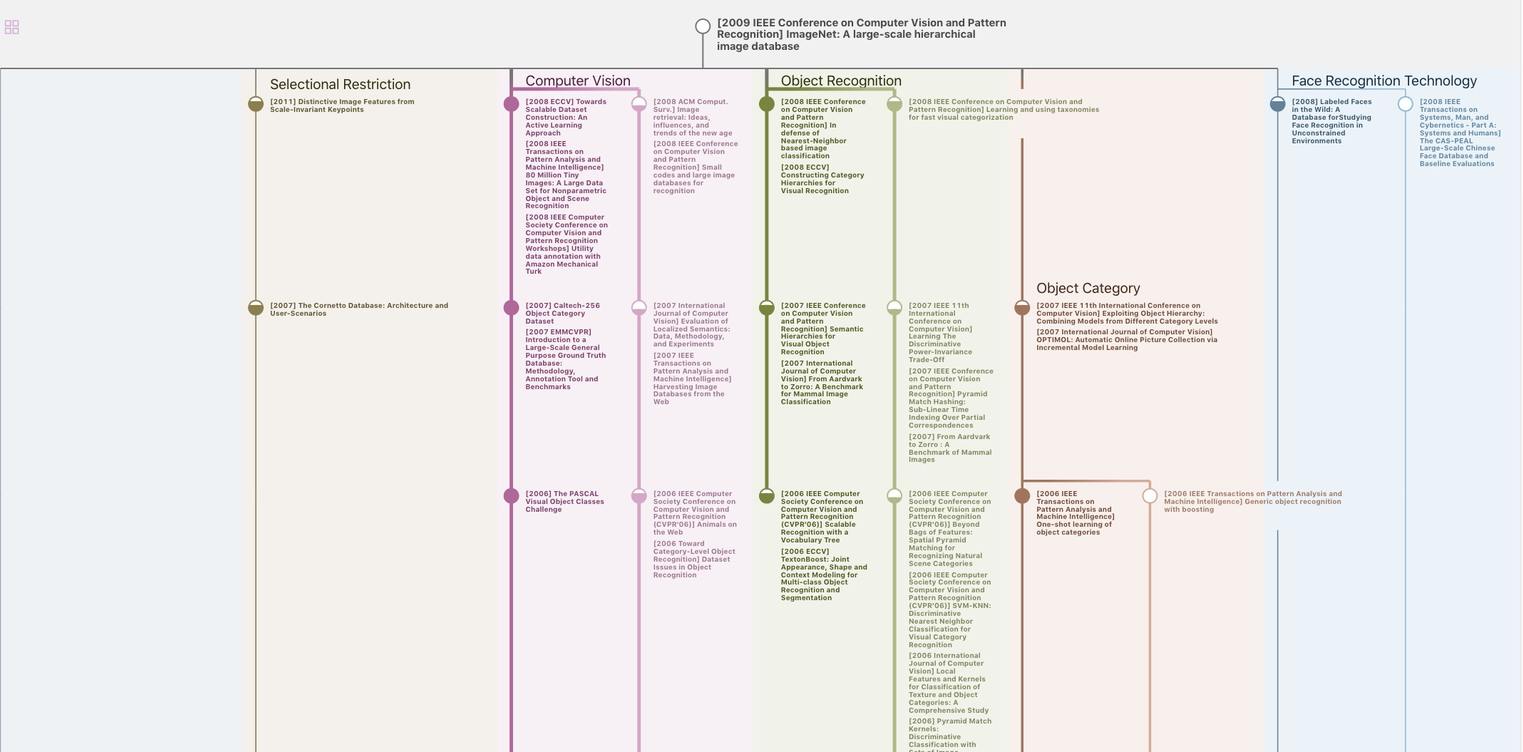
生成溯源树,研究论文发展脉络
Chat Paper
正在生成论文摘要