A comparison of classification methods across different data complexity scenarios and datasets
Expert Systems with Applications(2021)
摘要
Recent research assessed the performance of classification methods mainly on concrete datasets whose statistical characteristics are unknown or unreported. The performance furthermore is often determined by only one performance measure, such as the area under the receiver operating characteristic curve. The performance of several classification methods in four different complexity scenarios and on datasets described by five data characteristics is compared in this paper. Synthetical datasets are used to control their statistical characteristics and real datasets are used to verify our findings. The performance of each classification method is determined by six measures. The investigation reveals that heterogeneous classifiers perform best on average, bagged CART is especially recommendable for datasets with low dimensionality and high sample size, kernel-based classification methods perform very well especially with a polynomial kernel, but require a rather long time for training and a nearest shrunken neighbor classifier is recommendable in case of unbalanced datasets. These findings help researchers and practitioners finding an appropriate method for their binary classification problems.
更多查看译文
关键词
Binary classification,Classification methods,Performance comparison,Data characteristics
AI 理解论文
溯源树
样例
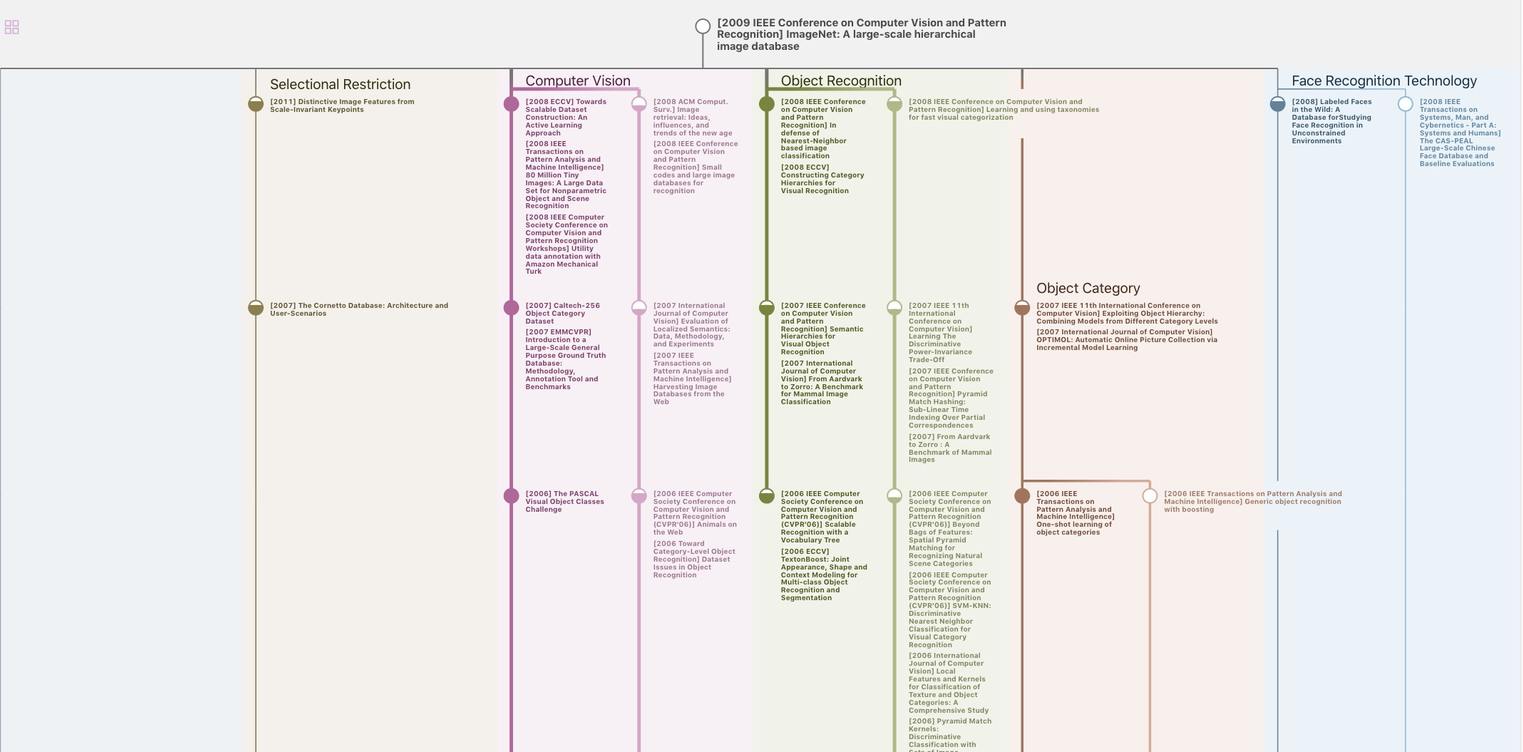
生成溯源树,研究论文发展脉络
Chat Paper
正在生成论文摘要