Convolution-GRU Based on Independent Component Analysis for fMRI Analysis with Small and Imbalanced Samples
APPLIED SCIENCES-BASEL(2020)
摘要
Functional magnetic resonance imaging (fMRI) is a commonly used method of brain research. However, due to the complexity and particularity of the fMRI task, it is difficult to find enough subjects, resulting in a small and, often, imbalanced dataset. A dataset with small samples causes overfitting of the learning model, and the imbalance will make the model insensitive to the minority class, which has been a problem in classification. It is of great significance to classify fMRI data with small and imbalanced samples. In the present study, we propose a 3-step method on a small and imbalanced fMRI dataset from a word-scene memory task. The steps of the method are as follows: (1) An independent component analysis is performed to reduce the dimension of data; (2) The synthetic minority oversampling technique is used to generate new samples of the minority class to balance data; (3) A convolution-Gated Recurrent Unit (GRU) network is used to classify the independent component signals, indicating whether the subjects are performing episodic memory tasks. The accuracy of the proposed method is 72.2%, which improves the classification performance compared with traditional classifiers such as support vector machines (SVM), logistic regression (LGR), linear discriminant analysis (LDA) and k-nearest neighbor (KNN), and this study gives a biomarker for evaluating the reactivation of episodic memory.
更多查看译文
关键词
functional magnetic resonance imaging,independent component analysis,deep learning,recurrent neural network,functional connectivity,episodic memory,small sample learning
AI 理解论文
溯源树
样例
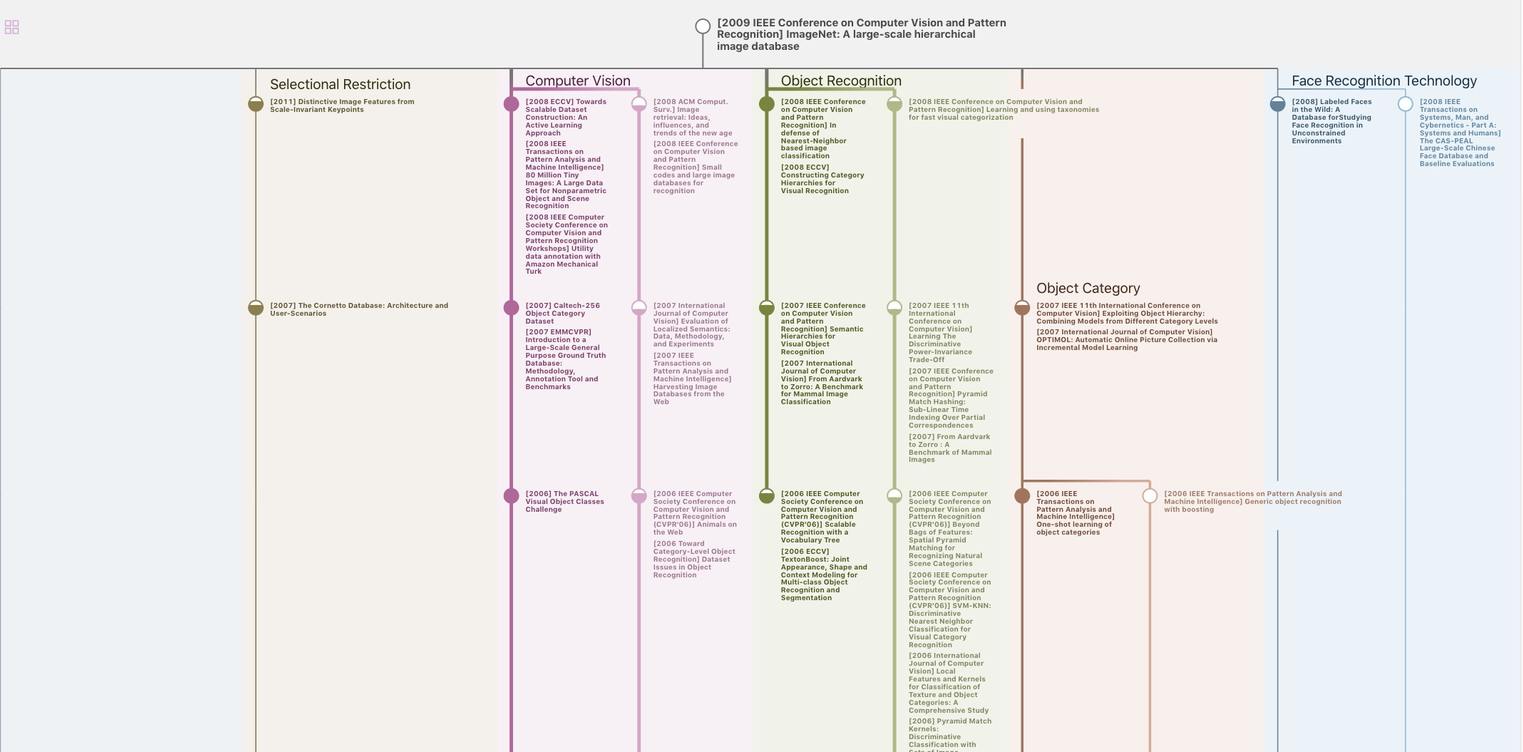
生成溯源树,研究论文发展脉络
Chat Paper
正在生成论文摘要