Highly-Efficient and Automatic Spectrum Inspection Based on AutoEncoder and Semi-Supervised Learning for Anomaly Detection in EONs
Journal of Lightwave Technology(2021)
摘要
To closely monitor the performance of lightpaths in an elastic optical network (EON), people need to rely on real-time and fine-grained spectrum monitoring. This, however, will generate tremendous telemetry data, which can put great pressure on both the control and data planes. In this work, we design and experimentally demonstrate AutoSpecheck, which is a DL-assisted network automation (DLaNA) system that can realize highly-efficient and automatic spectrum inspection for anomaly detection in EONs. Specifically, we architect AutoSpecheck based on the software-defined EON (SD-EON) architecture, and propose techniques to greatly reduce the loads of data reporting (in the data plane) and data analyzing (in the control plane). To reduce the loads of data reporting, we leverage the AutoEncoder (AE) technique to design a spectrum data compression method. To improve the efficiency of data analytics, we first design a coarse filtering module (CFM) to let the control plane filter out most of the normal data before invoking the DL-based anomaly detection. Then, to address the difficulty of labeling massive spectrum data, we develop a DL-based anomaly detection based on semi-supervised learning. Our experimental demonstrations consider two representative intra-channel anomalies (i.e., the filter drifting and in-band jamming), and the results confirm that AutoSpecheck can achieve highly-efficient and automatic spectrum inspection for anomaly detection in EONs.
更多查看译文
关键词
Software-defined networking (SDN),elastic optical networks (EONs),network automation,anomaly detection,deep learning (DL),autoEncoder (AE),spectrum inspection
AI 理解论文
溯源树
样例
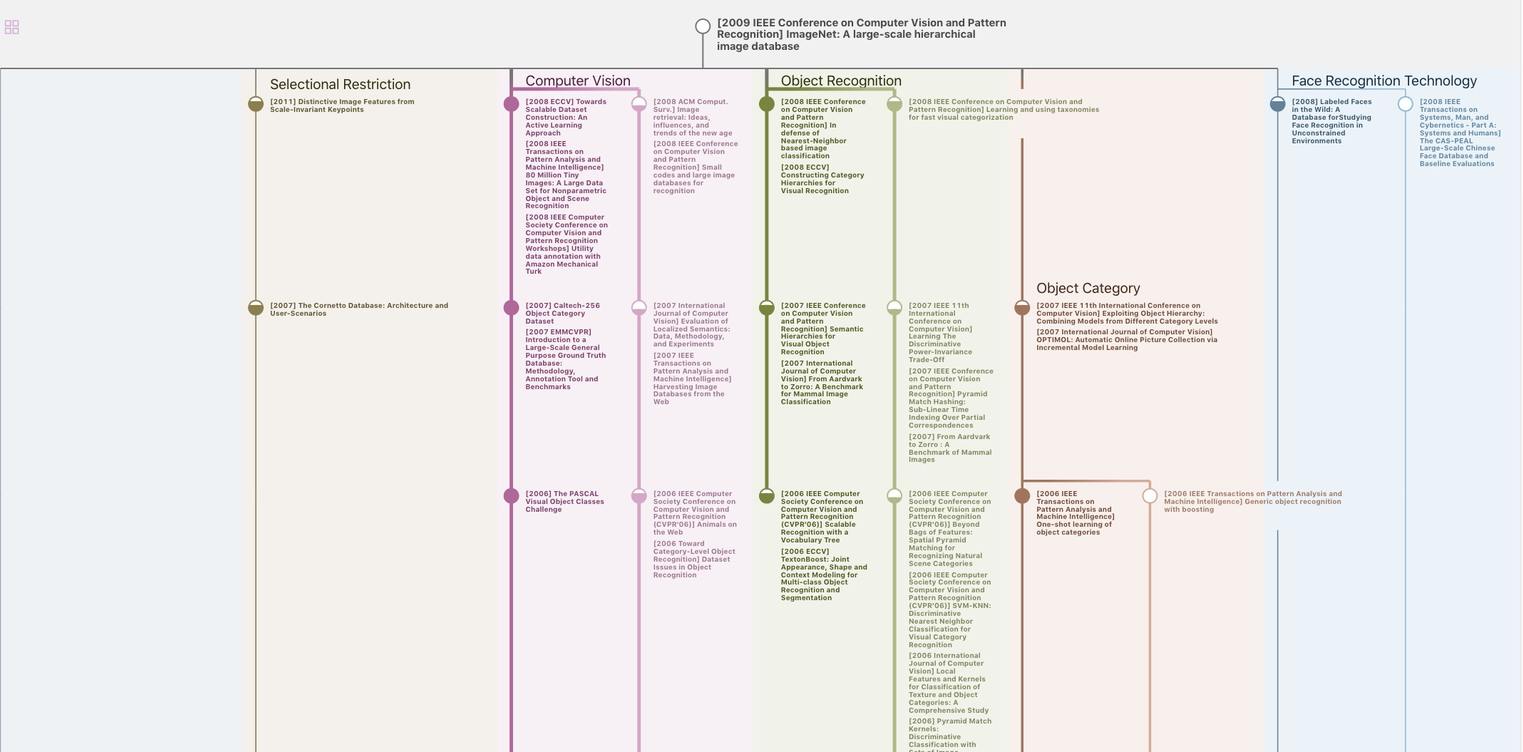
生成溯源树,研究论文发展脉络
Chat Paper
正在生成论文摘要