Fast Adapting Without Forgetting for Face Recognition
IEEE Transactions on Circuits and Systems for Video Technology(2021)
摘要
Although face recognition has made dramatic improvements in recent years, there are still many challenges in real-world applications such as face recognition for the elderly and children, for the surveillance scenes and for Near infrared vs. Visible light (NIR-VIS) heterogeneous scene, etc. Due to the existence of these challenges, there are usually domain gaps between training (source domain) and test (target domain). A common way to improve the performance on the target domain is fine-tuning the base model trained on source domain using target data. However, it will severely degrade performance on the source domain. Another way which jointly trains models using both source and target data, suffers from the heavy computations and large data storage, especially when we continue to encounter new domains. In response to these problems, we introduce a new challenging task: Single Exemplar Domain Incremental Learning (SE-DIL), which utilizes the target domain data and just one exemplar per identity from source domain data to quickly improve the performance on the target domain while keeping the performance on the source domain. To deal with SE-DIL, we propose our Fast Adapting without Forgetting (FAwF) method with three components: margin-based exemplar selection, prototype-based class extension and hard&soft knowledge distillation. Through FAwF, we can well maintain the source domain performance with only one sample per source domain class, greatly reducing the fine-tuning time-cost and data storage. Besides, we collected a large-scale children face dataset KidsFace with 12 K identities for studying the SE-DIL in face recognition. Extensive analysis and experiments on our KidsFace-Test protocol and other challenging face test sets show that our method performs better than the state-of-the-art methods on both target and source domain.
更多查看译文
关键词
Single exemplar,domain incremental learning,fast adapting without forgetting,face recognition
AI 理解论文
溯源树
样例
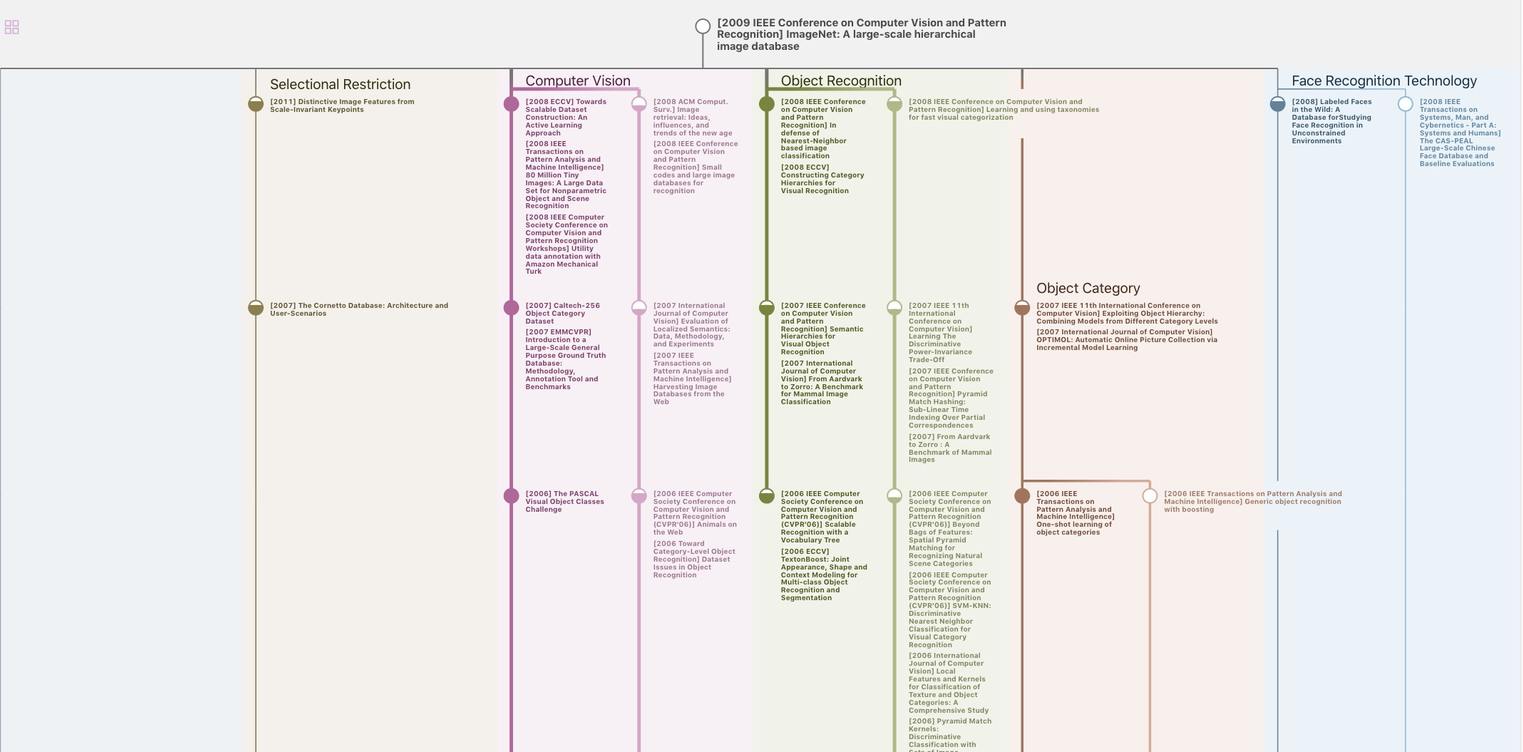
生成溯源树,研究论文发展脉络
Chat Paper
正在生成论文摘要