Wavelet Based Deep Recursive Pyramid Convolution Residual Network For Single Image Rain Removal
IEEE ACCESS(2020)
摘要
Image rain removal aims to separate the background image from the rainy image. During the past three years, the image rain removal with deep convolutional neural networks has achieved impressive performance. However, how to reach tradeoff between high de-raining performance and low model parameters is still a challenge. To address the issue, the paper is devoted to exploring a novel method based on wavelet deep recursive pyramid convolution residual network (WDRPRN), in which discrete wavelet transform is embedded to decompose the rainy image in different frequency domains, and the deep recursive pyramid convolution residual network (DRPRN) can well predict the residual coefficients between rainy image and clean image. In addition, compared with other neural networks, the DRPRN adopts recursive model that can cost fewer parameters. Plentiful of experiments on synthetic and real-world datasets show that the proposed method is significantly superior to the recent state-of-the-art algorithms.
更多查看译文
关键词
Rain, Low-pass filters, Discrete wavelet transforms, Feature extraction, Convolution, Image rain removal, wavelet transform, residual coefficients, low model parameters
AI 理解论文
溯源树
样例
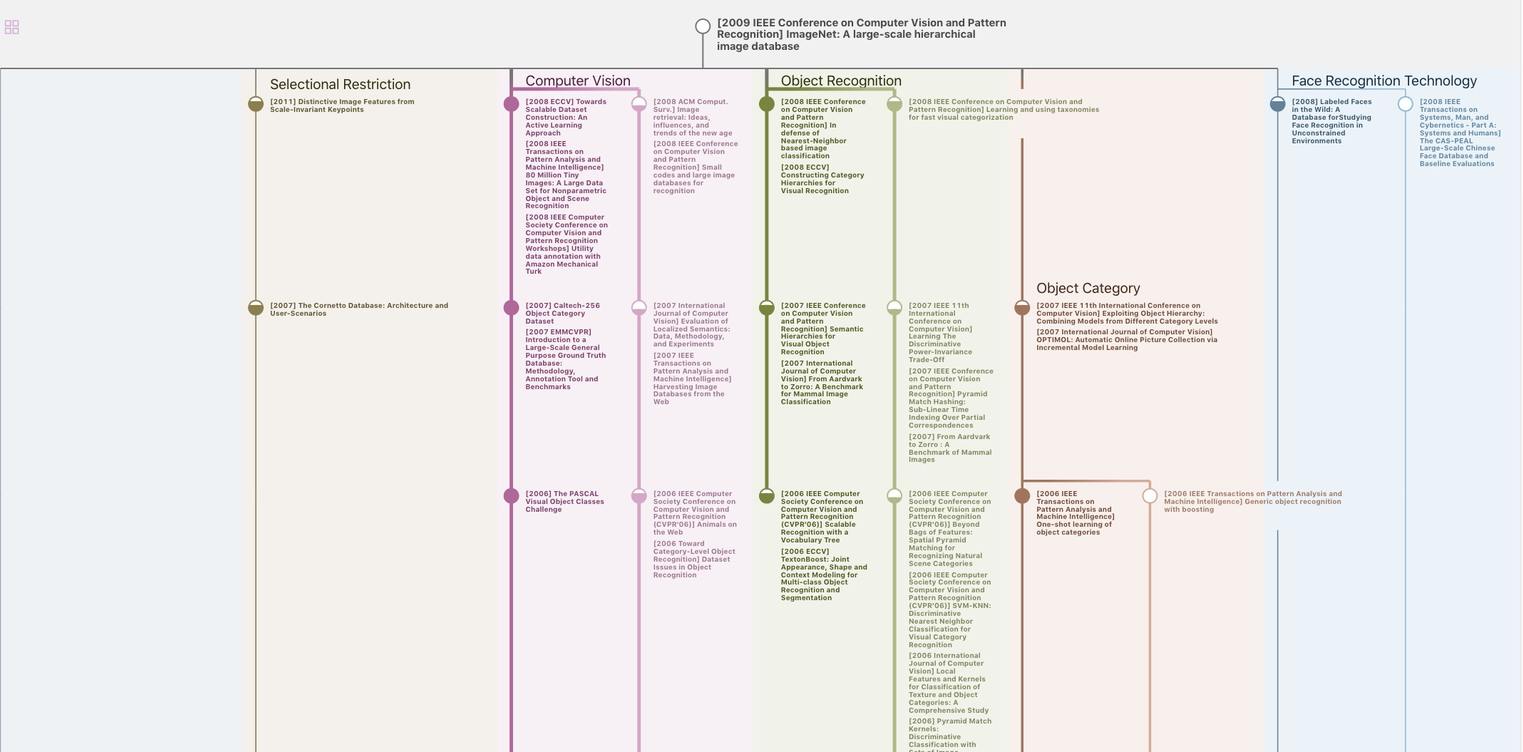
生成溯源树,研究论文发展脉络
Chat Paper
正在生成论文摘要