High Order Semantic Relations-Based Temporal Recommendation Model by Collaborative Knowledge Graph Learning.
Interational Conference on Web-Age Information Management(2020)
摘要
Knowledge graph (KG) as the source of side information has been proven to be useful to alleviate the data sparsity and cold start. Existing methods usually exploit the semantic relations between entities by learning structural or semantic paths information. However, they ignore the difficulty of information fusion and network alignment when constructing knowledge graph from different domains, and do not take temporal context into account. To address the limitations of existing methods, we propose a novel High-order semantic Relations-based Temporal Recommendation (HRTR), which captures the joint effects of high-order semantic relations in Collaborative Knowledge Graph (CKG) and temporal context. Firstly, it automatically extracts different order connectivities to represent semantic relations between entities from CKG. Then, we define a joint learning model to capture high-quality representations of users, items, and their attributes by employing TransE and recurrent neural network, which captures not only structural information, but also sequence information by encoding semantic paths, and to take their representations as the users’/items’ long-term static features. Next, we respectively employ LSTM and attention machine to capture the users’ and items’ short-term dynamic preferences. At last, the long-short term features are seamlessly fused into recommender system. We conduct extensive experiments on real-world datasets and the evaluation results show that HRTR achieves significant superiority over several state-of-the-art baselines.
更多查看译文
关键词
Collaborative knowledge graph, High-order semantic relation, Structural information, Temporal recommendation
AI 理解论文
溯源树
样例
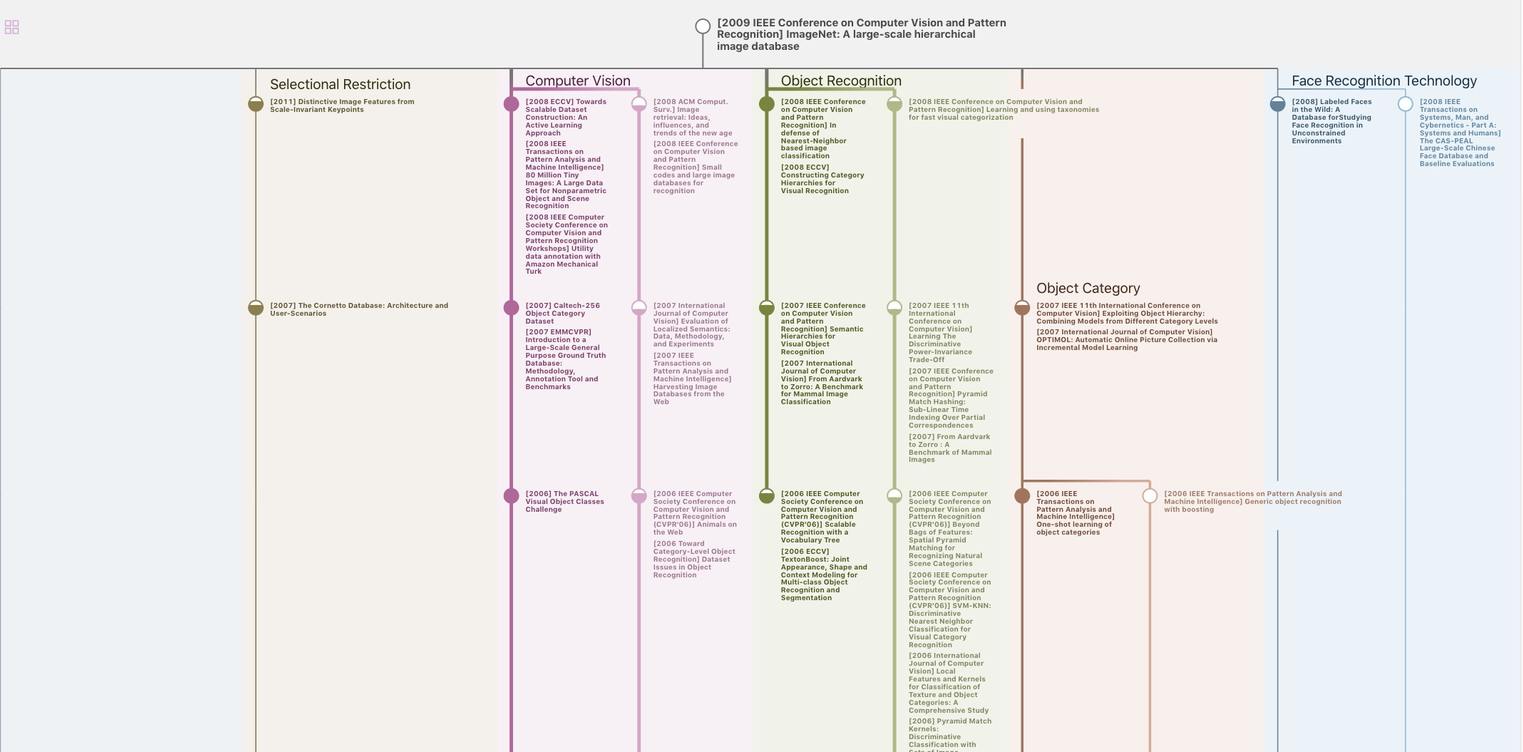
生成溯源树,研究论文发展脉络
Chat Paper
正在生成论文摘要