An Empirical Study of Downstream Analysis Effects of Model Pre-Processing Choices
Open Journal of Statistics(2020)
摘要
This study uses an empirical analysis to quantify the downstream analysis effects of data pre-processing\r\nchoices. Bootstrap data simulation is used to measure the bias-variance\r\ndecomposition of an empirical risk function, mean square error (MSE). Results\r\nof the risk function decomposition are used to measure the effects of model\r\ndevelopment choices on model bias,\r\nvariance, and irreducible error. Measurements of bias and variance are then\r\napplied as diagnostic procedures for model pre-processing and development. Best\r\nperforming model-normalization-data structure combinations were found to\r\nillustrate the downstream analysis effects of these model development choices. In additions,\r\nresults found from simulations were verified and expanded to include additional\r\ndata characteristics (imbalanced, sparse) by testing on benchmark datasets\r\navailable from the UCI Machine Learning Library. Normalization results on\r\nbenchmark data were consistent with those found using simulations, while also\r\nillustrating that more complex and/or non-linear models provide better\r\nperformance on datasets with additional complexities. Finally, applying the\r\nfindings from simulation experiments to previously tested applications led to\r\nequivalent or improved results with less model development overhead and processing\r\ntime.
更多查看译文
AI 理解论文
溯源树
样例
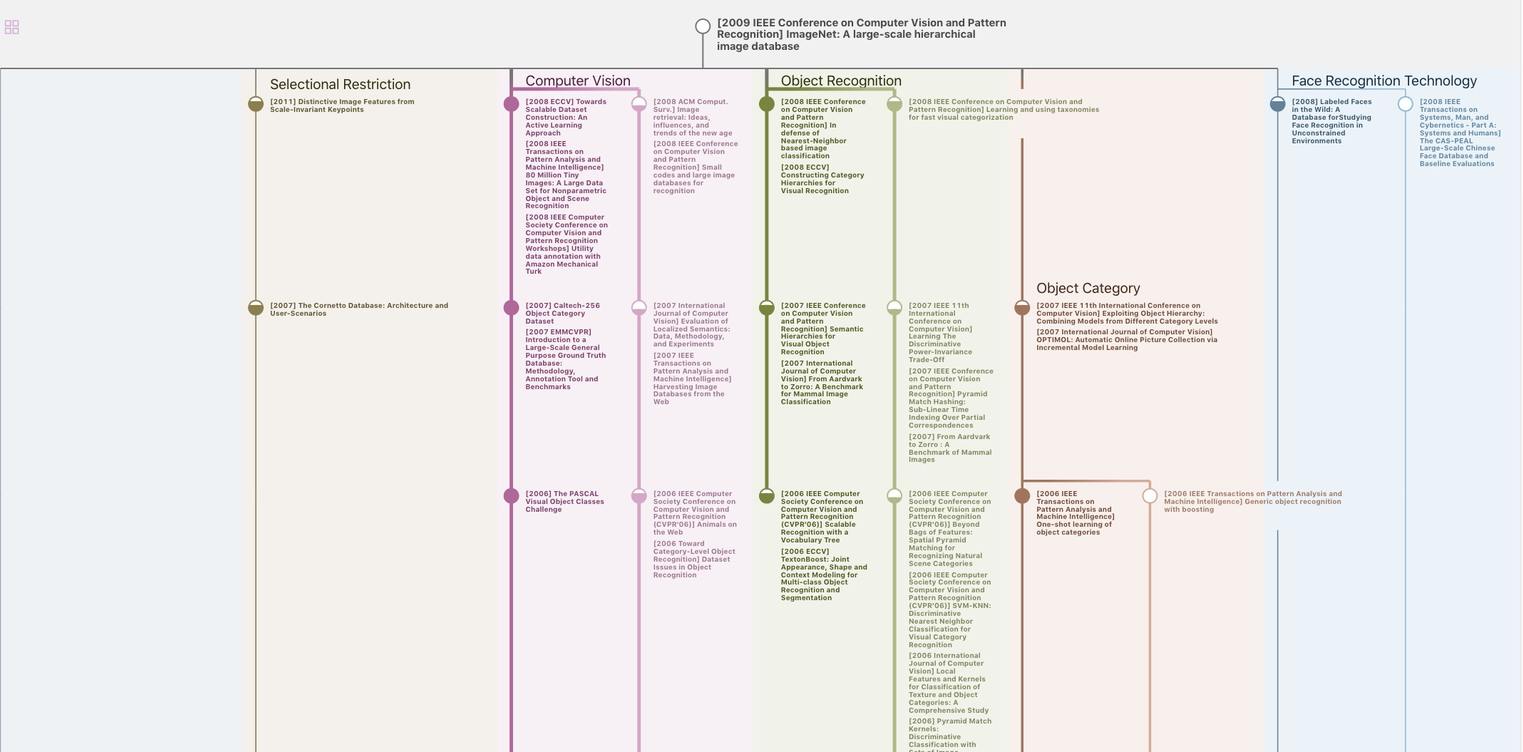
生成溯源树,研究论文发展脉络
Chat Paper
正在生成论文摘要