Bcrl: Long Text Friendly Knowledge Graph Representation Learning
SEMANTIC WEB - ISWC 2020, PT I(2020)
摘要
The sparse data and large computational overhead in the use of large-scale knowledge graphs have caused widespread attention to Knowledge Representation Learning (KRL) technology. Although many KRL models have been proposed to embed structure information, their ability to accurately represent newly added entities or entities with few relations is significantly insufficient. In some studies, the introduction of textual information has partially solved this problem. However, most existing text-enhanced models only consider the shallow description information of the entities, and ignore the relation mention information between entities, and deep semantic information between sentences and words, which is not optimized for long texts supplementary information like Wikipedia.In this paper, we proposed a long text friendly structure-text joint KRL model, named BCRL (BERT and CNN Representation Learning), which can effectively explore rich semantics embedded in entity description and relation mention text taking Wikipedia as supplementary information. For the obtained text of entity description and relation mention, the model first uses the BERT model to generate sentence vector representation respectively. Then it uses a convolutional neural network with an attention mechanism to select valid information in the text and obtain the overall vector representation of the text. Finally, the gate mechanism is used to combine the structure-based and the text-based vectors to generate the final joint representation. We evaluated the performance of our BCRL model on link prediction tasks using FB15K and WN18 datasets. The experimental results show that BCRL outperforms structure-only models and text-enhanced models in most cases, and has significant advantages in complex relation representation.
更多查看译文
关键词
Knowledge Representation Learning, Long text, BERT, Convolutional neural network
AI 理解论文
溯源树
样例
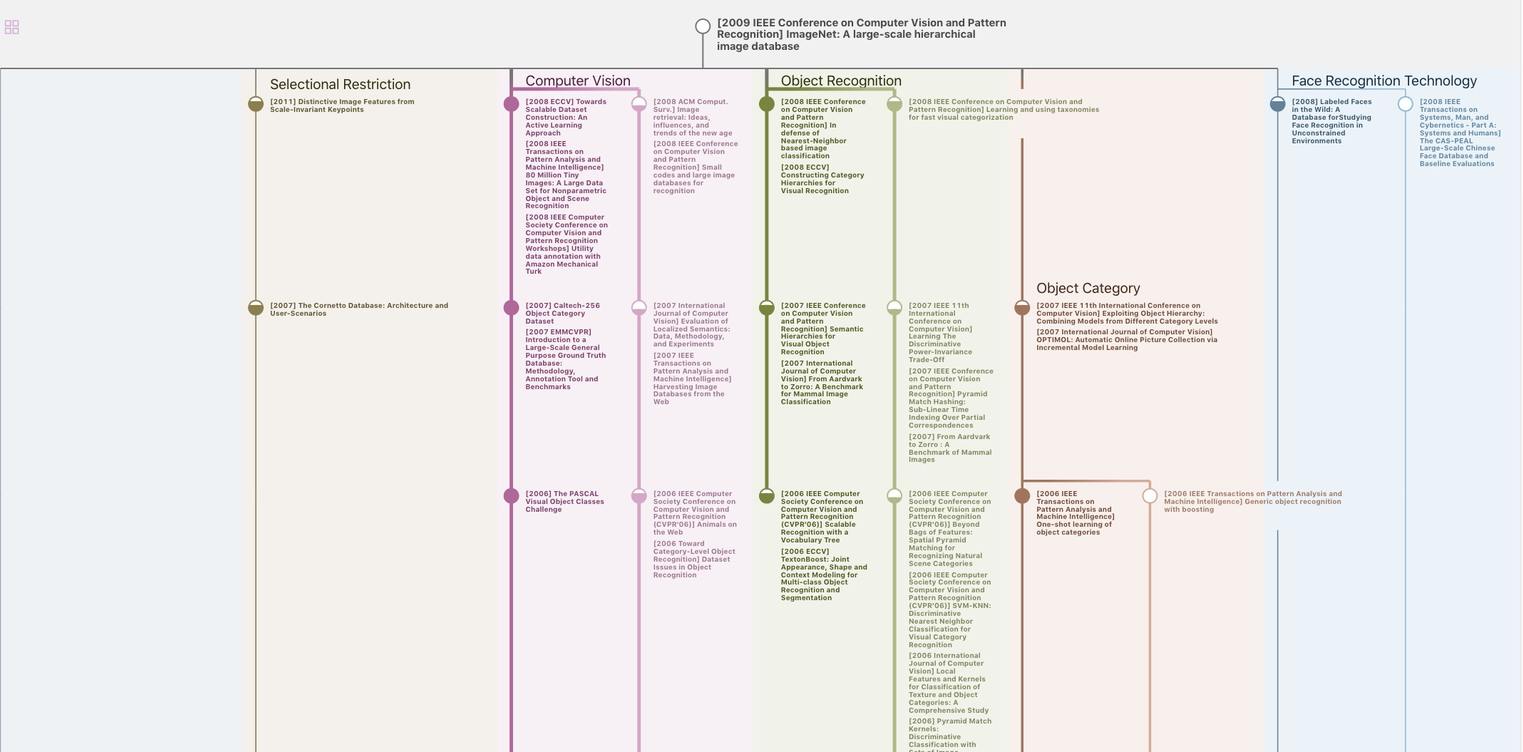
生成溯源树,研究论文发展脉络
Chat Paper
正在生成论文摘要