Data-driven Cyberattack Detection for Photovoltaic (PV) Systems through Analyzing Micro-PMU Data
2020 IEEE Energy Conversion Congress and Exposition (ECCE)(2020)
摘要
With increasing exposure to software-based sensing and control, Photovoltaic (PV) systems are facing higher risks of cyber attacks. To ensure the system stability and minimize potential economic losses, it is imperative to monitor operating states and detect attacks at the early stage. To meet this demand, Micro-Phasor Measurement Units (μPMU) are increasingly popular in monitoring distribution networks. However, due to the relatively low sampling rate, μPMU has not yet been used to detect and classify cyber-attacks in power electronics enabled smart grid. To our knowledge, this is one of the first attempts to use μPMU to detect cyber attacks that degrade the performance of power electronics systems. We propose to apply data-driven methods on micro-PMU data to implement attack detection. We have evaluated data-driven methods, including decision tree (DT), K-nearest neighbor (KNN), support vector machine (SVM), artificial neural network (ANN), long short-term memory (LSTM) and convolutional neural network (CNN). The proposed CNN model achieves the required performances with the highest 99.23% accuracy and 0.9963 F
1
score.
更多查看译文
关键词
PV systems,Cyber physical security,Data-driven models
AI 理解论文
溯源树
样例
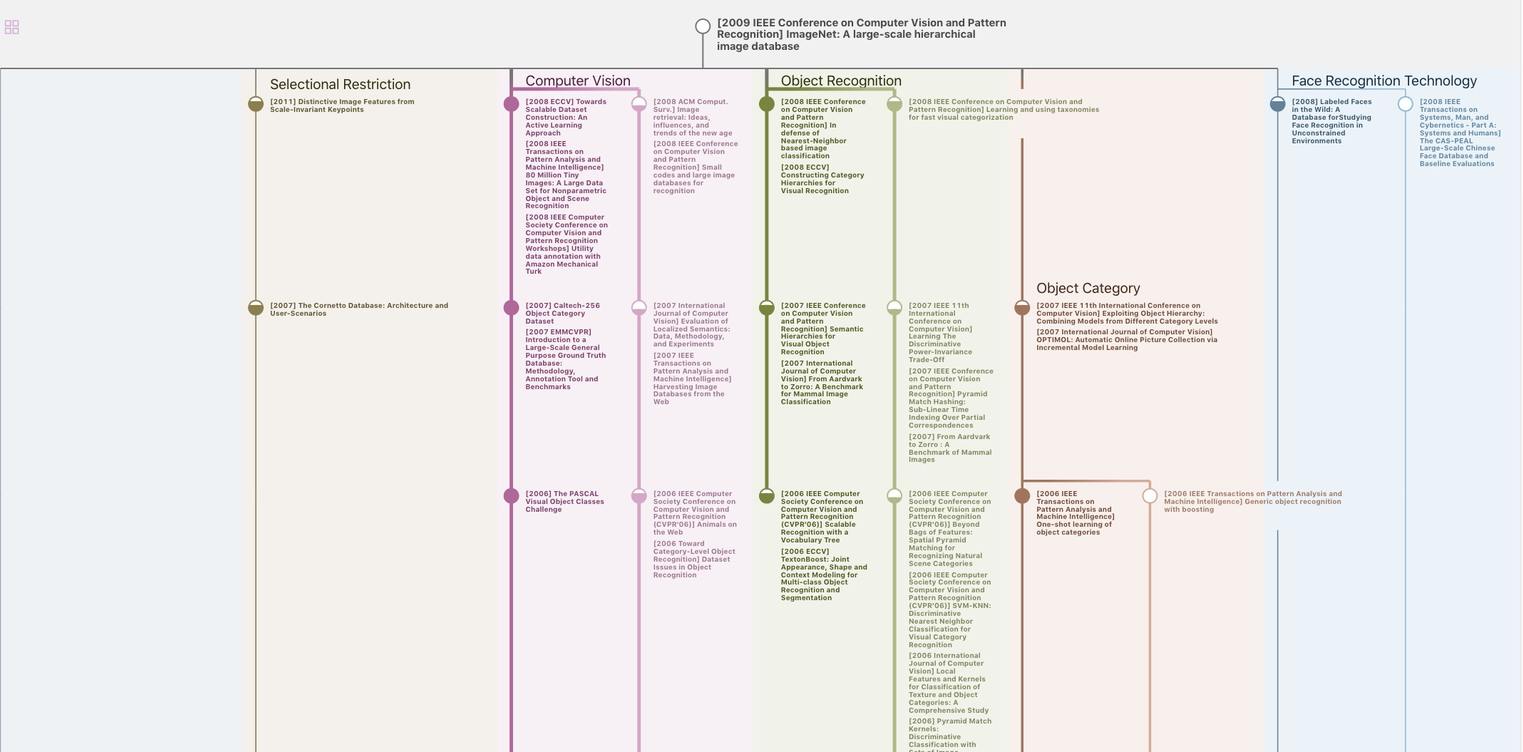
生成溯源树,研究论文发展脉络
Chat Paper
正在生成论文摘要