Multiclass Classification on High Dimension and Low Sample Size Data Using Genetic Programming
IEEE Transactions on Emerging Topics in Computing(2022)
摘要
Multiclass classification is one of the most fundamental tasks in data mining. However, traditional data mining methods rely on the model assumption, they generally can suffer from the overfitting problem on high dimension and low sample size (HDLSS) data. Trying to address multiclass classification problems on HDLSS data from another perspective, we utilize Genetic Programming (GP), an intrinsic evolutionary classification algorithm that can implement feature construction automatically without model assumption. This article develops an ensemble-based genetic programming classification framework, the Sigmoid-based Ensemble Gene Expression Programming (SE-GEP). To relieve the problem of output conflict in GP-based multiclass classifiers, the proposed method employs a flexible probability representation with continuous relaxation to better integrate the output of all the binary classifiers, an effective data division strategy to further enhance the ensemble performance, and a novel sampling strategy to refine the existing GP-based binary classifier. The experiment results indicate that SE-GEP can attain better classification accuracy compared to other GP methods. Moreover, the comparison with other representative machine learning methods indicates that SE-GEP is a competitive method for multiclass classification in HDLSS data.
更多查看译文
关键词
Genetic programming,gene expression programming,high dimension,classification,low sample size,ensemble learning
AI 理解论文
溯源树
样例
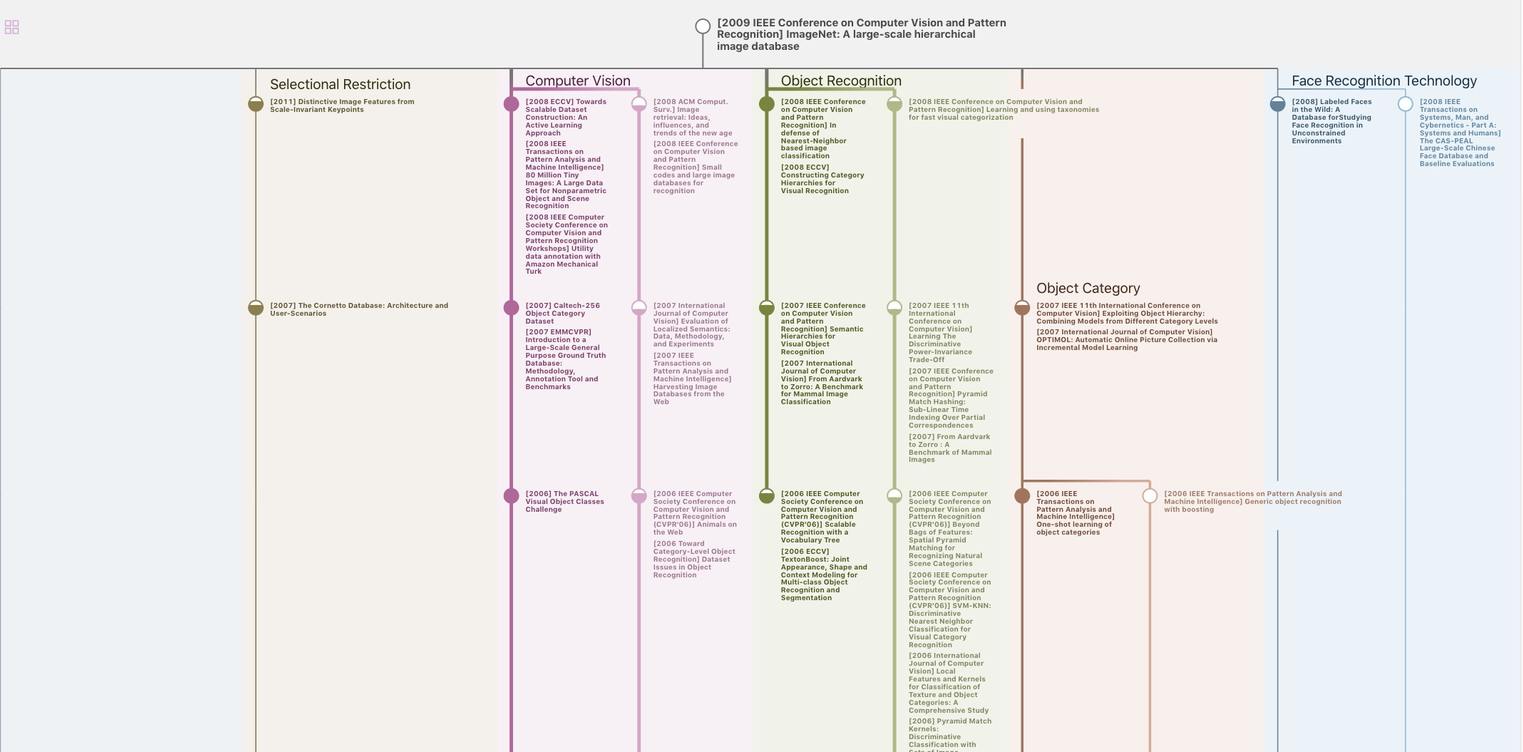
生成溯源树,研究论文发展脉络
Chat Paper
正在生成论文摘要