Knowledge Graph Representation Learning with Multi-Scale Capsule-Based Embedding Model Incorporating Entity Descriptions
IEEE access(2020)
摘要
A Knowledge Graph (KG) is a directed graph with nodes as entities and edges as relations. KG representation learning (KGRL) aims to embed entities and relations in a KG into continuous low-dimensional vector spaces, so as to simplify the manipulation while preserving the inherent structure of the KG. In this paper, we propose a KG embedding framework, namely MCapsEED (Multi-Scale Capsule-based Embedding Model Incorporating Entity Descriptions). MCapsEED employs a Transformer in combination with a relation attention mechanism to identify the relation-specific part of an entity description and obtain the description representation of an entity. The structured and description representations of an entity are integrated into a synthetic representation. A 3-column matrix with each column a synthetic representation of an element of a triple is fed into a Multi-Scale Capsule-based Embedding model to produce final representations of the head entity, the tail entity and the relation. Experiments show that MCapsEED achieves better performance than state-of-the-art embedding models for the task of link prediction on four benchmark datasets. Our code can be found at https://github.com/1780041410/McapsEED.
更多查看译文
关键词
Knowledge graph representation learning,capsule network,link prediction,knowledge graph embedding
AI 理解论文
溯源树
样例
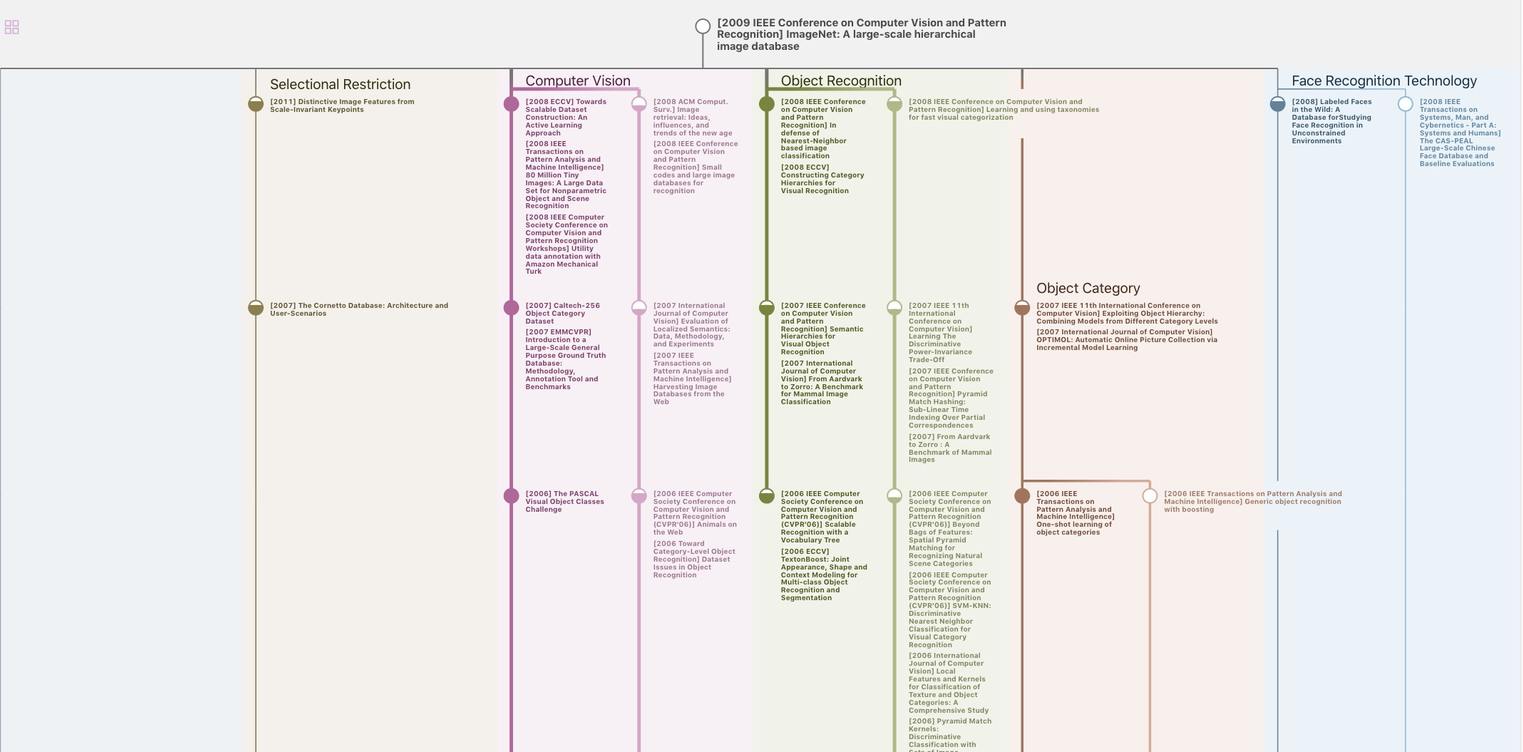
生成溯源树,研究论文发展脉络
Chat Paper
正在生成论文摘要