Boosting One-Shot Spectral Super-Resolution Using Transfer Learning
IEEE transactions on computational imaging(2020)
摘要
Though deep learning based spectral super-resolution (SSR) methods have state-of-the-art performances, most previous deep spectral super-resolution approaches require extensive paired RGB images and hyperspectral images (HSIs) for well-fitting learning. However, in real cases, the cost of generating such paired images is too prohibitive to collect sufficient training samples. To solve this problem, we investigated one-shot SSR in a target domain. To avoid over-fitting, we introduced knowledge from a source domain to guide the one-shot SSR in the target domain and use the idea of spectral unmixing to remove the interference of different spectral characteristics, with which we proposed a spectral-unmixing inspired deep SSR framework. Experimental results on three benchmark SSR datasets showed the effectiveness of the proposed method.
更多查看译文
关键词
Hyperspectral imagery,spectral super-resolution,deep neural network,transfer learning,one-shot learning
AI 理解论文
溯源树
样例
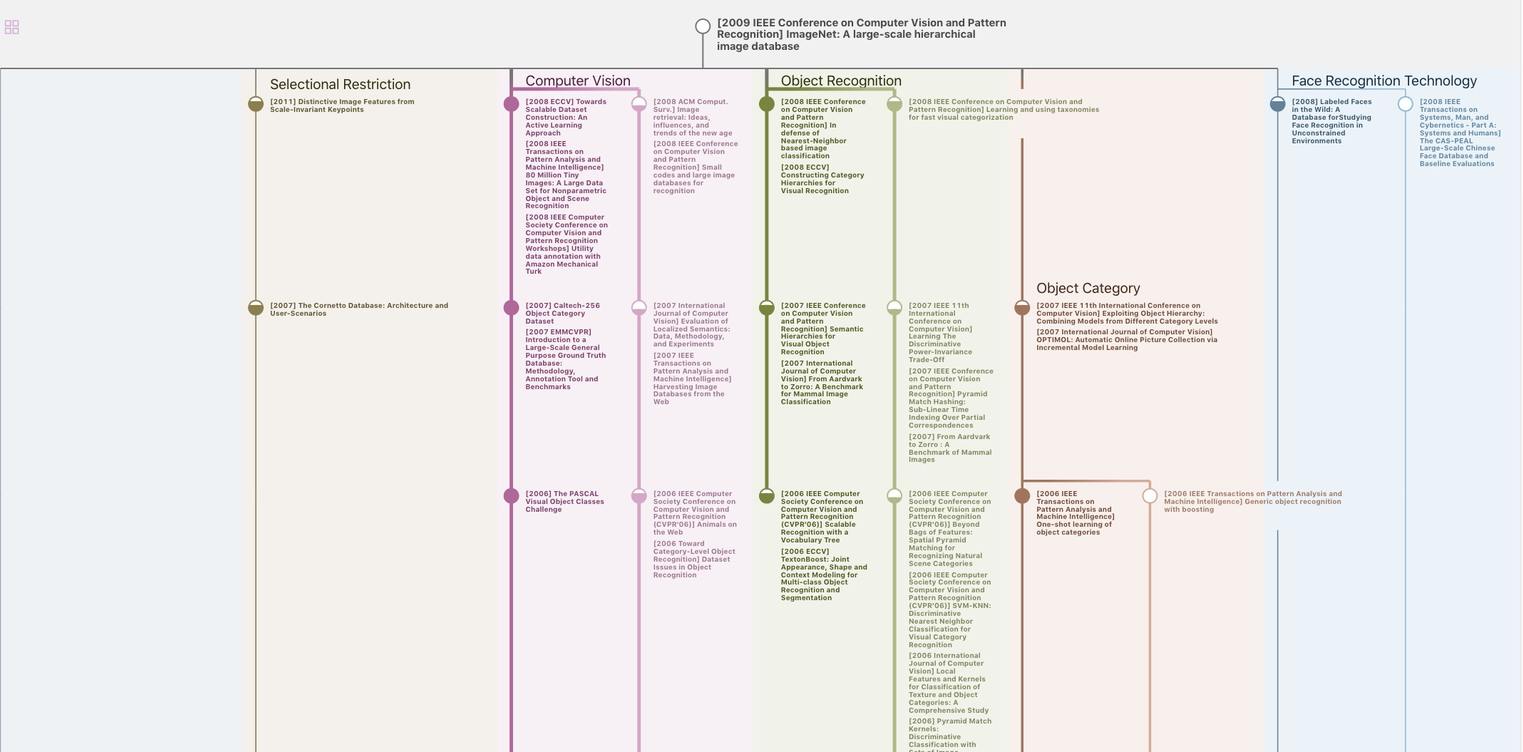
生成溯源树,研究论文发展脉络
Chat Paper
正在生成论文摘要