Mask-Guided Noise Restriction Adversarial Attacks For Image Classification
COMPUTERS & SECURITY(2021)
摘要
Deep neural networks (DNNs) are vulnerable to adversarial examples, which are generated by adding small noises to the benign examples, but make a deep model output inaccurate predictions. The noises are often imperceptible to humans, but are more likely to be perceived for the images with plain backgrounds or increased noise size. To address this issue, we propose a mask-guided adversarial attack method to remove the noises of semantically irrelevant regions in the backgrounds and make the adversarial noises more imperceptible. In addition, we enhance the transferability of the adversarial examples by rotation input strategy. We first convert the image saliency maps produced by the salient object detection technique to binary masks, then we combine the proposed rotation input strategy with iterative attack method to generate stronger adversarial images, and use the binary masks to restrict the noises to the salient objects/regions at each iteration. Experimental results show that the noises of the resultant adversarial examples are far less visible than the vanilla global noise adversarial examples, and our best attack reaches an average success rate of 85.9% under the black-box attack setting, demonstrating the effectiveness of the proposed method. (C) 2020 Elsevier Ltd. All rights reserved.
更多查看译文
关键词
Deep neural network, Noise restriction, Adversarial example, Transferability, Adversarial attack
AI 理解论文
溯源树
样例
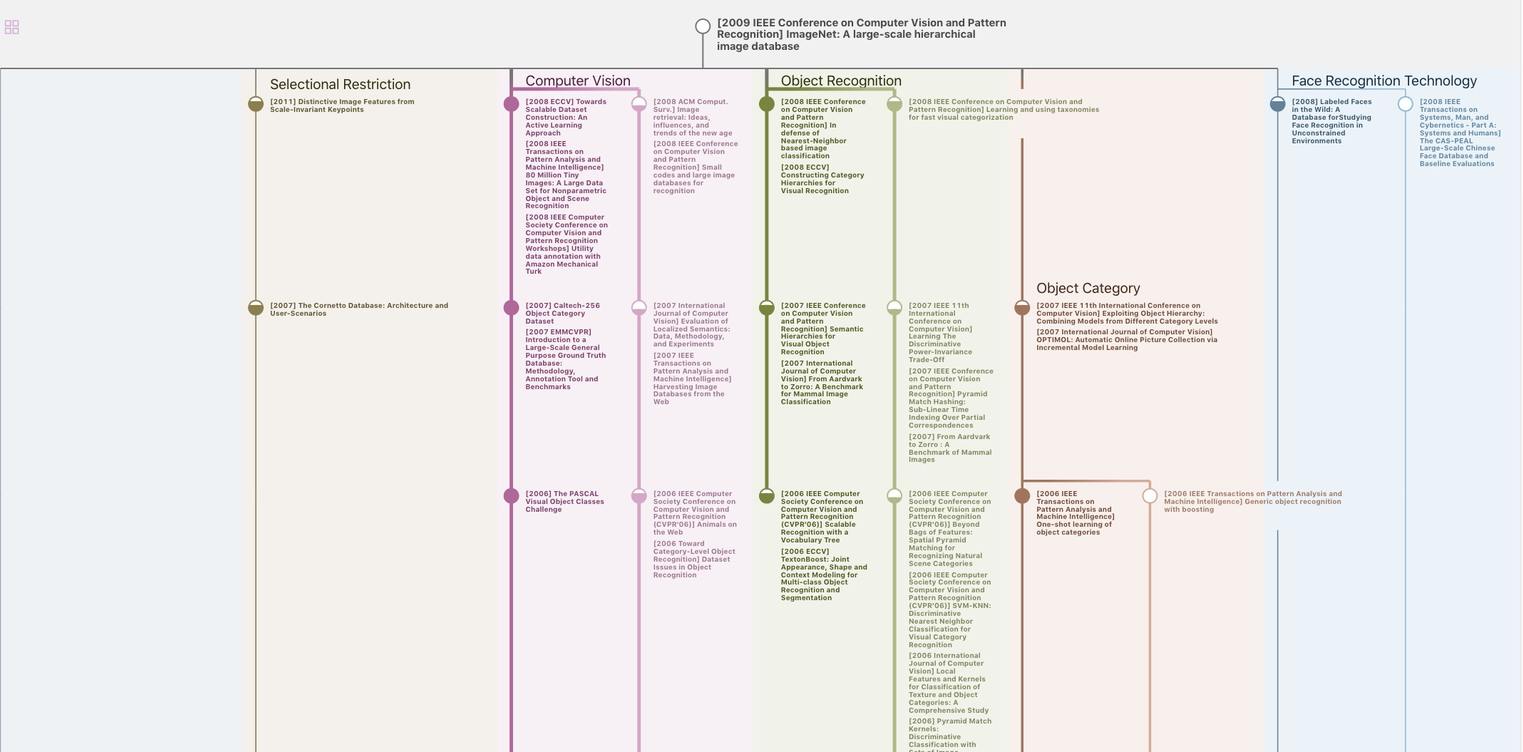
生成溯源树,研究论文发展脉络
Chat Paper
正在生成论文摘要